Lignin-Based Polymer Networks Enabled N, S Co-Doped Defect-Rich Hierarchically Porous Carbon Anode for Long-Cycle Li-Ion Batteries
ACS sustainable chemistry & engineering(2024)SCI 2区
Dalian Polytech Univ | Zhejiang Normal Univ
Abstract
The commercial graphitic anode with low specific capacity and serious lithium dendrite growth has limited further improvements of Li-ion batteries (LIBs). Here, we report a hierarchical porous hard carbon with N, S codoping (LSCF) that is synthesized using trifunctional sodium lignosulfonate (LS) from the pulp waste as the self-sacrificing template, carbon source, sulfur source, and natural chitosan as both the carbon source and nitrogen source. The strong hydrogen-bond interaction between the LS and chitosan enabled the robust stability of the carbon framework. And the self-template of sulfates and carbonates from LS enabled the formation of the hierarchical porous structure. First-principles calculations indicate that the hierarchical porous LSCF carbon with heteroatom doping has a lower diffusion barrier of Li+ and a higher electron conductivity. As an anode for LIBs, the LSCF delivers the specific capacity of 350 mAh g(-1) at 100 mA g(-1) with 85% retention after 1000 cycles. This work offers a one-step and low-cost method to fabricate the porous hard carbon anode for LIBs with dual-atom doping based on green natural polymers.
MoreTranslated text
Key words
lignin,chitosan,doping,hard carbonanode,lithium-ion battery
求助PDF
上传PDF
View via Publisher
AI Read Science
AI Summary
AI Summary is the key point extracted automatically understanding the full text of the paper, including the background, methods, results, conclusions, icons and other key content, so that you can get the outline of the paper at a glance.
Example
Background
Key content
Introduction
Methods
Results
Related work
Fund
Key content
- Pretraining has recently greatly promoted the development of natural language processing (NLP)
- We show that M6 outperforms the baselines in multimodal downstream tasks, and the large M6 with 10 parameters can reach a better performance
- We propose a method called M6 that is able to process information of multiple modalities and perform both single-modal and cross-modal understanding and generation
- The model is scaled to large model with 10 billion parameters with sophisticated deployment, and the 10 -parameter M6-large is the largest pretrained model in Chinese
- Experimental results show that our proposed M6 outperforms the baseline in a number of downstream tasks concerning both single modality and multiple modalities We will continue the pretraining of extremely large models by increasing data to explore the limit of its performance
Upload PDF to Generate Summary
Must-Reading Tree
Example
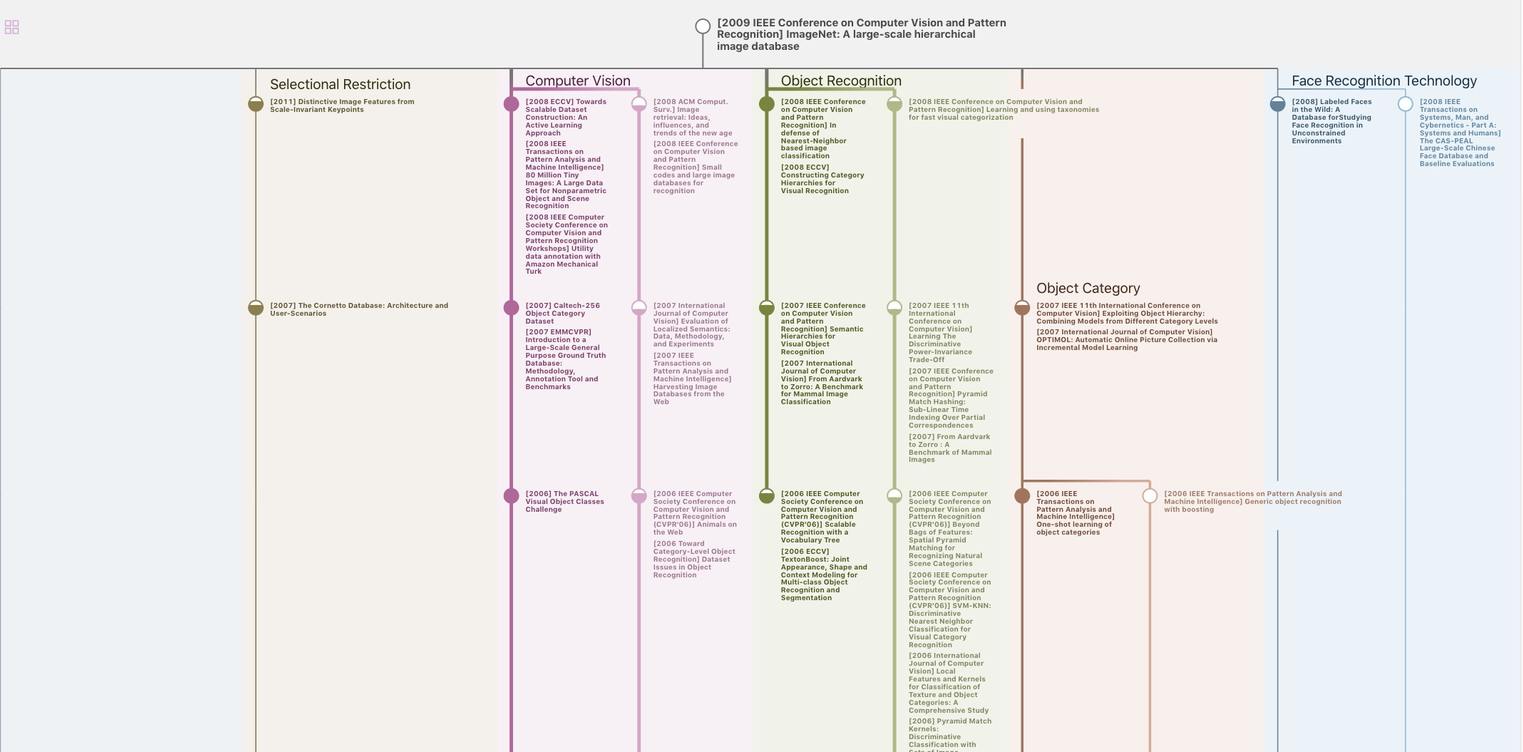
Generate MRT to find the research sequence of this paper
Related Papers
2015
被引用566 | 浏览
2016
被引用48 | 浏览
2018
被引用188 | 浏览
2017
被引用17 | 浏览
2018
被引用195 | 浏览
2018
被引用82 | 浏览
2019
被引用39 | 浏览
2020
被引用8 | 浏览
2022
被引用40 | 浏览
2022
被引用15 | 浏览
2022
被引用66 | 浏览
2022
被引用15 | 浏览
2022
被引用15 | 浏览
2022
被引用35 | 浏览
2023
被引用18 | 浏览
2014
被引用52 | 浏览
2023
被引用29 | 浏览
Data Disclaimer
The page data are from open Internet sources, cooperative publishers and automatic analysis results through AI technology. We do not make any commitments and guarantees for the validity, accuracy, correctness, reliability, completeness and timeliness of the page data. If you have any questions, please contact us by email: report@aminer.cn
Chat Paper