Optimizing Geo-Hazard Response: LBE-YOLO's Innovative Lightweight Framework for Enhanced Real-Time Landslide Detection and Risk Mitigation
REMOTE SENSING(2024)
摘要
Prompt detection of landslides is crucial for reducing the disaster risk and preventing landslides. However, landslide detection in practical applications still faces many challenges, such as the complexity of environmental backgrounds, the diversity of target scales, and the enormity of model weights. To address these issues, this paper proposes a lightweight LBE-YOLO model for real-time landslide detection. Firstly, a lightweight model is designed by integrating the GhostConv lightweight network with the YOLOv8n model. Inspired by GhostConv, this study innovatively designed the GhostC2f structure, which leverages linear thinking to further reduce the model parameters and computational burden. Additionally, the newly designed EGC2f structure, incorporating an attention mechanism, not only maintains the model's lightweight characteristics but also enhances the network's capability to extract valid information. Subsequently, the Path Aggregation Network (PAN) was optimized by introducing a bidirectional feature propagation mechanism to improve the model's feature fusion ability. Additionally, the Bijie landslide dataset was expanded through data augmentation strategies, thereby further improving the model's generalization capability. The experimental results indicate that, compared to the YOLOv8n model, the proposed model increased accuracy by 4.2%, while the model's weight and computational load were reduced by 32.0% and 35.5%, respectively. This verifies the superiority of the LBE-YOLO model in landslide target detection, which will help mitigate the impacts of natural disasters.
更多查看译文
关键词
attention mechanism,landslide detection,feature fusion,GhostConv,YOLOv8n
AI 理解论文
溯源树
样例
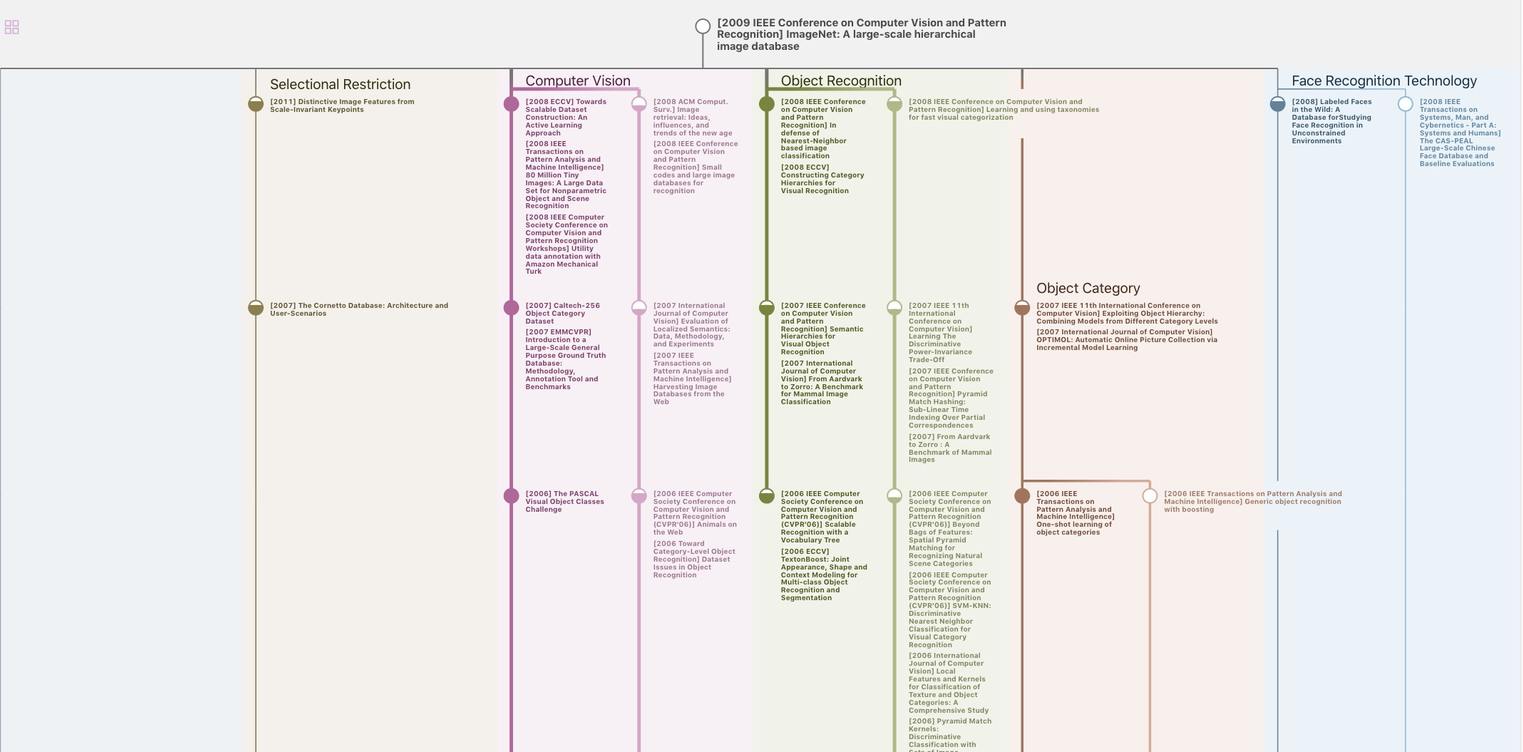
生成溯源树,研究论文发展脉络
Chat Paper
正在生成论文摘要