FSKT-GE: Feature maps similarity knowledge transfer for low-resolution gaze estimation
IET IMAGE PROCESSING(2024)
摘要
The limited of texture details information in low-resolution facial or eye images presents a challenge for gaze estimation. To address this, FSKT-GE (feature maps similarity knowledge transfer for low-resolution gaze estimation) is proposed, a gaze estimation framework consisting of both a high resolution (HR) network and low resolution (LR) network with the identical structure. Rather than mere feature imitation, this issue is addressed by assessing the cosine similarity of feature layers, emphasizing the distribution similarity between the HR and LR networks. This enables the LR network to acquire richer knowledge. This framework utilizes a combination loss function, incorporating cosine similarity measurement, soft loss based on probability distribution difference and gaze direction output, along with a hard loss from the LR network output layer. This approach on low-resolution datasets derived from Gaze360 and RT-Gene datasets is validated, demonstrating excellent performance in low-resolution gaze estimation. Evaluations on low-resolution images obtained through 2x, 4x, and 8x down-sampling are conducted on two datasets. On the Gaze360 dataset, the lowest mean angular errors of 10.97 degrees, 11.22 degrees, and 13.61 degrees were achieved, while on the RT-Gene dataset, the lowest mean angular errors of 6.73 degrees, 6.83 degrees, and 7.75 degrees were obtained. Here, a novel approach called feature map similarity-based knowledge transfer for low-resolution gaze estimation (FSKT-GE) is proposed. The motivation behind this work is to address the challenge of accurately estimating gaze direction for low-resolution facial images encountered in unconstrained outdoor environments. image
更多查看译文
关键词
computer graphics,computer vision,convolutional neural nets
AI 理解论文
溯源树
样例
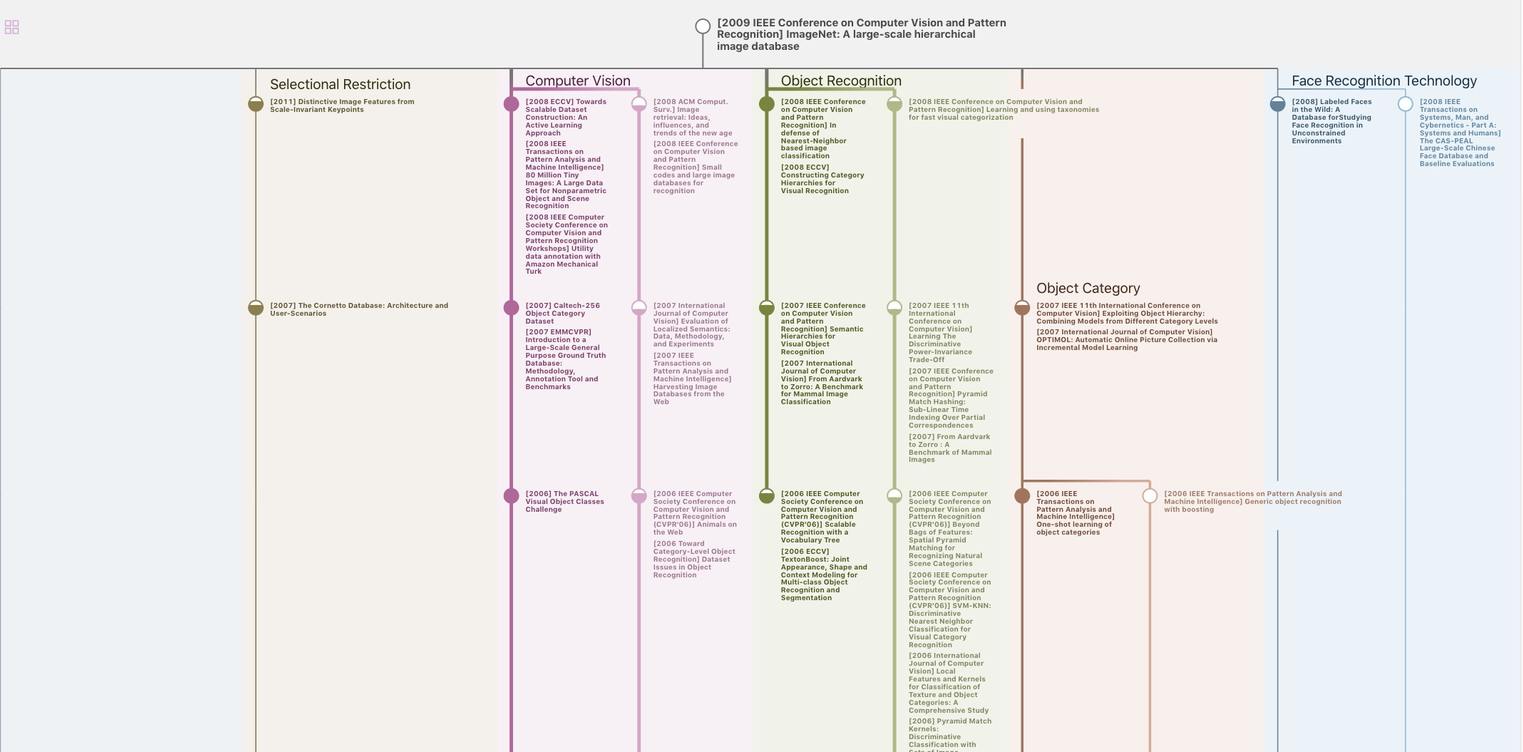
生成溯源树,研究论文发展脉络
Chat Paper
正在生成论文摘要