Transformer-based dual path cross fusion for pansharpening remote sensing images
INTERNATIONAL JOURNAL OF REMOTE SENSING(2024)
摘要
The purpose of pan-sharpening is to generate high-resolution multispectral (HRMS) images by combining multispectral images (MS) and panchromatic images (PAN), which have become an important part of remote sensing image processing. Therefore, how to better extract complete feature information from MS images and PAN images has become the focus of our attention. In this paper, we propose a new pansharpening method, called Transformer-based Dual-path cross fusion network (TDF) for Pan-sharpening remote sensing images, which aims to extract the spatial details of PAN images while maintaining the spectral fidelity of MS images. The whole network structure can be divided into two parts: in the encoder part, we adopt the Swin-Transformer module for the downsampling operation, which expands the sensory field of the network to the feature map, and then extracts the global information. However, since the Swin-Transformer module is not good at extracting pixel-level details, we introduce the Base Feature Extraction (BFE) and the Invertible Neural Network Block (INNB) modules for the interaction between the local and the global feature information. we also introduce the Edge-Enhancement Block (EEB) to further enhance the feature extraction at multi-scales during the image fusion process. In the decoder section, we once again employ the Swin Transformer module for downsampling. After the convolution operation and activation function, we utilize the Sub-Pixel Convolutional Neural Network for upsampling to generate the ultimate high-resolution multispectral images. Simulation experiments and real experiments are conducted on QuickBird (QB) and WorldView2 (WV2) datasets, which demonstrated our method are superior to the current methods.
更多查看译文
关键词
Pansharpening,convolutional neural network,transformer,multispectral image,panchromatic image
AI 理解论文
溯源树
样例
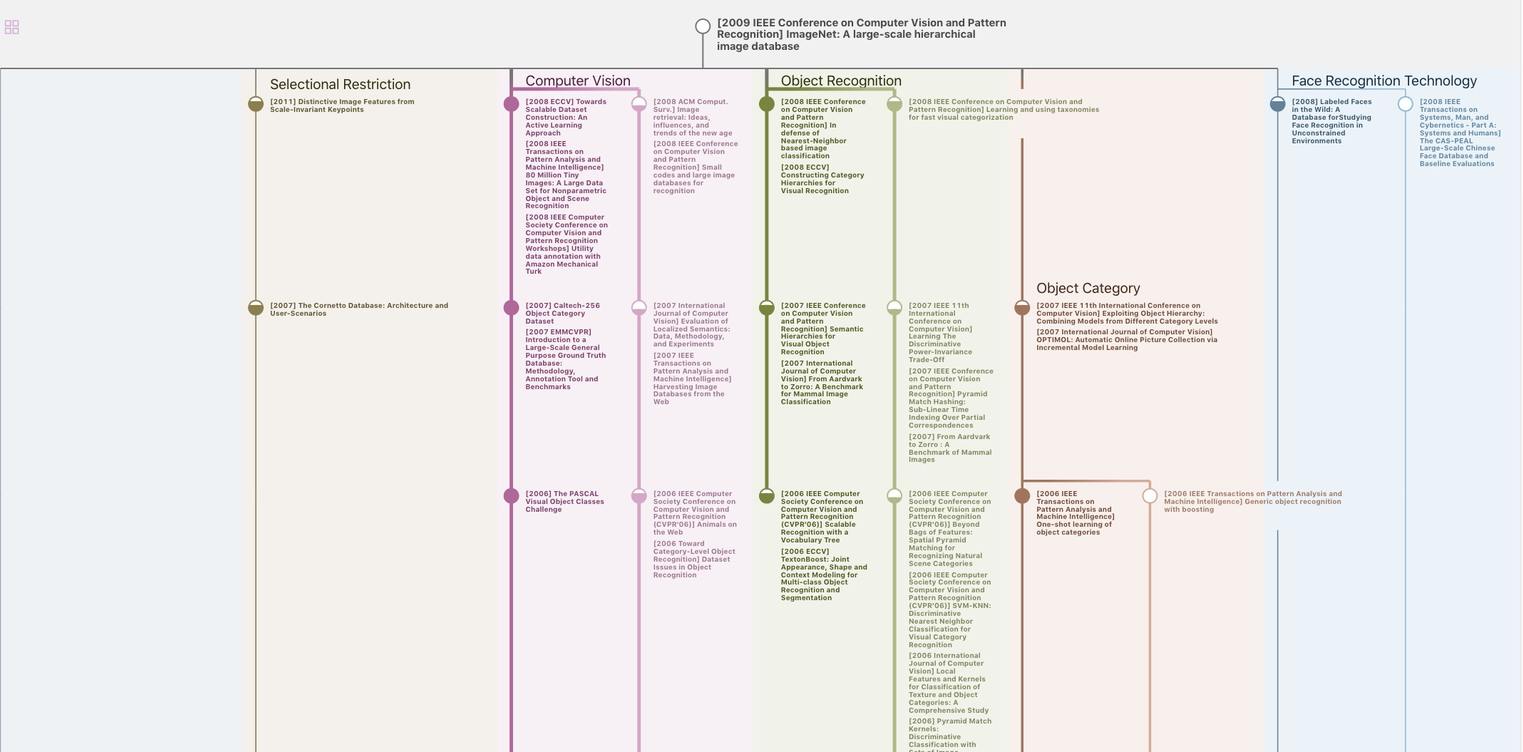
生成溯源树,研究论文发展脉络
Chat Paper
正在生成论文摘要