Multi-Task Learning for Real-Time Autonomous Driving Leveraging Task-Adaptive Attention Generator
ICRA 2024(2024)
摘要
Real-time processing is crucial in autonomous driving systems due to the imperative of instantaneous decision-making and rapid response. In real-world scenarios, autonomous vehicles are continuously tasked with interpreting their surroundings, analyzing intricate sensor data, and making decisions within split seconds to ensure safety through numerous computer vision tasks. In this paper, we present a new real-time multi-task network adept at three vital autonomous driving tasks: monocular 3D object detection, semantic segmentation, and dense depth estimation. To counter the challenge of negative transfer — the prevalent issue in multi-task learning — we introduce a task-adaptive attention generator. This generator is designed to automatically discern interrelations across the three tasks and arrange the task-sharing pattern, all while leveraging the efficiency of the hard-parameter sharing approach. To the best of our knowledge, the proposed model is pioneering in its capability to concurrently handle multiple tasks, notably 3D object detection, while maintaining real-time processing speeds. Our rigorously optimized network, when tested on the Cityscapes-3D datasets, consistently outperforms various baseline models. Moreover, an in-depth ablation study substantiates the efficacy of the methodologies integrated into our framework.
更多查看译文
关键词
Deep Learning for Visual Perception,Computer Vision for Transportation,Vision-Based Navigation
AI 理解论文
溯源树
样例
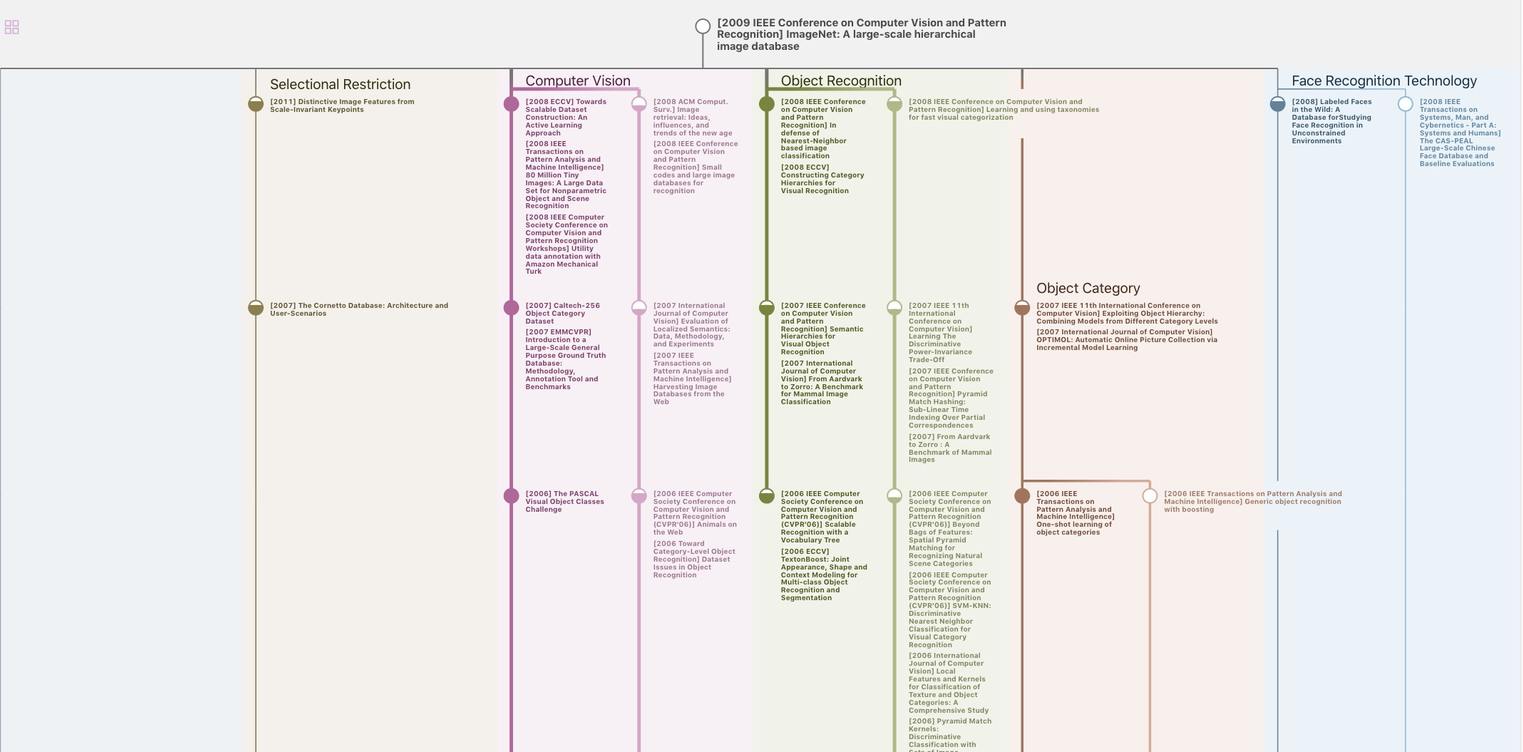
生成溯源树,研究论文发展脉络
Chat Paper
正在生成论文摘要