Improved lightweight federated learning network for fault feature extraction of reciprocating machinery
MEASUREMENT SCIENCE AND TECHNOLOGY(2024)
摘要
The working environment of reciprocating machinery is complex, characterized by nonlinear and non-stationary signals. Deep learning can solve the above problems, but it has its own problems such as complex model and large amount of parameters. Additionally, privacy considerations among enterprises prevent data sharing, leading to the emergence of 'data islands' and inadequate training of deep learning models. Based on the above analysis, this paper proposes a reciprocating mechanical feature extraction method based on an improved federated lightweight network. A lightweight network SqueezeNet model is used to solve the problems such as long training time of deep learning. By establishing a federated learning framework, the reciprocating mechanical data can be collectively diagnosed across various enterprises, thereby addressing the problem of limited model training caused by insufficient data. Furthermore, to enhance the accuracy of network training and diagnosis, modifications are made to the SqueezeNet network to reduce the number of model parameters while increasing the number and variety of feature extractions. Experimental results demonstrate that when the number of 1 x 1 and 3 x 3 channels is 1-7, the fault diagnosis accuracy is the highest, about 97.96%, which enriches the categories of feature extraction. The number of parameters in In-SqueezeNet is 56% of that in SqueezeNet network model, and the training time is reduced by nearly 15%. The fault diagnosis accuracy is increased from 95.1% to 97.3%, and the diversity of extracted features is increased. Compared with other network models such as ResNet, the improved lightweight federated learning network has a fault diagnosis accuracy of 96.6%, an improvement of 10.6%. At the same time, the training time was reduced to 1982s, a reduction of about 41.5%. The validity of the proposed model is further verified.
更多查看译文
关键词
reciprocating machinery,federated learning,SqueezeNet,fault diagnosis
AI 理解论文
溯源树
样例
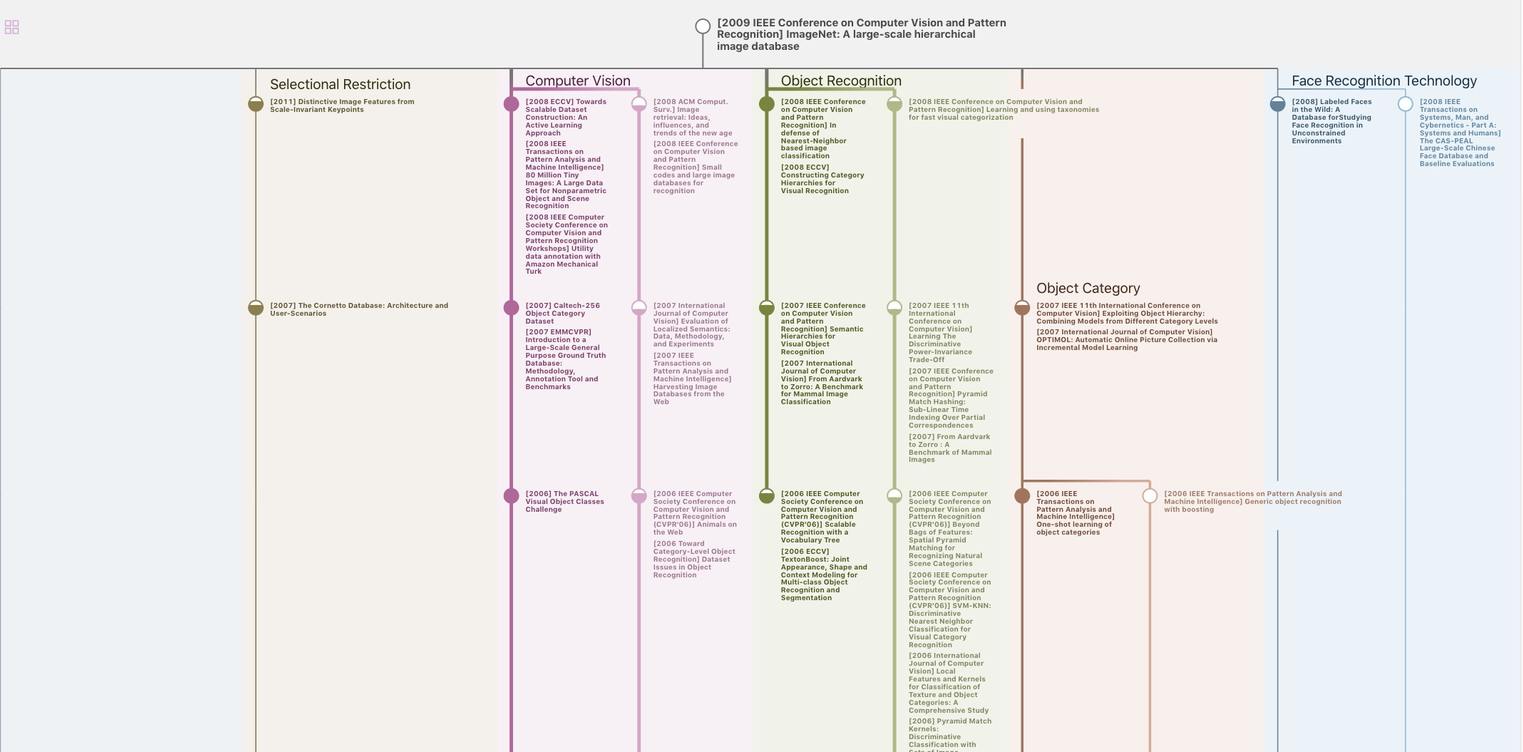
生成溯源树,研究论文发展脉络
Chat Paper
正在生成论文摘要