Remaining Life Prediction of Li-Ion Batteries Considering Sufficiency of Historical Data
JOURNAL OF THE ELECTROCHEMICAL SOCIETY(2024)
摘要
With the development of new batteries, the historical data available for training for remaining useful life (RUL) prediction of li-ion batteries will be greatly reduced, and the capacity regeneration phenomenon (CRP) of batteries will also bring challenges to the prediction. This paper proposes a hybrid model that combines decomposition algorithms incorporating the broad learning system with dropout (BLSD) to predict the RUL of batteries. Firstly, the improved complete ensemble empirical mode decomposition with adaptive noise (ICEEMDAN) method is employed to decompose the raw data into several intrinsic mode function (IMF) which is divided into the global components (GC) and local components (LC) by introducing the Pearson correlation coefficient (PCC). Secondly, considering that traditional BLS methods might exacerbate overfitting and lack the capacity to express uncertainty, Dropout techniques are incorporated into BLS to address these issues. Thirdly, multiple distinct BLSD models are employed to individually train GC and LC, and the summation of multiple predicted values yields the final capacity curve. Finally, the maximum observed root mean square error (RMSE) is 0.006679 when the battery history data is sufficient, and the maximum RMSE is 0.005737 when the battery history data is insufficient, which verifies the validity of the model.
更多查看译文
关键词
ICEEMDAN,BLSD,historical data,capacity regeneration phenomenon,remaining useful life
AI 理解论文
溯源树
样例
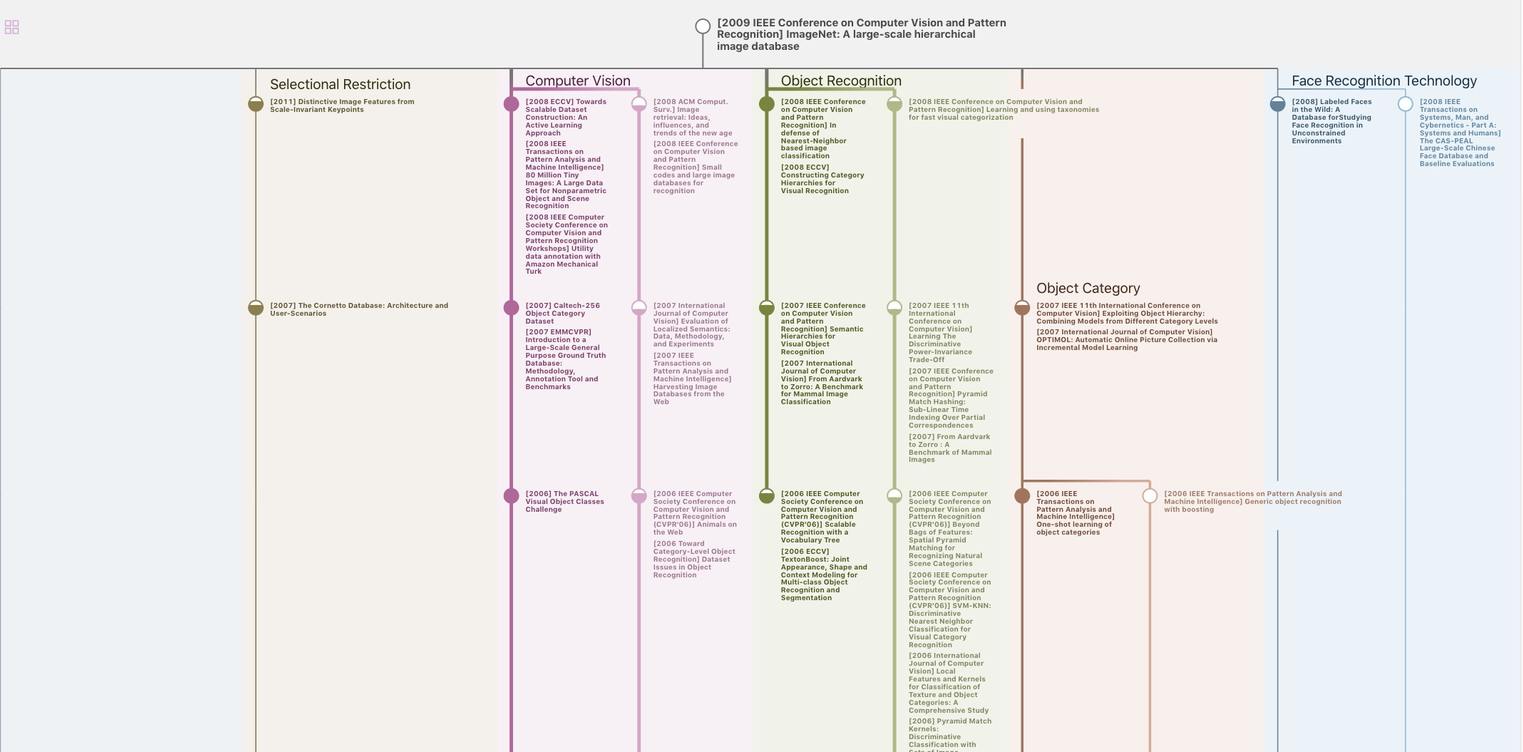
生成溯源树,研究论文发展脉络
Chat Paper
正在生成论文摘要