An Investigation of LES Wall Modeling for Rayleigh-Benard Convection via Interpretable and Physics-Aware Feedforward Neural Networks with DNS
JOURNAL OF THE ATMOSPHERIC SCIENCES(2024)
摘要
The traditional approach of using the Monin-Obukhov similarity theory (MOST) to model near -surface processes in large -eddy simulations (LESs) can lead to significant errors in natural convection. In this study, we propose an alternative approach based on feedforward neural networks (FNNs) trained on output from direct numerical simulation (DNS). To evaluate the performance, we conduct both a priori and a posteriori tests. In the a priori (offline) tests, we compare the statistics of the surface shear stress and heat flux, computed from filtered DNS input variables, to the stress and flux obtained from the filtered DNS. Additionally, we investigate the importance of various input features using the Shapley additive explanations value and the conditional average of the filter grid cells. In the a posteriori (online) tests, we implement the trained models in the System for Atmospheric Modeling (SAM) LES and compare the LES-generated surface shear stress and heat flux with those in the DNS. Our findings reveal that vertical velocity, a traditionally overlooked flow quantity, is one of the most important input features for determining the wall fluxes. Increasing the number of input features improves the a priori test results but does not always improve the model performance in the a posteriori tests because of the differences in input variables between the LES and DNS. Last, we show that physics -aware FNN models trained with logarithmic and scaled parameters can well extrapolate to more intense convection scenarios than in the training dataset, whereas those trained with primitive flow quantities cannot.
更多查看译文
关键词
Convection,Turbulence,Boundary layer,Large eddy simulations,Subgrid-scale processes,Machine learning
AI 理解论文
溯源树
样例
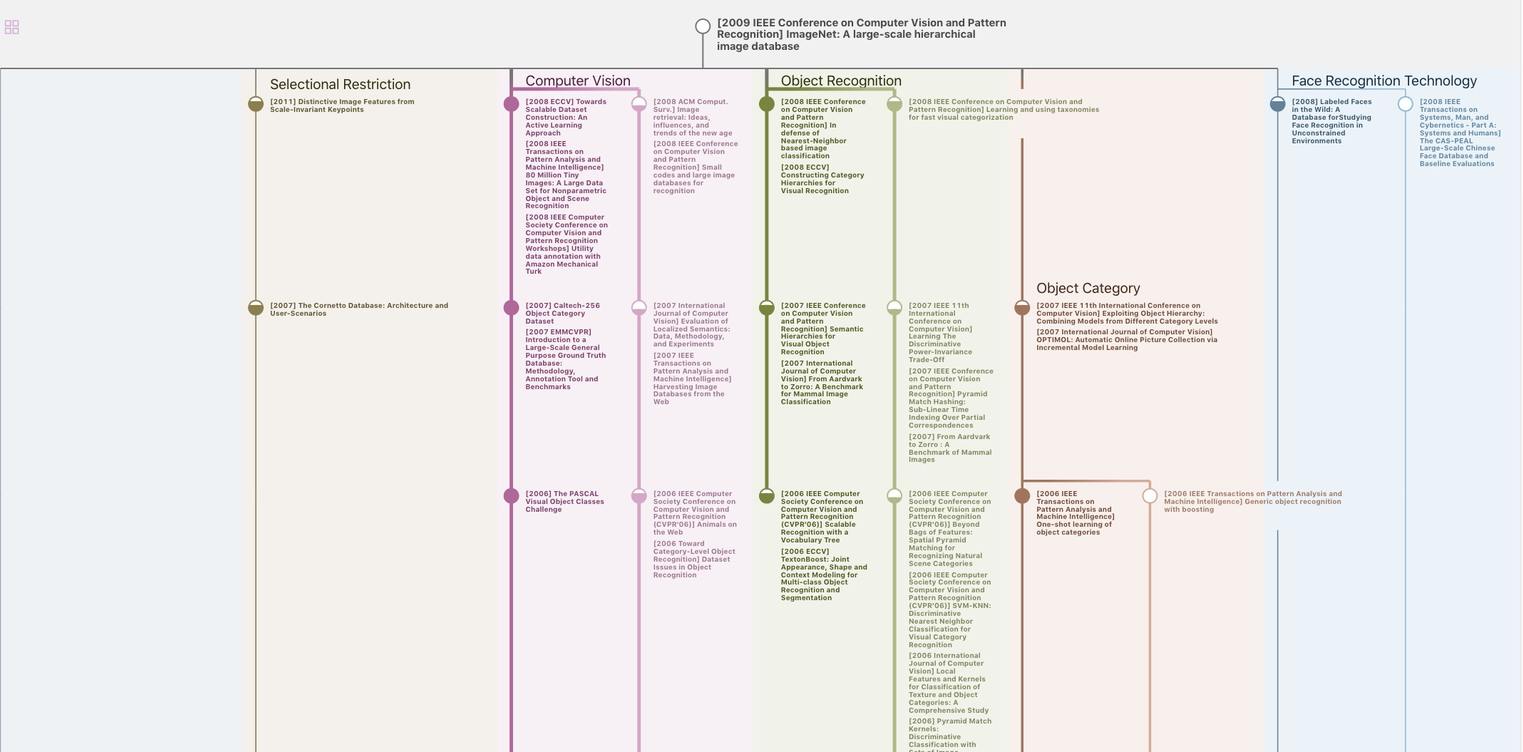
生成溯源树,研究论文发展脉络
Chat Paper
正在生成论文摘要