Robust MITL Planning under Uncertain Navigation Times
ICRA 2024(2024)
Key words
Formal Methods in Robotics and Automation,Planning under Uncertainty
AI Read Science
Must-Reading Tree
Example
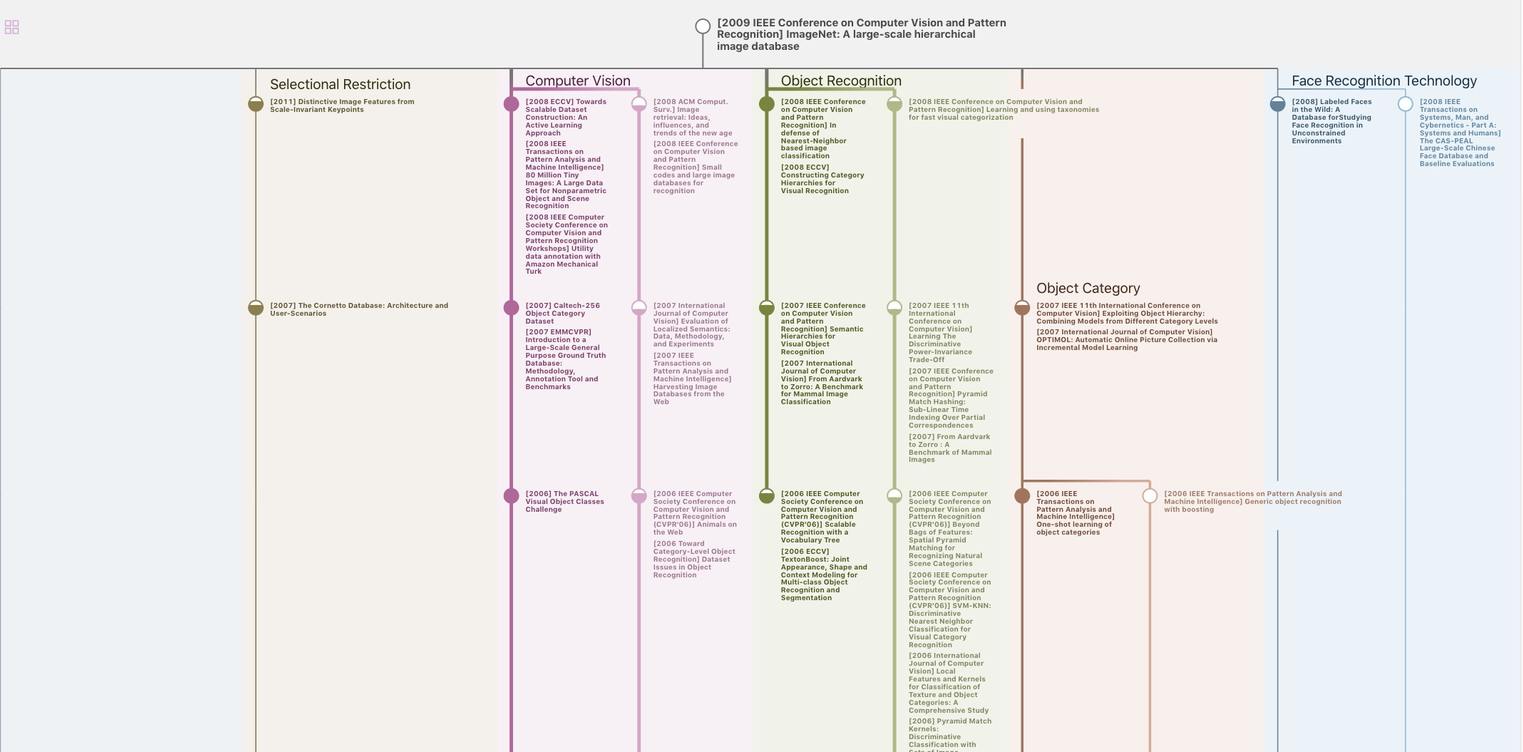
Generate MRT to find the research sequence of this paper
Chat Paper
Summary is being generated by the instructions you defined