Joint multi-task learning improves weakly-supervised biomarker prediction in computational pathology
CoRR(2024)
摘要
Deep Learning (DL) can predict biomarkers directly from digitized cancer
histology in a weakly-supervised setting. Recently, the prediction of
continuous biomarkers through regression-based DL has seen an increasing
interest. Nonetheless, clinical decision making often requires a categorical
outcome. Consequently, we developed a weakly-supervised joint multi-task
Transformer architecture which has been trained and evaluated on four public
patient cohorts for the prediction of two key predictive biomarkers,
microsatellite instability (MSI) and homologous recombination deficiency (HRD),
trained with auxiliary regression tasks related to the tumor microenvironment.
Moreover, we perform a comprehensive benchmark of 16 approaches of task
balancing for weakly-supervised joint multi-task learning in computational
pathology. Using our novel approach, we improve over the state-of-the-art area
under the receiver operating characteristic by +7.7
yielding better clustering of latent embeddings by +8
prediction of MSI and HRD in external cohorts, respectively.
更多查看译文
AI 理解论文
溯源树
样例
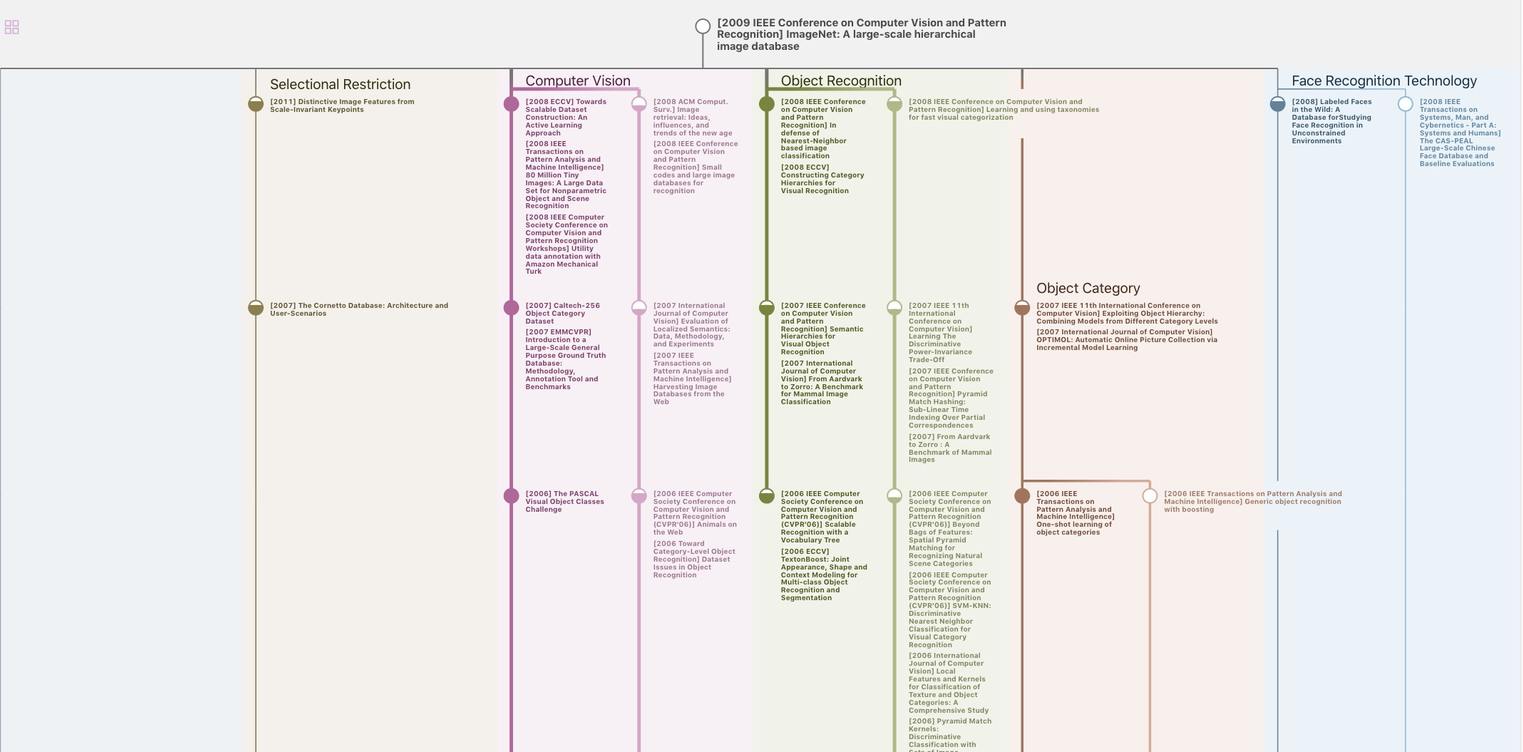
生成溯源树,研究论文发展脉络
Chat Paper
正在生成论文摘要