Comparing three machine learning algorithms with existing methods for natural streamflow estimation
HYDROLOGICAL SCIENCES JOURNAL(2024)
摘要
Natural streamflow data is required in many hydrological applications. However, many basins are located in data-scarce regions or are impacted by human construction and activities. In this paper, we explore three machine learning algorithms, namely artificial neural networks, random forest and light gradient boosting machine, to simultaneously estimate all the parameters of the coupled modele du Genie Rural a 4 parametres Journaliers (GR4J) and snow accounting routine called CemaNeige model. A database of 675 basins in the USA and Quebec is used to train and test ensembles. After using the estimated parameters in GR4J, the resulting naturalized streamflow series are compared with those obtained by the established drainage area ratio and spatial proximity transfer methods in 11 test basins. The results indicate that the machine learning algorithms outperform the drainage area ratio and spatial proximity transfer methods. Among machine learning algorithms, random forests obtain lower (better) continuous ranked probability scores than the other methods for 10 out of 11 test basins.
更多查看译文
关键词
neural networks,random forest,light gradient boosting machine,drainage area ratio method,spatial proximity transfer
AI 理解论文
溯源树
样例
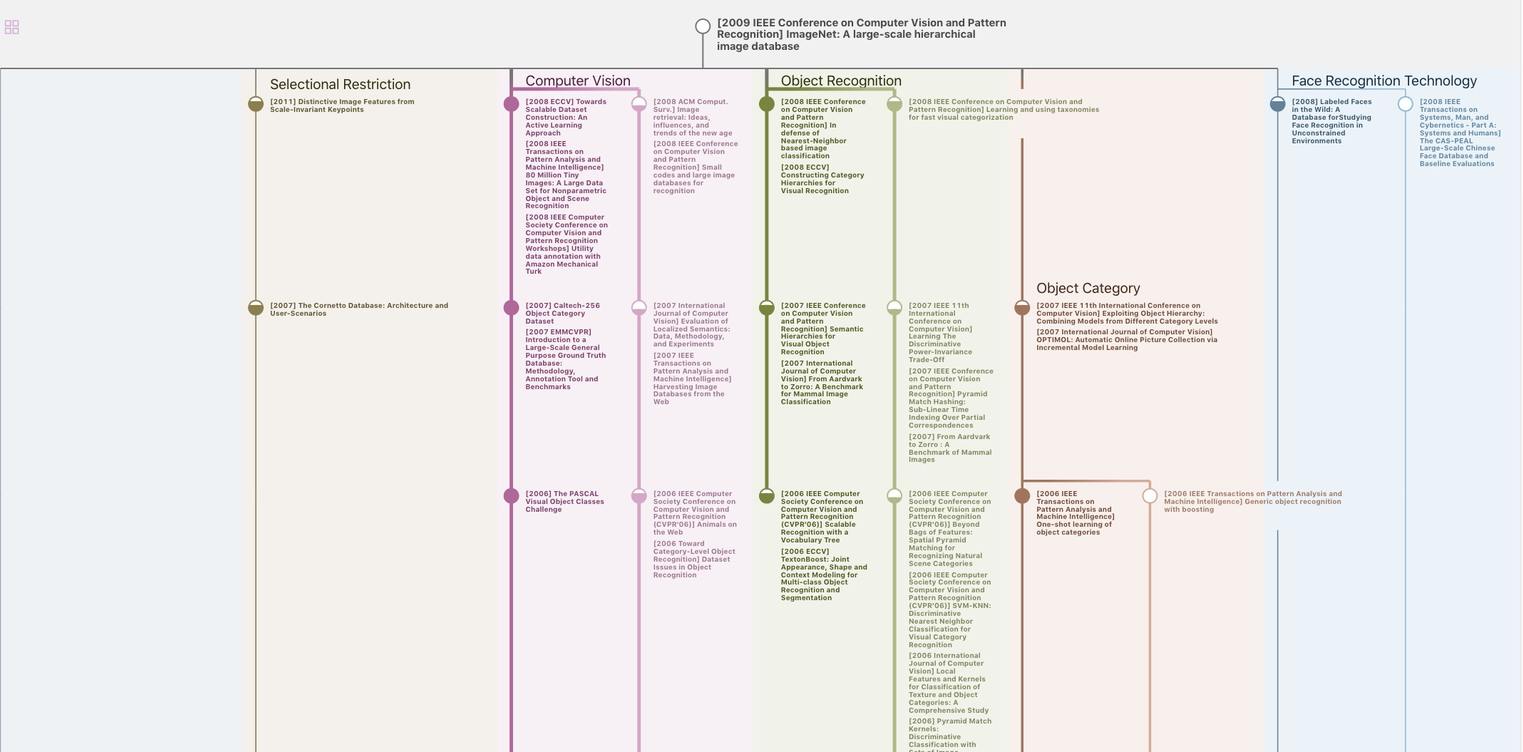
生成溯源树,研究论文发展脉络
Chat Paper
正在生成论文摘要