Two-Stage Point Cloud Registration Framework Based on Graph Neural Network and Attention
ELECTRONICS(2024)
摘要
In recent years, due to the wide application of 3D vision in the fields of autonomous driving, robot navigation, and the protection of cultural heritage, 3D point cloud registration has received much attention. However, most current methods are time-consuming and are very sensitive to noises and outliers, resulting in low registration accuracy. Therefore, we propose a two-stage framework based on graph neural network and attention-TSGANet, which is effective in registering low-overlapping point cloud pairs and is robust to variable noises as well as outliers. Our method decomposes rigid transformation estimation into two stages: global estimation and fine-tuning. At the global estimation stage, multilayer perceptrons are employed to estimate a seven-dimensional vector representing rigid transformation directly from the fusion of two initial point cloud features. For the fine-tuning stage, we extract contextual information through an attentional graph neural network consisting of attention and feature-enhancing modules. A mismatch-suppression mechanism is also proposed and applied to keep our method robust to partially visible data with noises and outliers. Experiments show that our method yields a state-of-the-art performance on the ModelNet40 dataset.
更多查看译文
关键词
point cloud registration,deep learning,graph neural network,attention
AI 理解论文
溯源树
样例
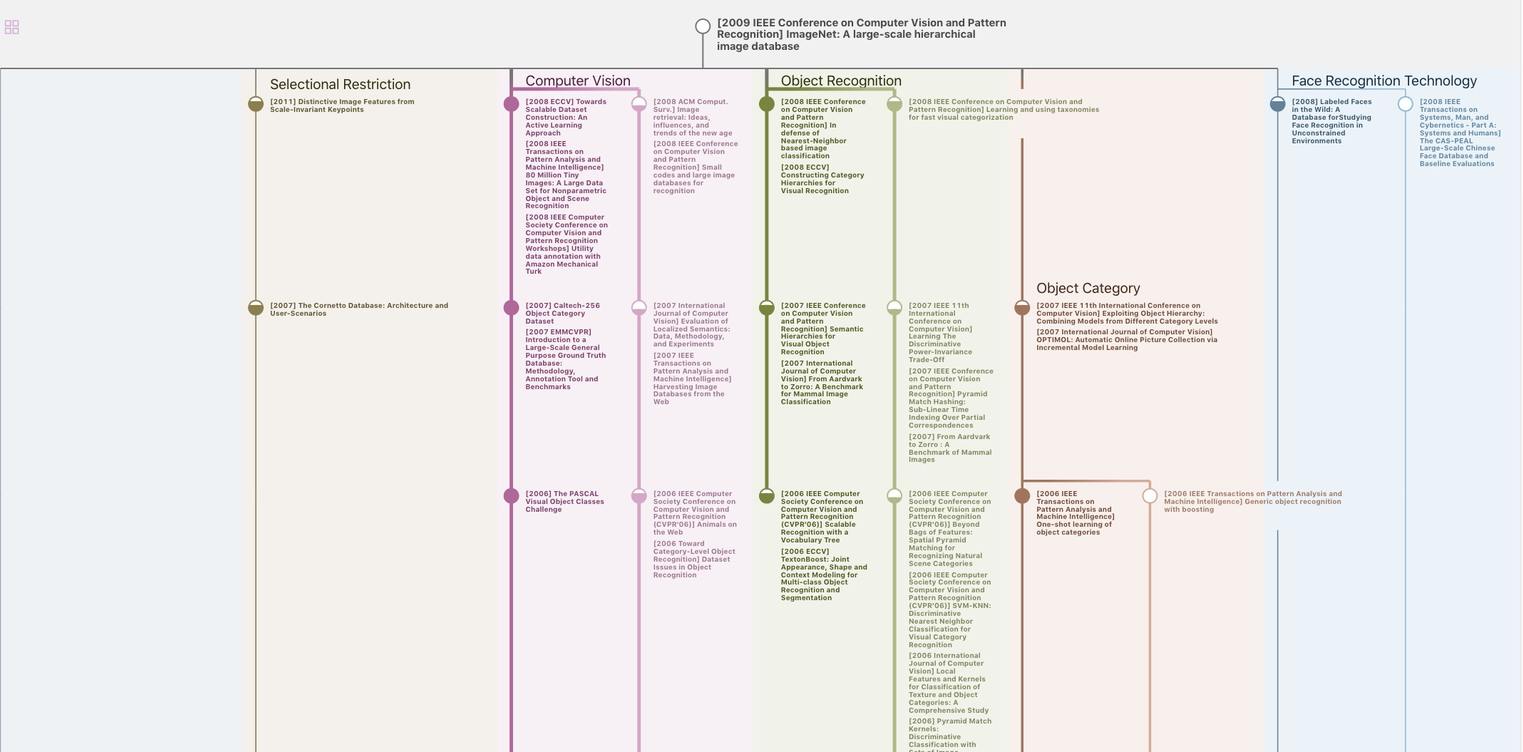
生成溯源树,研究论文发展脉络
Chat Paper
正在生成论文摘要