Generalizations of the Jaccard index and S?rensen index for assessing agreement across multiple readers in object detection and instance segmentation in biomedical imaging
JOURNAL OF MEDICAL IMAGING(2023)
摘要
Significance Manual annotations are necessary for training supervised learning algorithms for object detection and instance segmentation. These manual annotations are difficult to acquire, noisy, and inconsistent across readers.Aim The goal of this work is to describe and demonstrate multireader generalizations of the Jaccard and Sorensen indices for object detection and instance segmentation.Approach The multireader Jaccard and Sorensen indices are described in terms of "calls," "objects," and number of readers. These generalizations reduce to the equations defined by confusion matrix variables in the two-reader case. In a test set of 50 cell microscopy images, we use these generalizations to assess reader variability and compare the performance of an object detection network (Yolov5) and an instance segmentation algorithm (Cellpose2.0) with a group of five human readers using the Mann-Whitney U-test with Bonferroni correction for multiplicity.Results The multireader generalizations were statistically different from the mean of pairwise comparisons of readers (p < 0.0001). Further, these multireader generalizations informed when a reader was performing differently than the group. Finally, these generalizations show that Yolov5 and Cellpose2.0 performed similarly to the pool of human readers. The lower bound of the one-sided 90% confidence interval for the difference in the multireader Jaccard index between the pool of human readers and the pool of human readers plus an algorithm were -0.019 and -0.016 for Yolov5 and Cellpose2.0, respectively.Conclusions Multireader generalizations of the Jaccard and Sorensen indices provide metrics for characterizing the agreement of an arbitrary number of readers on object detection and instance segmentation tasks.
更多查看译文
关键词
reader performance,reader agreement,algorithm performance,supervised learning,biomedical imaging
AI 理解论文
溯源树
样例
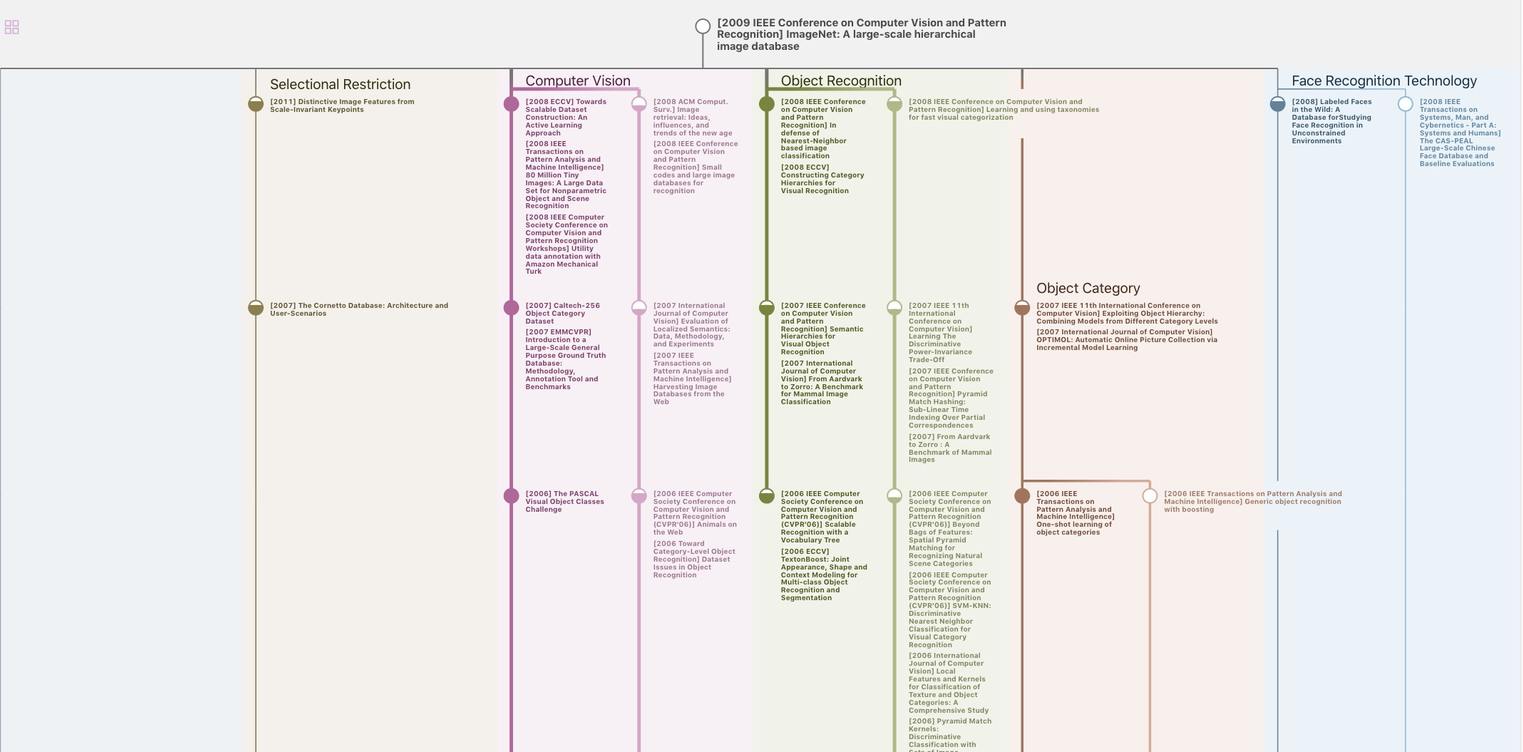
生成溯源树,研究论文发展脉络
Chat Paper
正在生成论文摘要