Machine Learning-Based Prediction of the CO2 Concentration in the Flue Gas and Carbon Emissions from a Waste Incineration Plant
ACS ES&T ENGINEERING(2024)
摘要
Monitoring the CO2 concentration in flue gas (CO2_G) is crucial to accurately calculate the direct carbon emissions associated with waste incineration. In this study, random forest (RF) and extreme gradient boosting (XGBoost) algorithms were used to predict CO2_G, using 21 operating variables from a municipal solid waste (MSW) incineration plant as input variables. The results showed a strong prediction performance for both the RF and XGBoost-based models with R-2 values of 0.932 and 0.903, respectively. A feature importance analysis identified key variables used for model retraining, resulting in R-2 values of 0.917 and 0.894, respectively. Based on the predicted and measured values of CO2_G and a balance calculation, the direct carbon emissions from waste incineration were determined. The emissions based on the predicted CO2_G value ranged from 283.38 to 348.39 kgCO(2)-eq/t, while the emission based on the measured value was 269.21 kgCO(2)-eq/t. To further validate the accuracy of the calculation results, the physical composition of MSW in the incineration plant was analyzed, resulting in a direct carbon emission estimate of 257.59 kgCO(2)-eq/t. These findings demonstrate the effective application of machine learning (ML)-based CO2_G predictions and overcome the labor-intensive and data-lagging aspects of carbon emission accounting in waste incineration.
更多查看译文
关键词
random forest,extreme gradient boosting,municipalsolid waste incineration,greenhouse gas emission,balance method
AI 理解论文
溯源树
样例
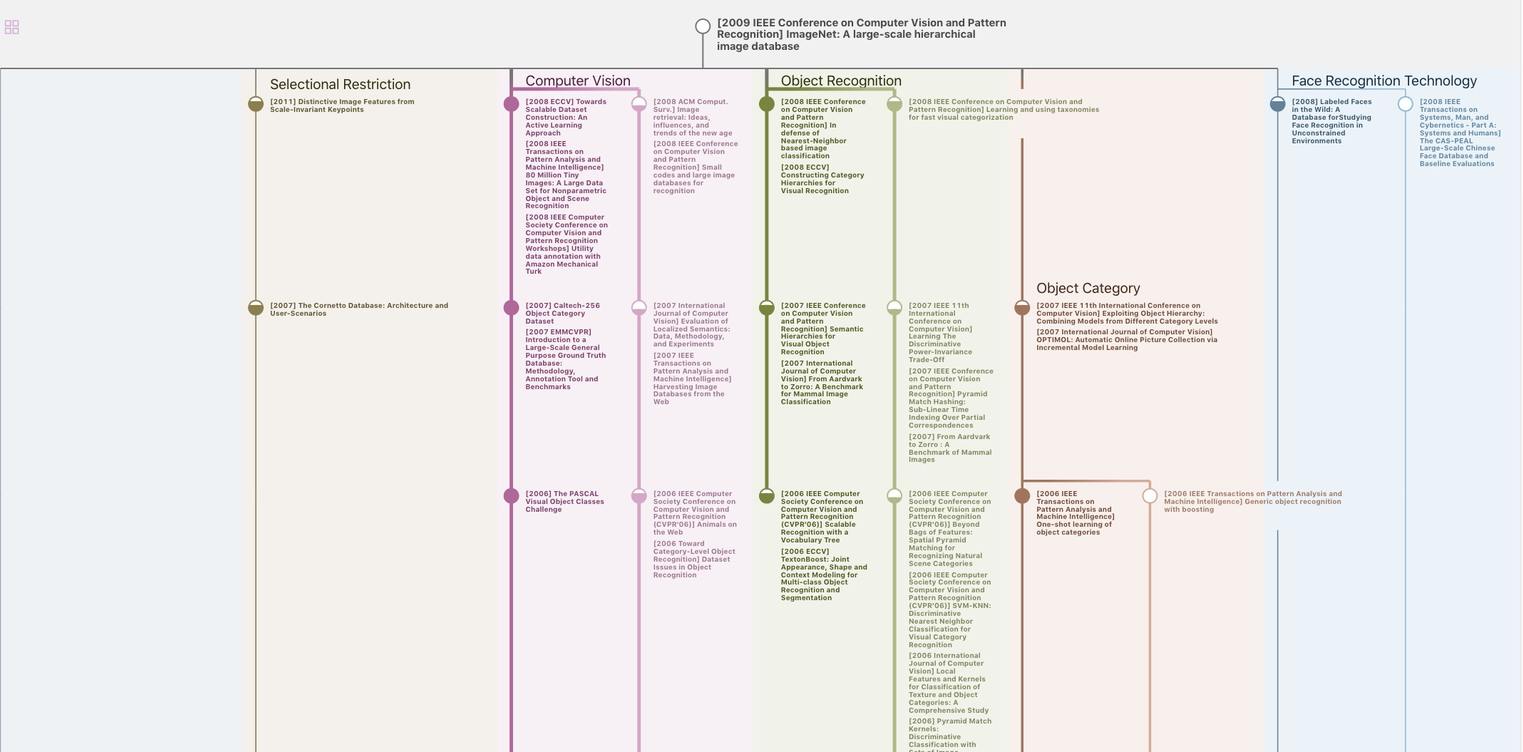
生成溯源树,研究论文发展脉络
Chat Paper
正在生成论文摘要