Cross-Dimension Attentive Feature Fusion Network for Unsupervised Time-Series Anomaly Detection
CMES-COMPUTER MODELING IN ENGINEERING & SCIENCES(2024)
摘要
Time series anomaly detection is crucial in various industrial applications to identify unusual behaviors within the time series data. Due to the challenges associated with annotating anomaly events, time series reconstruction has become a prevalent approach for unsupervised anomaly detection. However, effectively learning representations and achieving accurate detection results remain challenging due to the intricate temporal patterns and dependencies in real -world time series. In this paper, we propose a cross -dimension attentive feature fusion network for time series anomaly detection, referred to as CAFFN. Specifically, a series and feature mixing block is introduced to learn representations in 1D space. Additionally, a fast Fourier transform is employed to convert the time series into 2D space, providing the capability for 2D feature extraction. Finally, a cross -dimension attentive feature fusion mechanism is designed that adaptively integrates features across different dimensions for anomaly detection. Experimental results on real -world time series datasets demonstrate that CAFFN performs better than other competing methods in time series anomaly detection.
更多查看译文
关键词
Time series anomaly detection,unsupervised feature learning,feature fusion
AI 理解论文
溯源树
样例
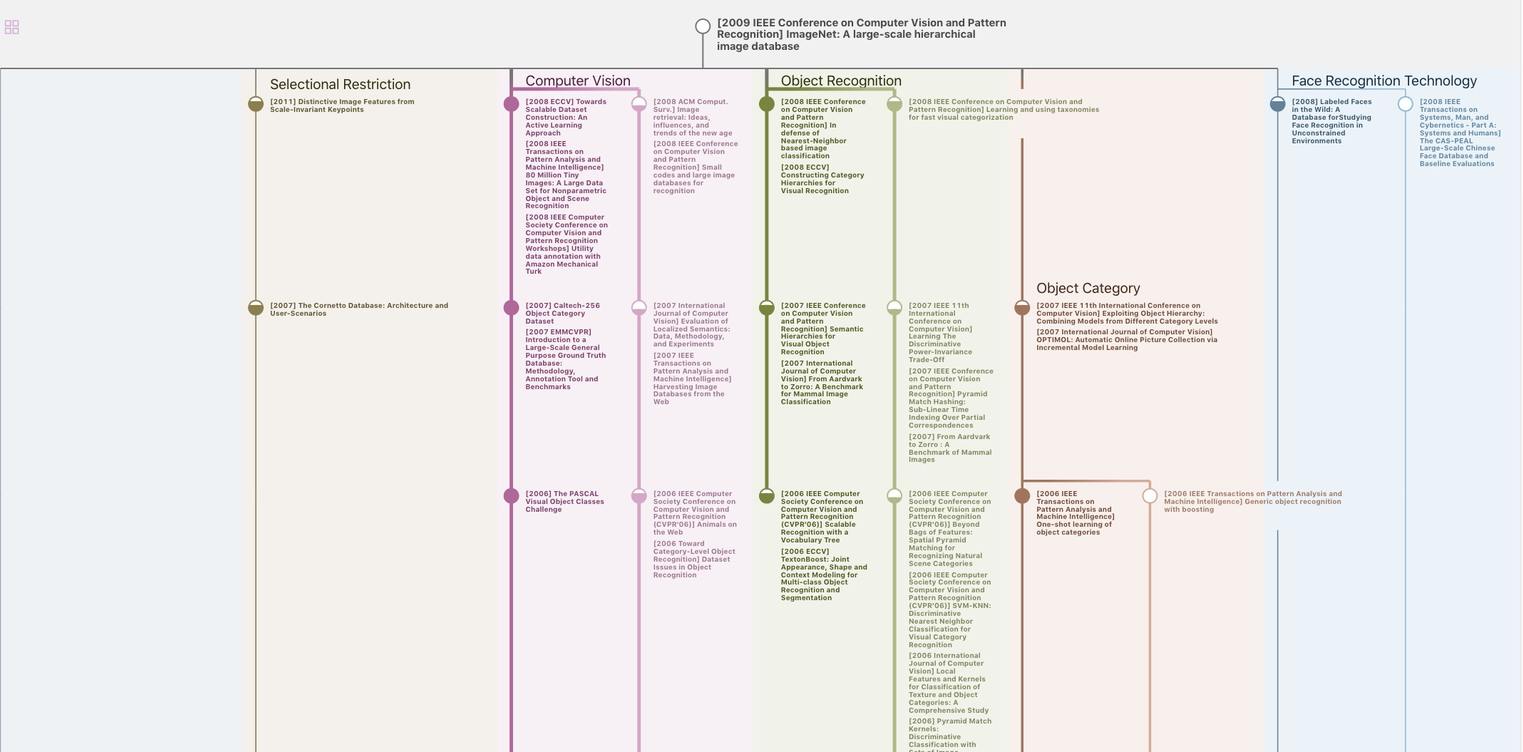
生成溯源树,研究论文发展脉络
Chat Paper
正在生成论文摘要