Multi-fidelity reduced-order surrogate modelling
PROCEEDINGS OF THE ROYAL SOCIETY A-MATHEMATICAL PHYSICAL AND ENGINEERING SCIENCES(2024)
摘要
High-fidelity numerical simulations of partial differential equations (PDEs) given a restricted computational budget can significantly limit the number of parameter configurations considered and/or time window evaluated. Multi-fidelity surrogate modelling aims to leverage less accurate, lower-fidelity models that are computationally inexpensive in order to enhance predictive accuracy when high-fidelity data are scarce. However, low-fidelity models, while often displaying the qualitative solution behaviour, fail to accurately capture fine spatio-temporal and dynamic features of high-fidelity models. To address this shortcoming, we present a data-driven strategy that combines dimensionality reduction with multi-fidelity neural network surrogates. The key idea is to generate a spatial basis by applying proper orthogonal decomposition (POD) to high-fidelity solution snapshots, and approximate the dynamics of the reduced states-time-parameter-dependent expansion coefficients of the POD basis-using a multi-fidelity long short-term memory network. By mapping low-fidelity reduced states to their high-fidelity counterpart, the proposed reduced-order surrogate model enables the efficient recovery of full solution fields over time and parameter variations in a non-intrusive manner. The generality of this method is demonstrated by a collection of PDE problems where the low-fidelity model can be defined by coarser meshes and/or time stepping, as well as by misspecified physical features.
更多查看译文
关键词
reduced-order modelling,multi-fidelity surrogate modelling,LSTM networks,proper orthogonal decomposition,parametrized PDEs
AI 理解论文
溯源树
样例
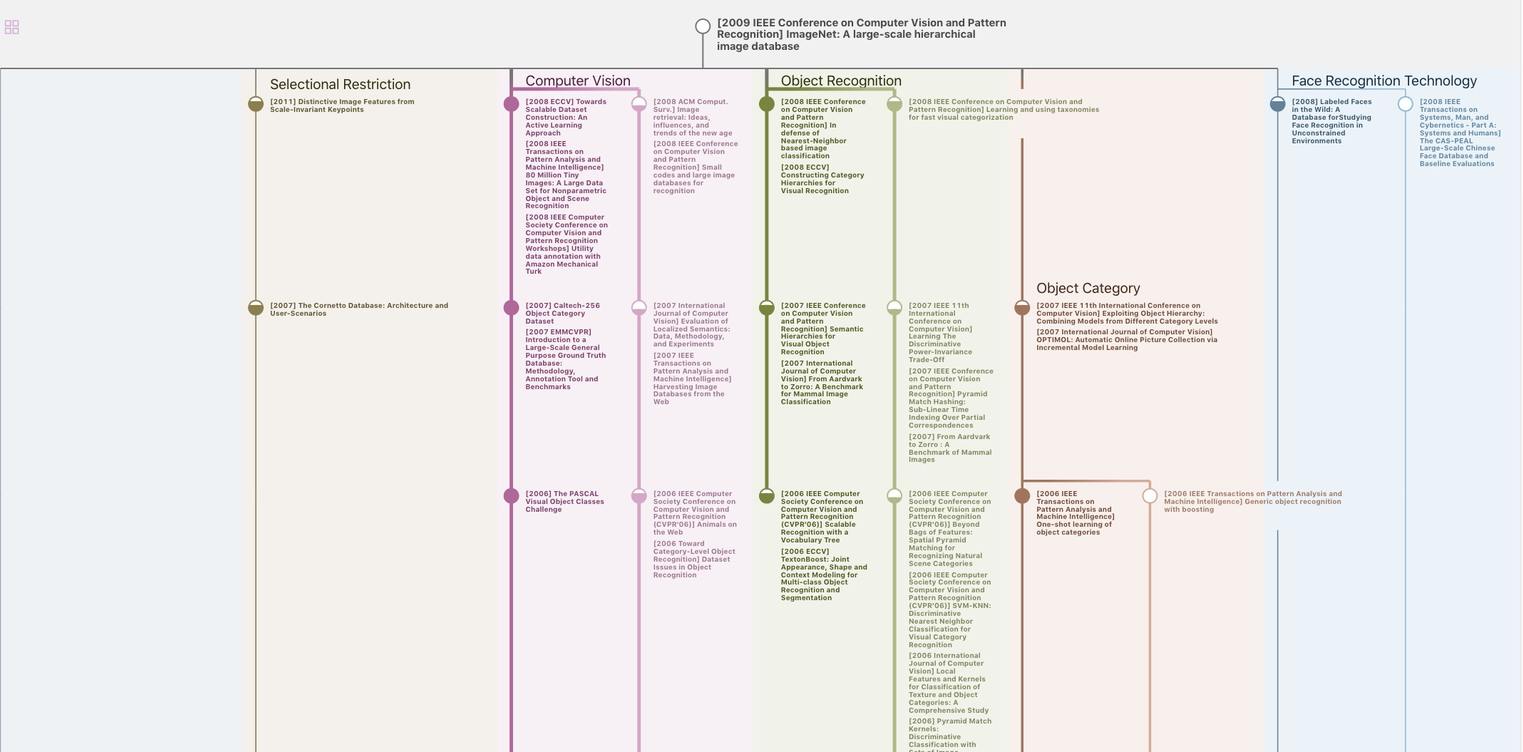
生成溯源树,研究论文发展脉络
Chat Paper
正在生成论文摘要