Elastic constant estimation for low-Q materials using convolutional neural network based resonant ultrasound spectroscopy
NONDESTRUCTIVE TESTING AND EVALUATION(2024)
摘要
Resonant ultrasound spectroscopy (RUS) is a non-destructive technique to calculate material elastic constants from resonant frequencies. However, low quality factor (i.e., low-Q) materials experience strong energy attenuation during vibration, resulting in the overlap and partial loss of resonant peaks in experimental spectra. Consequently, extracting authentic resonant frequencies becomes challenging, making the subsequent elastic constant calculation time-intensive and necessitating expert intervention. Regarding this, a novel RUS method using convolutional neural networks (CNN) is proposed. This method circumvents the traditional frequency extraction step and enables rapid and automated estimation of elastic constants for low-Q materials directly from the experimental spectra. The CNN model was designed to take real parts of the resonant spectra and mass density as inputs, outputting the material's independent elastic constants. Leveraging a simulation dataset for training, the model's feasibility was demonstrated by characterizing five elastic constants of a composite material with a Q of approximately 25. The trained model performed well on both the simulated test set and three real-world samples, achieving a sample proportion of 96.5% in the test set with all relative errors of elastic constants falling within 10%. This study contributes a fresh perspective to efficiently estimating elastic constants in RUS, particularly for low-Q materials.
更多查看译文
关键词
Resonant ultrasound spectroscopy,elastic constant,quality factor,convolutional neural network
AI 理解论文
溯源树
样例
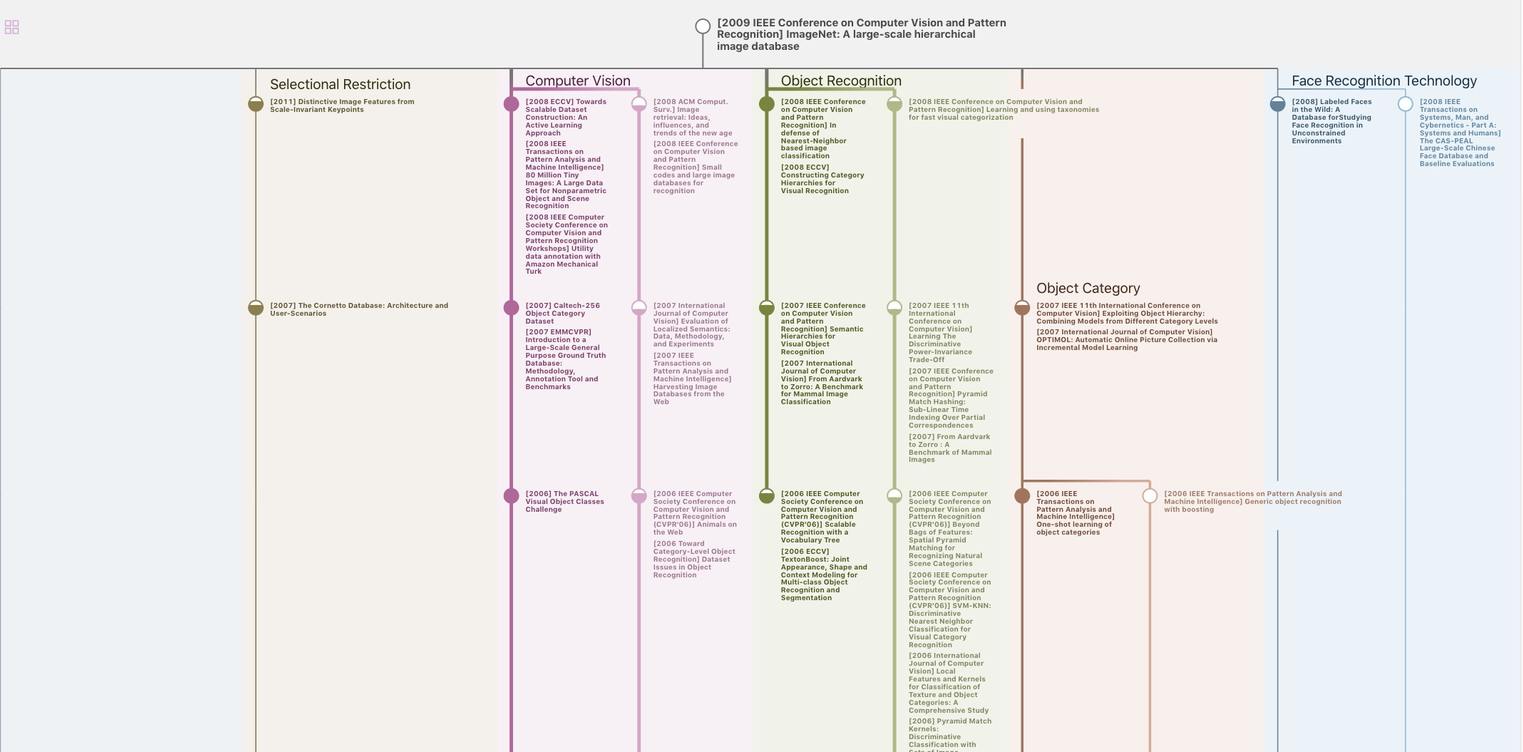
生成溯源树,研究论文发展脉络
Chat Paper
正在生成论文摘要