BERD plus : A Generic Sequential Recommendation Framework by Eliminating Unreliable Data with Item- and Attribute-level Signals
ACM TRANSACTIONS ON INFORMATION SYSTEMS(2024)
摘要
Most sequential recommendation systems (SRSs) predict the next item as the target for users given its preceding items as input, assuming the target is definitely related to its input. However, users may unintentionally click items that are inconsistent with their preference due to external factors, causing unreliable instances whose target mismatches the input. We, for the first time, verify SRSs can be misguided by such unreliable instances and design a generic SRS framework By Eliminating unReliable Data (BERD+), which can be flexibly plugged into existing SRSs. Specifically, BRED+ is guided with observations on the training process of instances: Unreliable instances generally have high training loss; high-loss instances are not necessarily unreliable but uncertain ones caused by blurry sequential patterns; and item attributes help rectify instance loss and uncertainty, but may also introduce disturbance. Accordingly, BERD+ models both the loss and uncertainty of each instance via a Gaussian distribution, whereby a heterogeneous uncertainty-aware graph convolution network is designed to learn accurate embeddings for different entities while reducing the disturbance caused by uncertain attribute values. Thereafter, an explicit preference extractor rectifies instance loss and uncertainty and reduces the disturbance caused by less-focused attribute types. Finally, instances with high loss and low uncertainty are eliminated as unreliable data. Extensive experiments verify the efficacy of BERD+.
更多查看译文
关键词
Sequential recommender systems,unreliable instances,heterogeneous graph,graph convolution network
AI 理解论文
溯源树
样例
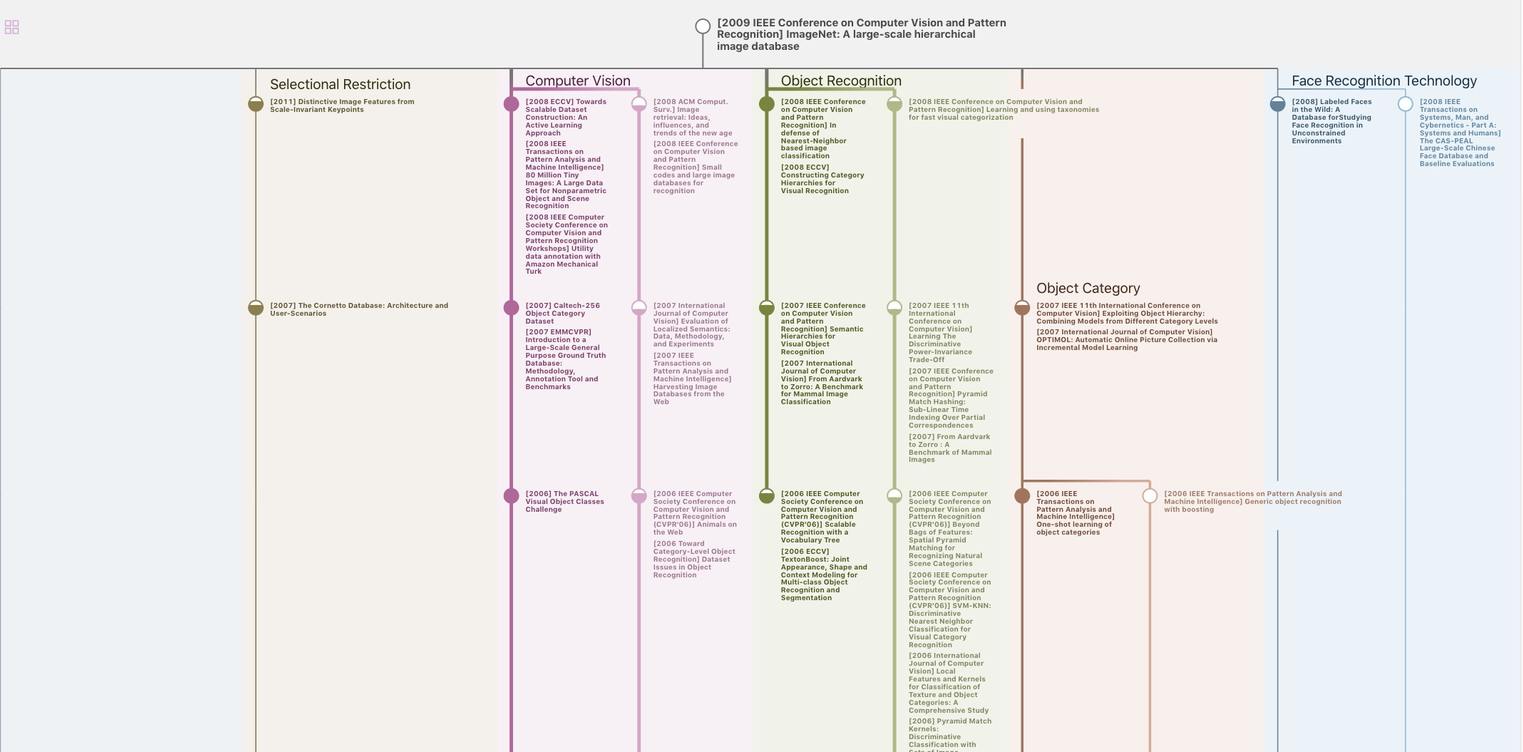
生成溯源树,研究论文发展脉络
Chat Paper
正在生成论文摘要