Popeye: A Unified Visual-Language Model for Multi-Source Ship Detection from Remote Sensing Imagery
arxiv(2024)
摘要
Ship detection needs to identify ship locations from remote sensing (RS)
scenes. However, due to different imaging payloads, various appearances of
ships, and complicated background interference from the bird's eye view, it is
difficult to set up a unified paradigm for achieving multi-source ship
detection. Therefore, in this article, considering that the large language
models (LLMs) emerge the powerful generalization ability, a novel unified
visual-language model called Popeye is proposed for multi-source ship detection
from RS imagery. First, to bridge the interpretation gap between multi-source
images for ship detection, a novel image-instruction-answer way is designed to
integrate the various ship detection ways (e.g., horizontal bounding box (HBB),
oriented bounding box (OBB)) into a unified labeling paradigm. Then, in view of
this, a cross-modal image interpretation method is developed for the proposed
Popeye to enhance interactive comprehension ability between visual and language
content, which can be easily migrated into any multi-source ship detection
task. Subsequently, owing to objective domain differences, a knowledge adaption
mechanism is designed to adapt the pre-trained visual-language knowledge from
the nature scene into the RS domain for multi-source ship detection. In
addition, the segment anything model (SAM) is also seamlessly integrated into
the proposed Popeye to achieve pixel-level ship segmentation without additional
training costs. Finally, extensive experiments are conducted on the newly
constructed instruction dataset named MMShip, and the results indicate that the
proposed Popeye outperforms current specialist, open-vocabulary, and other
visual-language models for zero-shot multi-source ship detection.
更多查看译文
AI 理解论文
溯源树
样例
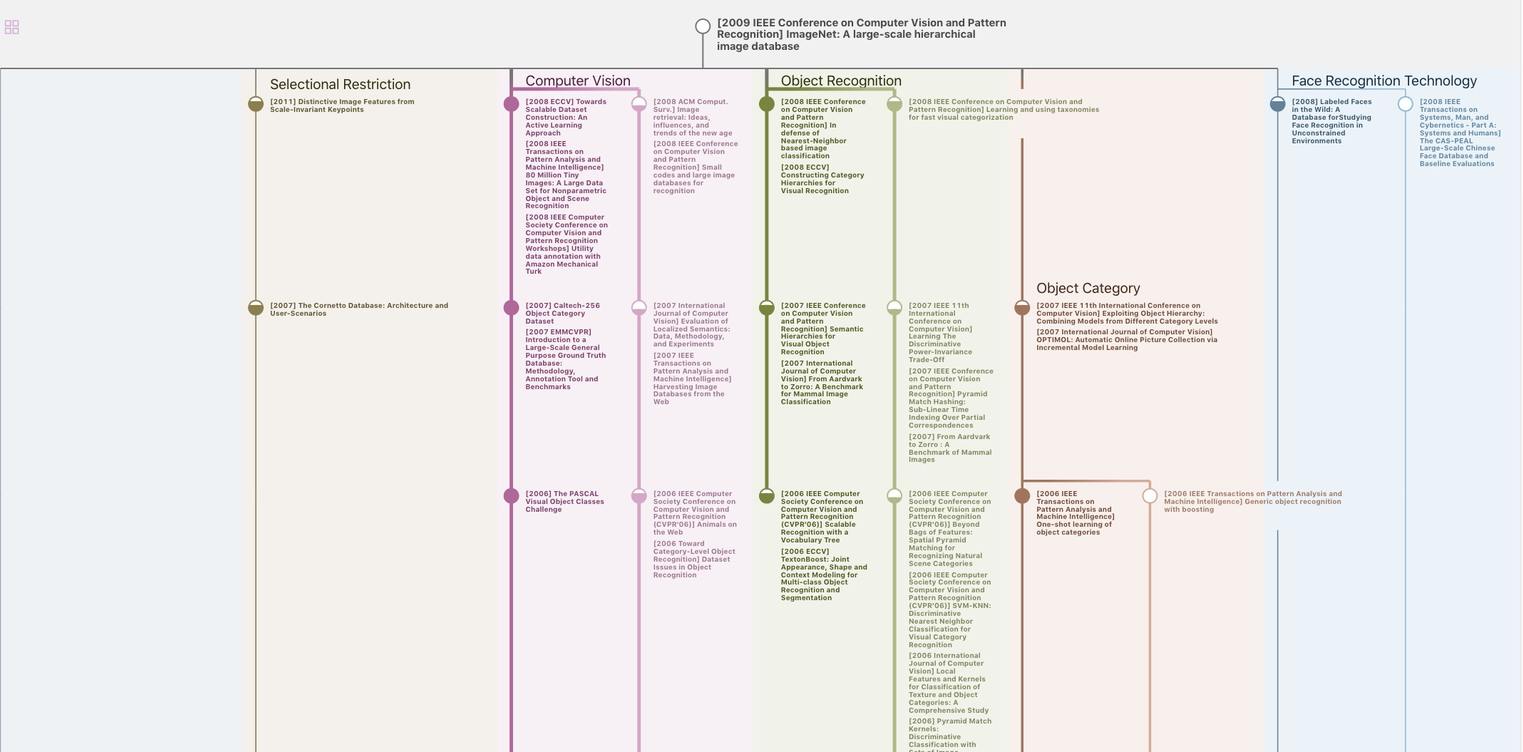
生成溯源树,研究论文发展脉络
Chat Paper
正在生成论文摘要