Knowledge Distillation on Cross-Modal Adversarial Reprogramming for Data-Limited Atribute Inference
COMPANION OF THE WORLD WIDE WEB CONFERENCE, WWW 2023(2023)
摘要
Social media generates a rich source of text data with intrinsic user attributes (e.g., age, gender), where different parties benefit from disclosing them. Attribute inference can be cast as a text classification problem, which, however, suffers from labeled data scarcity. To address this challenge, we propose a data-limited learning model to distill knowledge on adversarial reprogramming of a visual transformer (ViT) for attribute inferences. Not only does this novel cross-modal model transfers the powerful learning capability from ViT, but also leverages unlabeled texts to reduce the demand on labeled data. Experiments on social media datasets demonstrate the state-of-the-art performance of our model on data-limited attribute inferences.
更多查看译文
关键词
Attribute Inference,Adversarial Reprogramming,Data-limited Learning,Knowledge Distillation
AI 理解论文
溯源树
样例
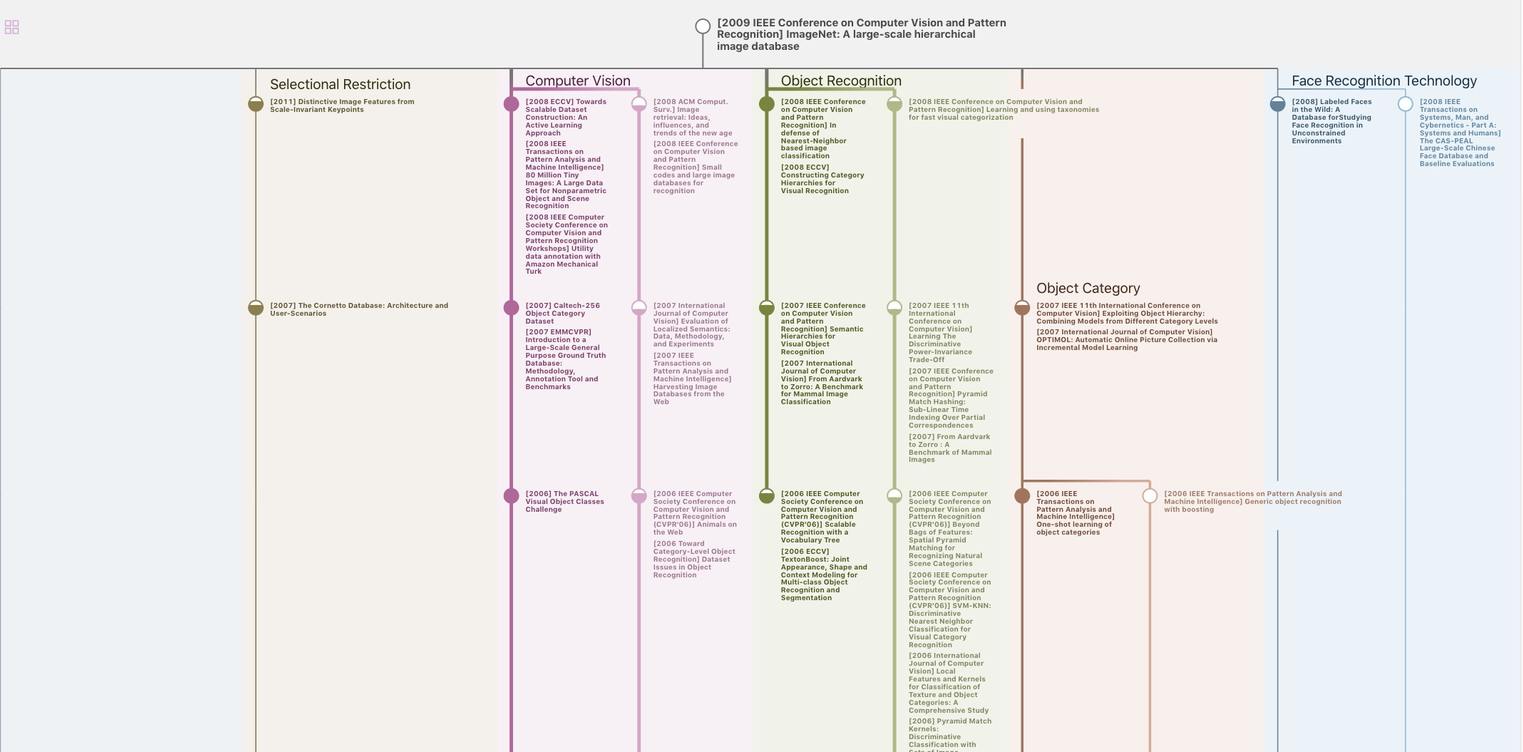
生成溯源树,研究论文发展脉络
Chat Paper
正在生成论文摘要