Optimizing Few-Shot Remote Sensing Scene Classification Based on an Improved Data Augmentation Approach
REMOTE SENSING(2024)
摘要
In the realm of few-shot classification learning, the judicious application of data augmentation methods has a significantly positive impact on classification performance. In the context of few-shot classification tasks for remote sensing images, the augmentation of features and the efficient utilization of limited features are of paramount importance. To address the performance degradation caused by challenges such as high interclass overlap and large intraclass variance in remote sensing image features, we present a data augmentation-based classification optimization method for few-shot remote sensing image scene classification. First, we construct a distortion magnitude space using different types of features, and we perform distortion adjustments on the support set samples while introducing an optimal search for the distortion magnitude (ODS) method. Then, the augmented support set offers a wide array of feature distortions in terms of types and degrees, significantly enhancing the generalization of intrasample features. Subsequently, we devise a dual-path classification (DC) decision strategy, effectively leveraging the discriminative information provided by the postdistortion features to further reduce the likelihood of classification errors. Finally, we evaluate the proposed method using a widely used remote sensing dataset. Our experimental results demonstrate that our approach outperforms benchmark methods, achieving improved classification accuracy.
更多查看译文
关键词
remote sensing scene classification,few-shot learning,data augmentation,feature distortion
AI 理解论文
溯源树
样例
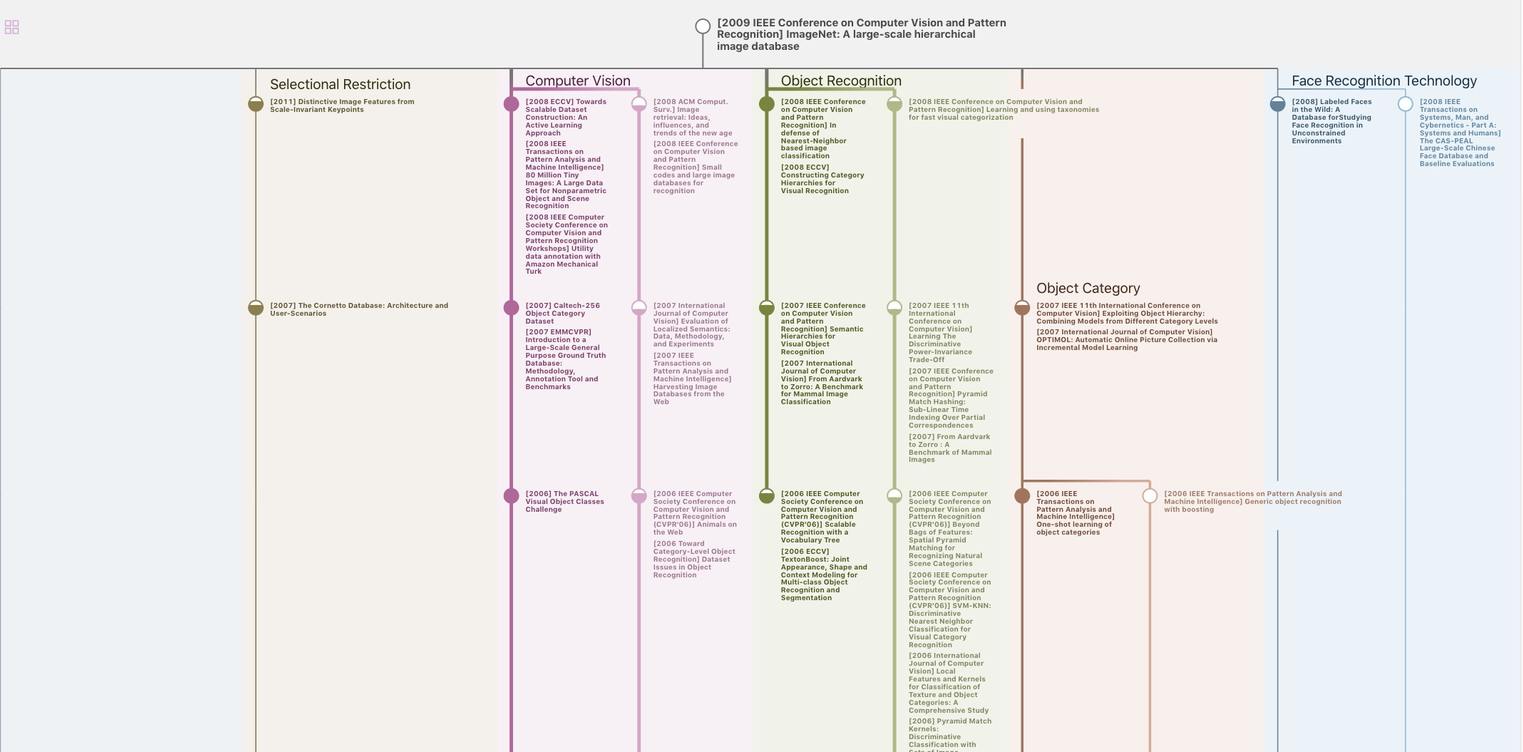
生成溯源树,研究论文发展脉络
Chat Paper
正在生成论文摘要