Appraising the Risk Assessment of Non-Structural Components via Simplified and Machine-Learning-Based Approaches
JOURNAL OF EARTHQUAKE ENGINEERING(2024)
摘要
Uncertainties in building element modelling and ground motions are indispensable for rigorous seismic risk quantification. demand-intensity models simply relate building performance to intensity and subsequently risk. This article describes developments toward non-structural element (NSEs) risk quantification. The demand-intensity models are applied to infilled reinforced concrete (RC) case-study buildings. Implicit and explicit NSE numerical modelling is used to validate the models versus the direct integration of risk exceedance. For faster estimation techniques, machine learning models are trained to estimate the demand-intensity model fitting parameters on a modest dataset of RC infilled buildings. Among these, the extreme gradient boosting (XGBoost) demonstrated superior performance, indicating further possible directions for improvement where larger datasets are available. These models facilitate the simple retrieving of demand-intensity models without extensive structural analysis and can be utilized for both structural and non-structural elements when assessing risk in single or multiple buildings as part of portfolio analysis.
更多查看译文
关键词
Seismic risk,non-structural elements,demand-intensity,RC buildings,machine learning
AI 理解论文
溯源树
样例
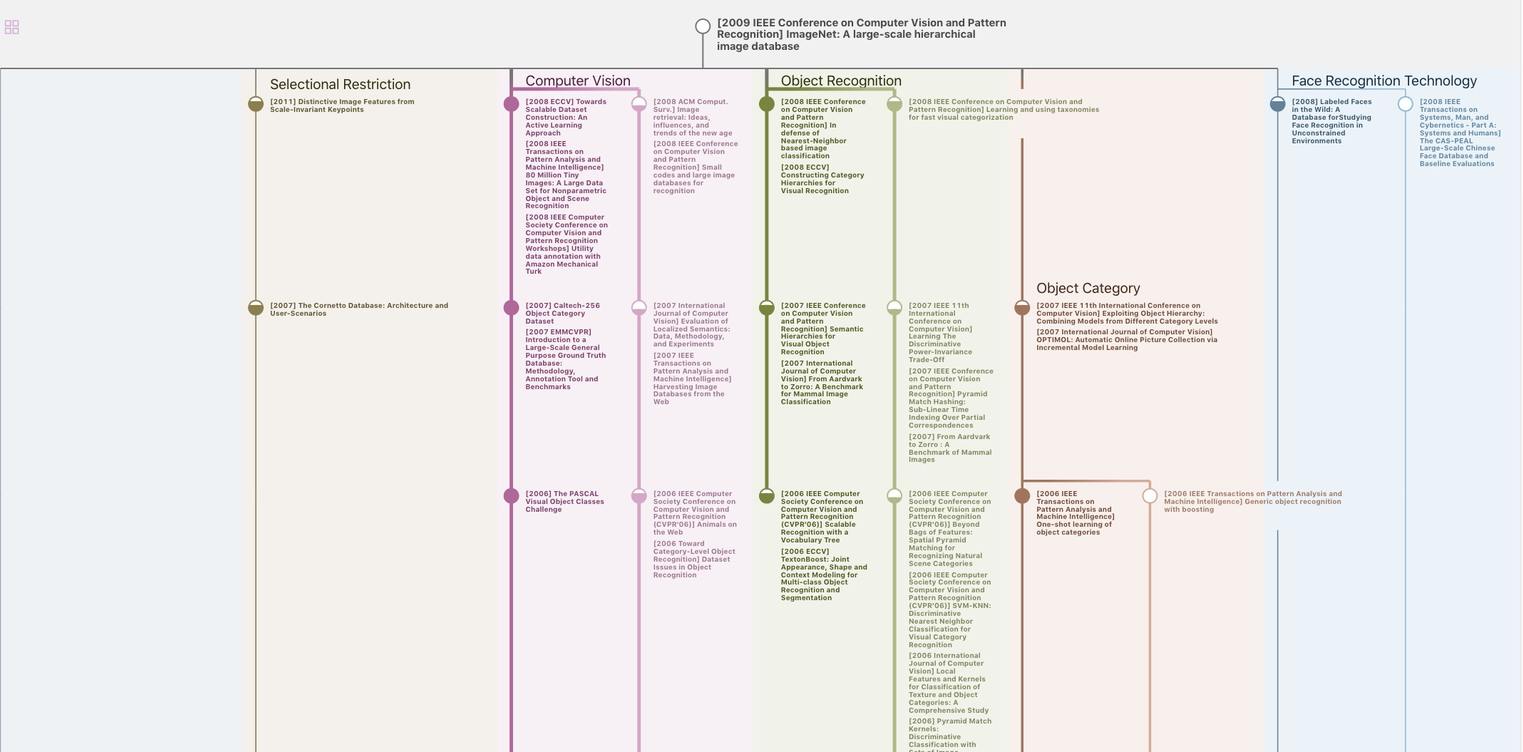
生成溯源树,研究论文发展脉络
Chat Paper
正在生成论文摘要