Data-Driven Superstabilizing Control under Quadratically-Bounded Errors-in-Variables Noise
arxiv(2024)
摘要
The Error-in-Variables model of system identification/control involves
nontrivial input and measurement corruption of observed data, resulting in
generically nonconvex optimization problems. This paper performs
full-state-feedback stabilizing control of all discrete-time linear systems
that are consistent with observed data for which the input and measurement
noise obey quadratic bounds. Instances of such quadratic bounds include
elementwise norm bounds (at each time sample), energy bounds (across the entire
signal), and chance constraints arising from (sub)gaussian noise.
Superstabilizing controllers are generated through the solution of a
sum-of-squares hierarchy of semidefinite programs. A theorem of alternatives is
employed to eliminate the input and measurement noise process, thus improving
tractability. Effectiveness of the scheme is generated on an example system in
the chance-constrained set-membership setting where the input and
state-measurement noise are i.i.d. normally distributed.
更多查看译文
AI 理解论文
溯源树
样例
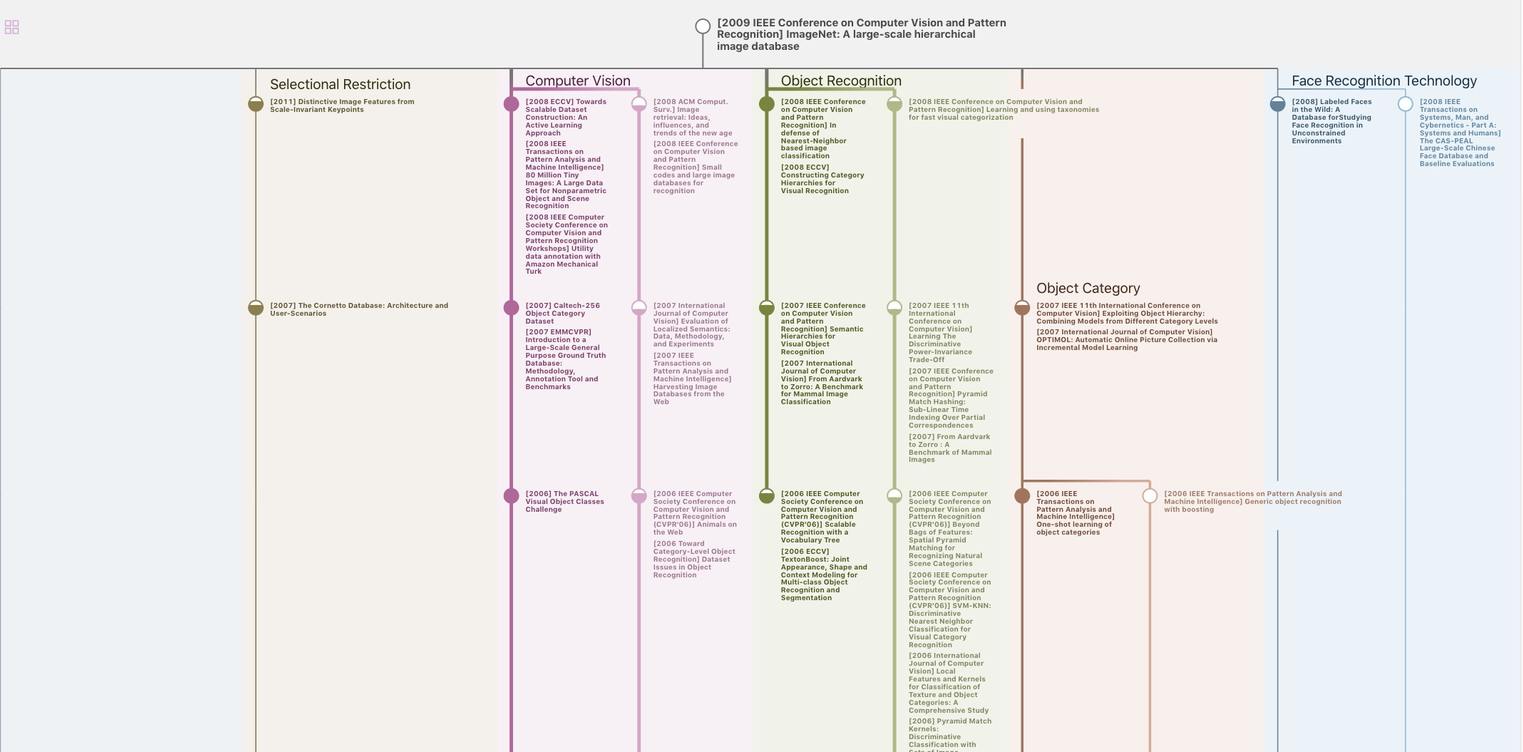
生成溯源树,研究论文发展脉络
Chat Paper
正在生成论文摘要