On bias reduction in parametric estimation in stage structured development models
MONTE CARLO METHODS AND APPLICATIONS(2024)
摘要
Multi-stage models for cohort data are popular statistical models in several fields such as disease progressions, biological development of plants and animals, and laboratory studies of life cycle development. A Bayesian approach on adopting deterministic transformations in the Metropolis-Hastings (MH) algorithm was used to estimate parameters for these stage structured models. However, the biases in later stages are limitations of this methodology, especially the accuracy of estimates for the models having more than three stages. The main aim of this paper is to reduce these biases in parameter estimation. In particular, we conjoin insignificant previous stages or negligible later stages to estimate parameters of a desired stage, while an adjusted MH algorithm based on deterministic transformations is applied for the non-hazard rate models. This means that current stage parameters are estimated separately from the information of its later stages. This proposed method is validated in simulation studies and applied for a case study of the incubation period of COVID-19. The results show that the proposed methods could reduce the biases in later stages for estimates in stage structured models, and the results of the case study can be regarded as a valuable continuation of pandemic prevention.
更多查看译文
关键词
Multi-stage models,stage structured models,stage duration data,stage frequency data,Bayesian analysis,incubation period of COVID-19
AI 理解论文
溯源树
样例
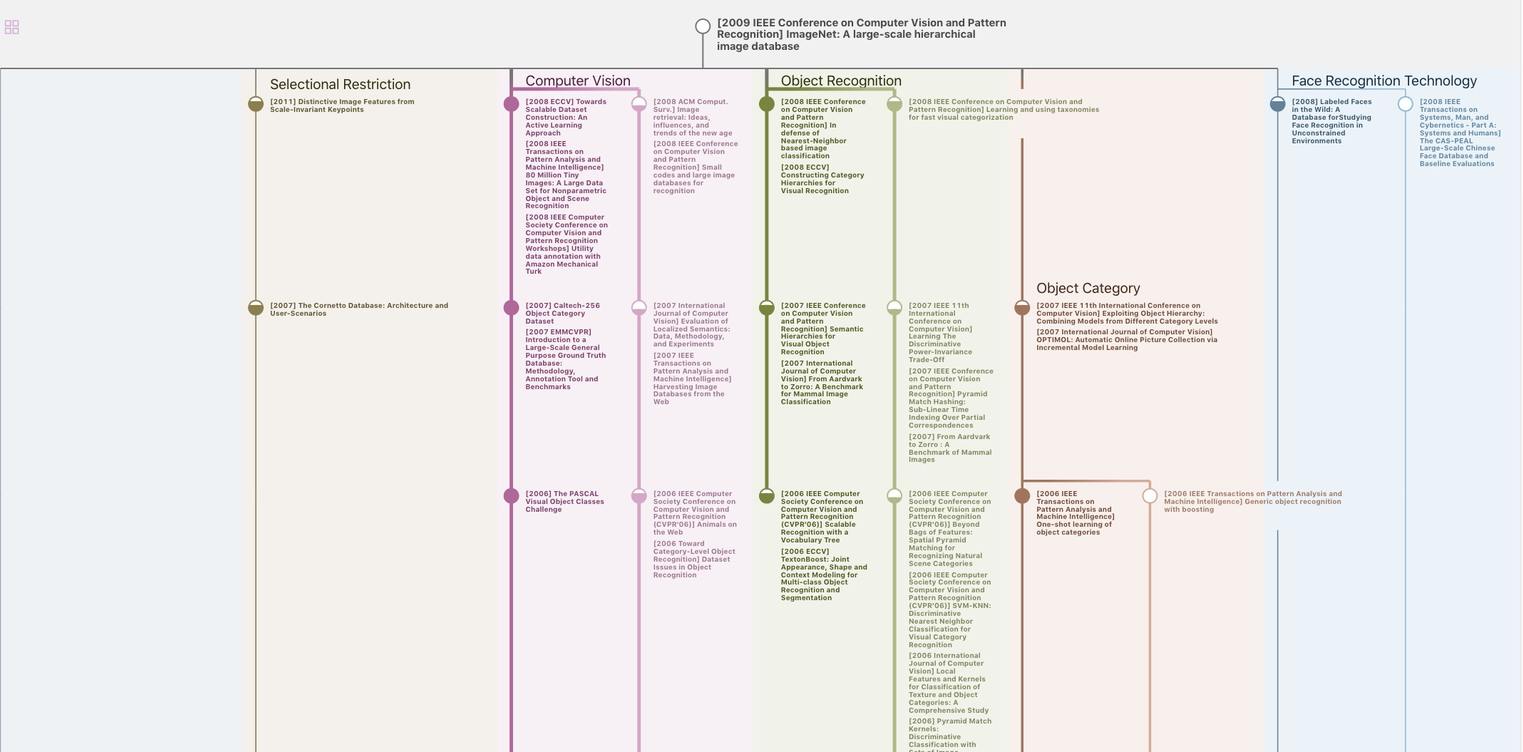
生成溯源树,研究论文发展脉络
Chat Paper
正在生成论文摘要