The impact of imputation methods on the performance of Phase I Hotelling's T2 control chart
COMMUNICATIONS IN STATISTICS-SIMULATION AND COMPUTATION(2024)
摘要
The objective of this study was to evaluate the impact of three different methods of handling missing data on the performance of Phase I Hotelling's T-2 multivariate control chart. Using a Monte Carlo simulation, we studied the average, median, and standard deviation of the run length performance of multivariate data imputed using mean substitution, regression imputation, and predictive mean matching at three different levels of missingness (1%, 10%, and 25%) and three levels of variable correlation coefficients (0.2, 0.4, and 0.8). We found that predictive mean matching has average run length performance results comparable to that of the complete in-control data set at all levels of missingness and variable correlation, while the performance of mean substitution was adversely affected by high levels of missingness and by strong variable correlation. Based on the simulation (multivariate normal data), we concluded that predictive mean matching is superior to both regression imputation and mean substitution as a method for imputing missing values for the analysis of Phase I Hotelling's T2 control chart. Two applications were presented using the Altenrhein wastewater treatment plant and Olive oil datasets.
更多查看译文
关键词
T-2 Hotelling's imputation methods,Missing data,Predictive mean matching
AI 理解论文
溯源树
样例
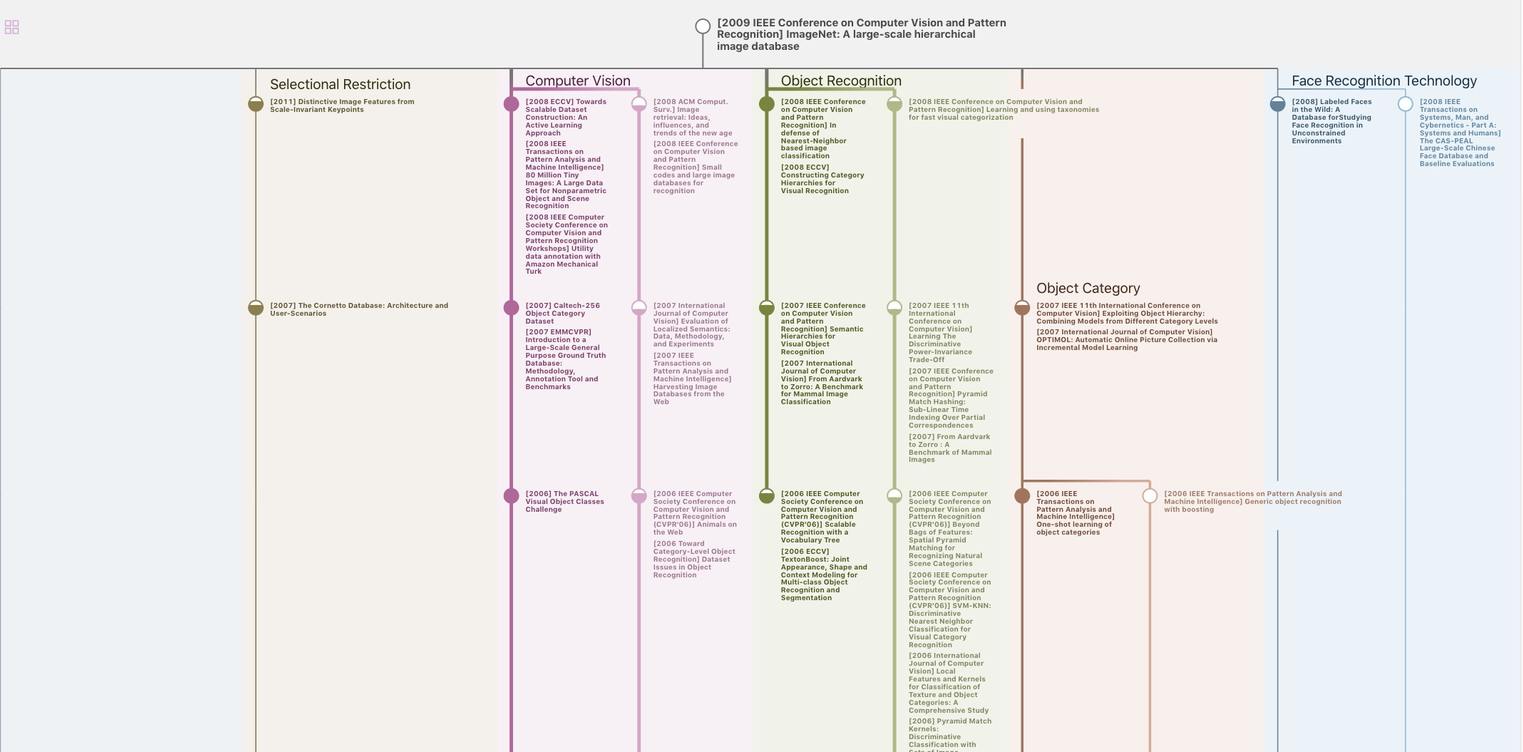
生成溯源树,研究论文发展脉络
Chat Paper
正在生成论文摘要