A Shrinkage Approach to Improve Direct Bootstrap Resampling under Input Uncertainty
INFORMS JOURNAL ON COMPUTING(2024)
Georgia Inst Technol | Columbia Univ | Penn State Univ
Abstract
The goal of this software is to demonstrate the shrinkage bootstrap methods for input uncertainty quantification proposed in "A Shrinkage Approach to Improve Direct Bootstrap Resampling under Input Uncertainty" by Eunhye Song, Henry Lam, and Russell Barton accepted for publication at INFORMS Journal on Computing.
MoreTranslated text
Key words
bootstrap resampling,input uncertainty,nonparametric,simulation,shrinkage
求助PDF
上传PDF
View via Publisher
AI Read Science
AI Summary
AI Summary is the key point extracted automatically understanding the full text of the paper, including the background, methods, results, conclusions, icons and other key content, so that you can get the outline of the paper at a glance.
Example
Background
Key content
Introduction
Methods
Results
Related work
Fund
Key content
- Pretraining has recently greatly promoted the development of natural language processing (NLP)
- We show that M6 outperforms the baselines in multimodal downstream tasks, and the large M6 with 10 parameters can reach a better performance
- We propose a method called M6 that is able to process information of multiple modalities and perform both single-modal and cross-modal understanding and generation
- The model is scaled to large model with 10 billion parameters with sophisticated deployment, and the 10 -parameter M6-large is the largest pretrained model in Chinese
- Experimental results show that our proposed M6 outperforms the baseline in a number of downstream tasks concerning both single modality and multiple modalities We will continue the pretraining of extremely large models by increasing data to explore the limit of its performance
Upload PDF to Generate Summary
Must-Reading Tree
Example
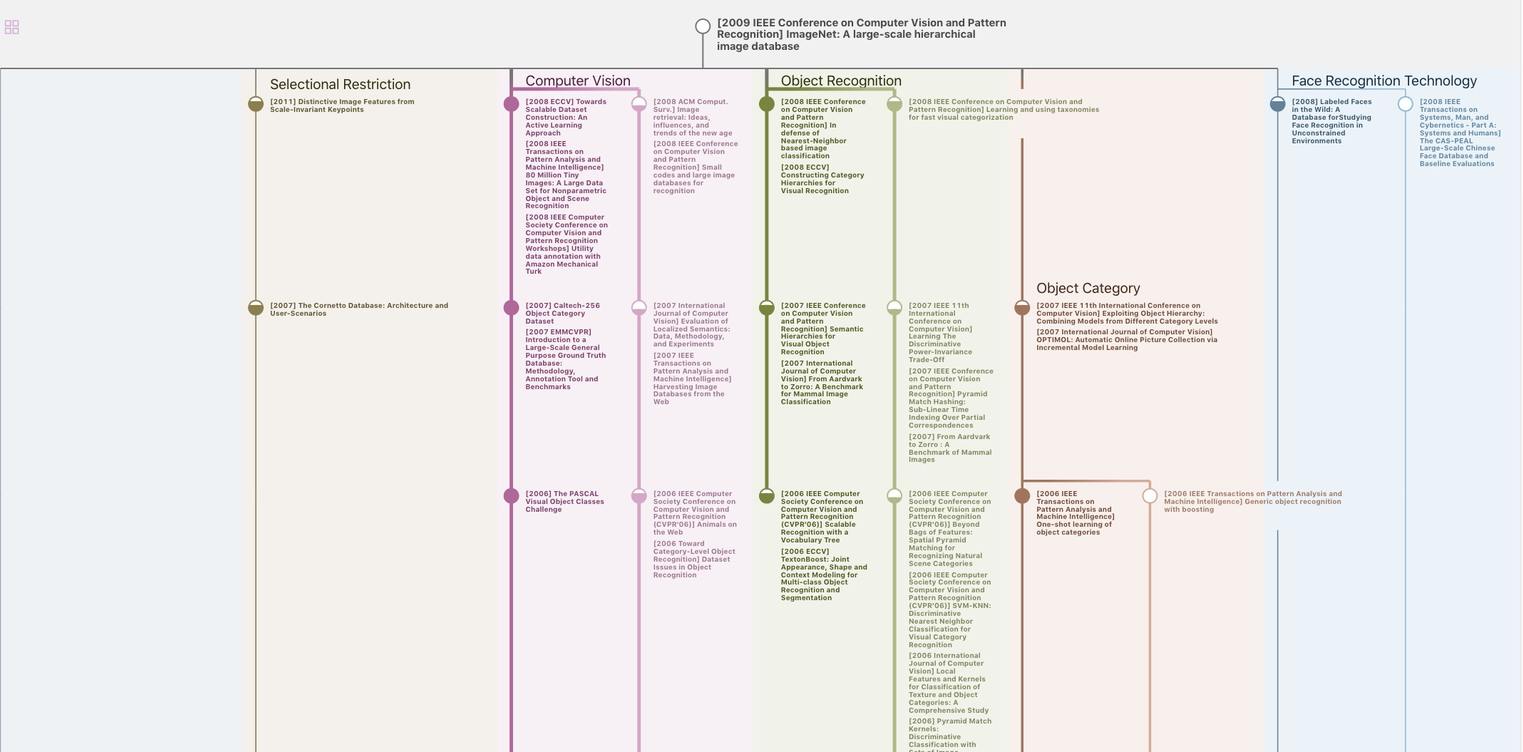
Generate MRT to find the research sequence of this paper
Related Papers
2004
被引用51 | 浏览
2013
被引用31 | 浏览
2017
被引用7 | 浏览
2021
被引用5 | 浏览
2019
被引用21 | 浏览
Data Disclaimer
The page data are from open Internet sources, cooperative publishers and automatic analysis results through AI technology. We do not make any commitments and guarantees for the validity, accuracy, correctness, reliability, completeness and timeliness of the page data. If you have any questions, please contact us by email: report@aminer.cn
Chat Paper