An improved solution for total least squares collocation in the EIV-model with random effects
ENGINEERING RESEARCH EXPRESS(2024)
摘要
The total least squares (TLS) method within the Errors-In-Variables (EIV) model has been comprehensively investigated in measurement and its relevant fields. However, when prior information is considered, existing solutions in the EIV model with random effects (EIV-REM) have many shortcomings, which limit the application of this approach. We have developed the total least squares collocation (TLSC) solution based on general adjustment, where prior information is added to the functional model in the form of pseudo-observations. The accuracy of the estimated parameters is evaluated, and our TLSC solution is converted into a recursive form. The proposed methodology is applied to a 3D datum transformation and an autoregression model. The results for the datum transformation show that our proposed TLSC solution provides more precise estimates than the traditional least squares (LS) and TLS methods. The results for the autoregression model show that TLSC yields similar performance to the batch solution of TLS, but has advantages in terms of computational complexity.
更多查看译文
关键词
total least squares collocation (TLSC),prior information,errors-in-variables (EIV) model,general adjustment,accuracy assessment
AI 理解论文
溯源树
样例
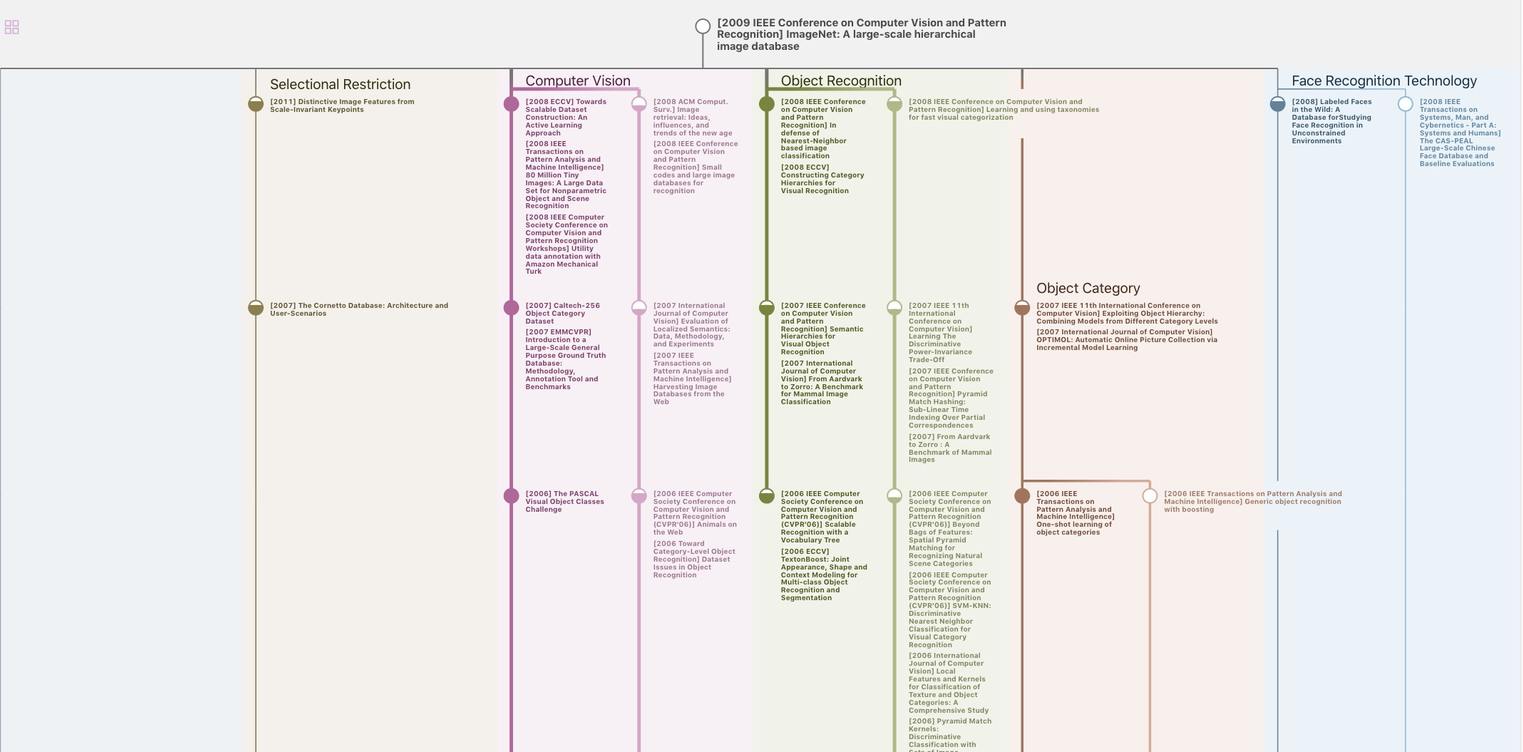
生成溯源树,研究论文发展脉络
Chat Paper
正在生成论文摘要