PEPNet: Parameter and Embedding Personalized Network for Infusing with Personalized Prior Information
PROCEEDINGS OF THE 29TH ACM SIGKDD CONFERENCE ON KNOWLEDGE DISCOVERY AND DATA MINING, KDD 2023(2023)
摘要
With the increase of content pages and interactive buttons in online services such as online-shopping and video-watching websites, industrial-scale recommender systems face challenges in multi-domain and multi-task recommendations. The core of multi-task and multi-domain recommendation is to accurately capture user interests in multiple scenarios given multiple user behaviors. In this paper, we propose a plug-and-play Parameter and Embedding Personalized Network (PEPNet) for multi-domain and multi-task recommendation. PEPNet takes personalized prior information as input and dynamically scales the bottom-level Embedding and top-level DNN hidden units through gate mechanisms. Embedding Personalized Network (EPNet) performs personalized selection on Embedding to fuse features with different importance for different users in multiple domains. Parameter Personalized Network (PPNet) executes personalized modification on DNN parameters to balance targets with different sparsity for different users in multiple tasks. We have made a series of special engineering optimizations combining the Kuaishou training framework and the online deployment environment. By infusing personalized selection of Embedding and personalized modification of DNN parameters, PEPNet tailored to the interests of each individual obtains significant performance gains, with online improvements exceeding 1% in multiple task metrics across multiple domains. We have deployed PEPNet in Kuaishou apps, serving over 300 million users every day.
更多查看译文
关键词
Multi-Domain Learning,Multi-Task Learning,Personalization,Recommender System
AI 理解论文
溯源树
样例
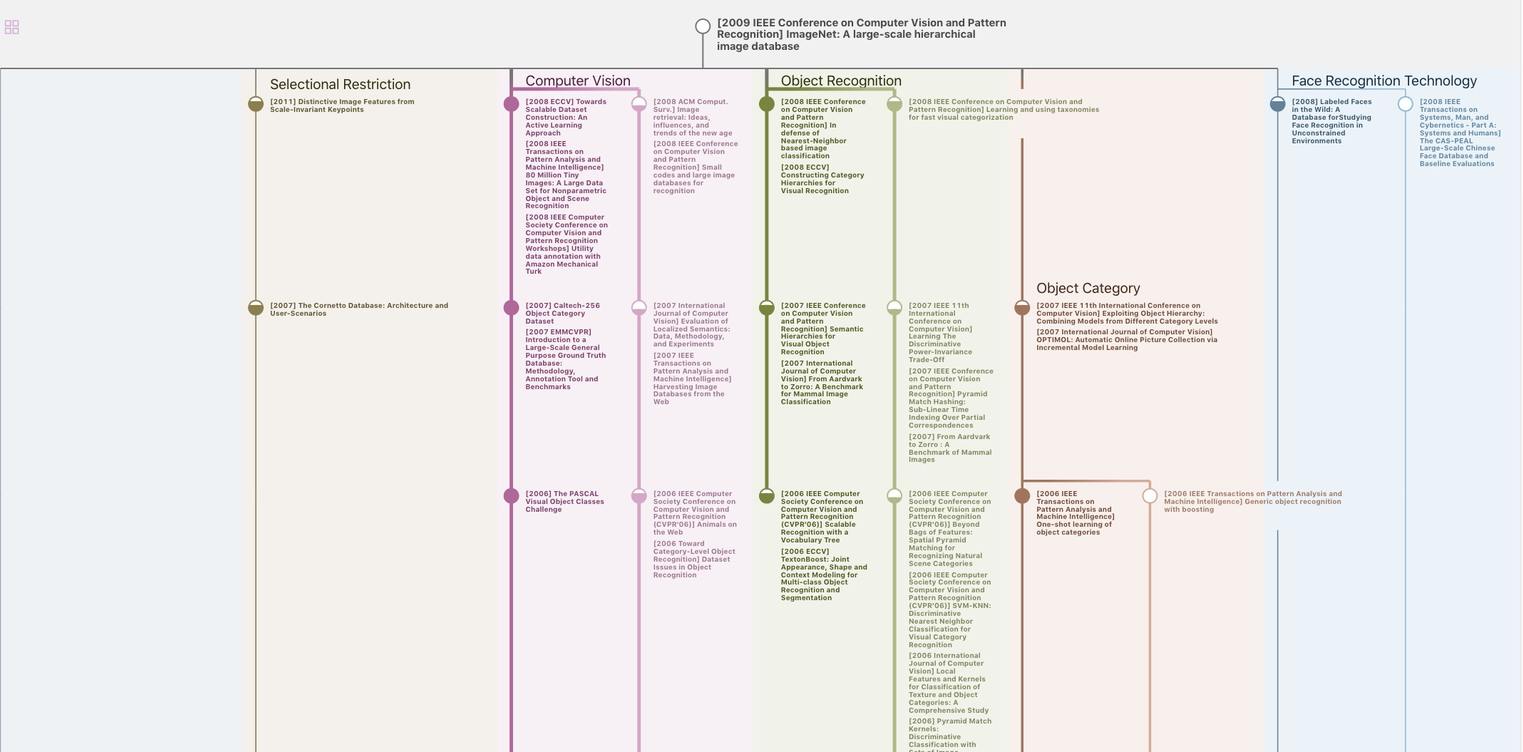
生成溯源树,研究论文发展脉络
Chat Paper
正在生成论文摘要