From "Thumbs Up" to "10 out of 10": Reconsidering Scalar Feedback in Interactive Reinforcement Learning
2023 IEEE/RSJ INTERNATIONAL CONFERENCE ON INTELLIGENT ROBOTS AND SYSTEMS, IROS(2023)
摘要
Learning from human feedback is an effective way to improve robotic learning in exploration-heavy tasks. Compared to the wide application of binary human feedback, scalar human feedback has been used less because it is believed to be noisy and unstable. In this paper, we compare scalar and binary feedback, and demonstrate that scalar feedback benefits learning when properly handled. We collected binary or scalar feedback respectively from two groups of crowdworkers on a robot task. We found that when considering how consistently a participant labeled the same data, scalar feedback led to less consistency than binary feedback; however, the difference vanishes if small mismatches are allowed. Additionally, scalar and binary feedback show no significant differences in their correlations with key Reinforcement Learning targets. We then introduce Stabilizing TEacher Assessment DYnamics (STEADY) to improve learning from scalar feedback. Based on the idea that scalar feedback is muti-distributional, STEADY re-constructs underlying positive and negative feedback distributions and re-scales scalar feedback based on feedback statistics. We show that models trained with scalar feedback + STEADY outperform baselines, including binary feedback and raw scalar feedback, in a robot reaching task with non-expert human feedback. Our results show that both binary feedback and scalar feedback are dynamic, and scalar feedback is a promising signal for use in interactive Reinforcement Learning.
更多查看译文
AI 理解论文
溯源树
样例
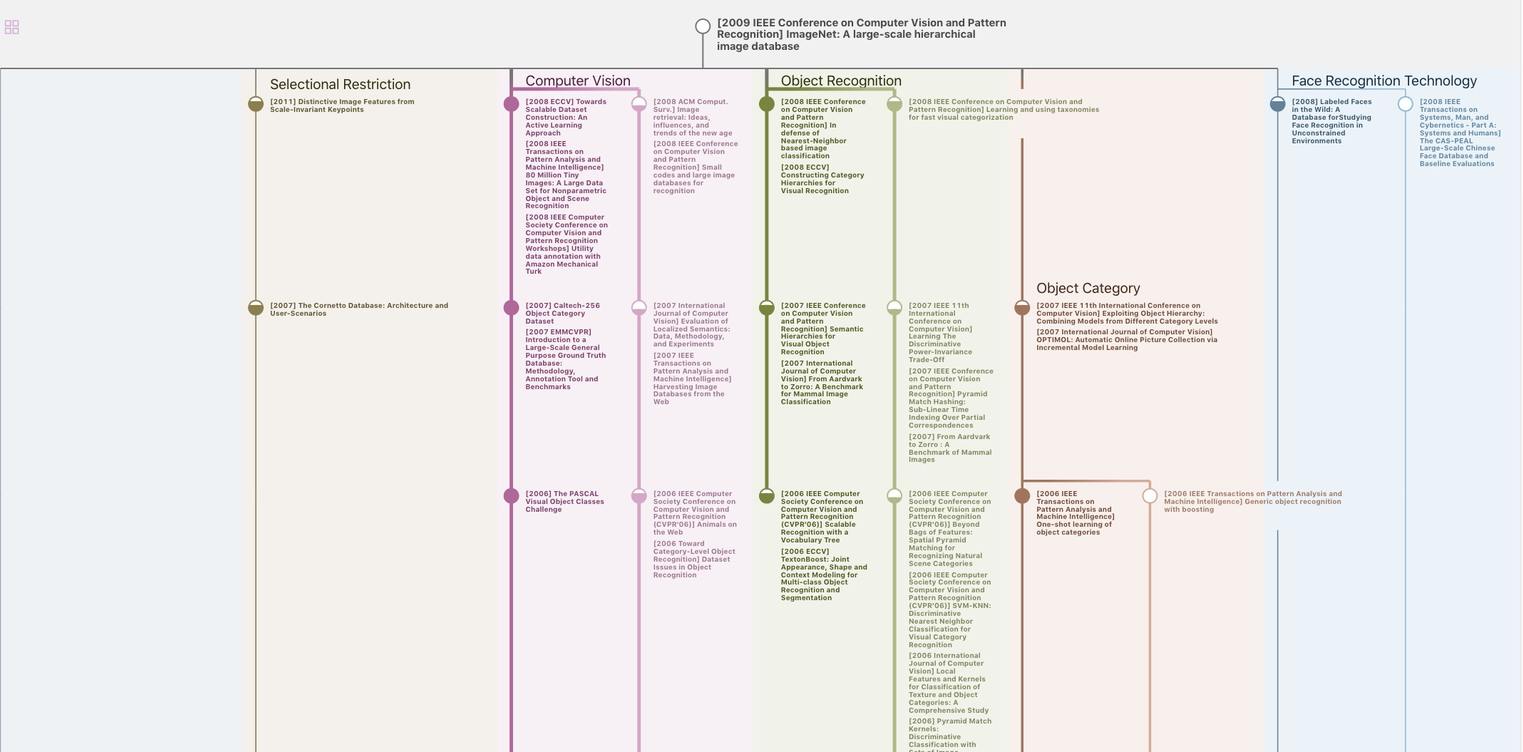
生成溯源树,研究论文发展脉络
Chat Paper
正在生成论文摘要