A Novel Approach for Intelligent Diagnosis and Grading of Diabetic Retinopathy
Computers in Biology and Medicine(2024)
摘要
Diabetic retinopathy (DR) is a severe ocular complication of diabetes that can lead to vision damage and even blindness. Currently, traditional deep convolutional neural networks (CNNs) used for DR grading tasks face two primary challenges: (1) insensitivity to minority classes due to imbalanced data distribution, and (2) neglecting the relationship between the left and right eyes by utilizing the fundus image of only one eye for training without differentiating between them. To tackle these challenges, we proposed the DRGCNN (DR Grading CNN) model. To solve the problem caused by imbalanced data distribution, our model adopts a more balanced strategy by allocating an equal number of channels to feature maps representing various DR categories. Furthermore, we introduce a CAM-EfficientNetV2-M encoder dedicated to encoding input retinal fundus images for feature vector generation. The number of parameters of our encoder is 52.88 M, which is less than RegNet_y_16gf (80.57 M) and EfficientNetB7 (63.79 M), but the corresponding kappa value is higher. Additionally, in order to take advantage of the binocular relationship, we input fundus retinal images from both eyes of the patient into the network for features fusion during the training phase. We achieved a kappa value of 86.62% on the EyePACS dataset and 86.16% on the Messidor-2 dataset. Experimental results on these representative datasets for diabetic retinopathy (DR) demonstrate the exceptional performance of our DRGCNN model, establishing it as a highly competitive intelligent classification model in the field of DR. The code is available for use at https://github.com/Fat-Hai/DRGCNN.
更多查看译文
关键词
Diabetic retinopathy grading,Deep learning,CAM-EfficientNetV2-M,Category attention module (CAM),Binocular features fusion network (BFFN)
AI 理解论文
溯源树
样例
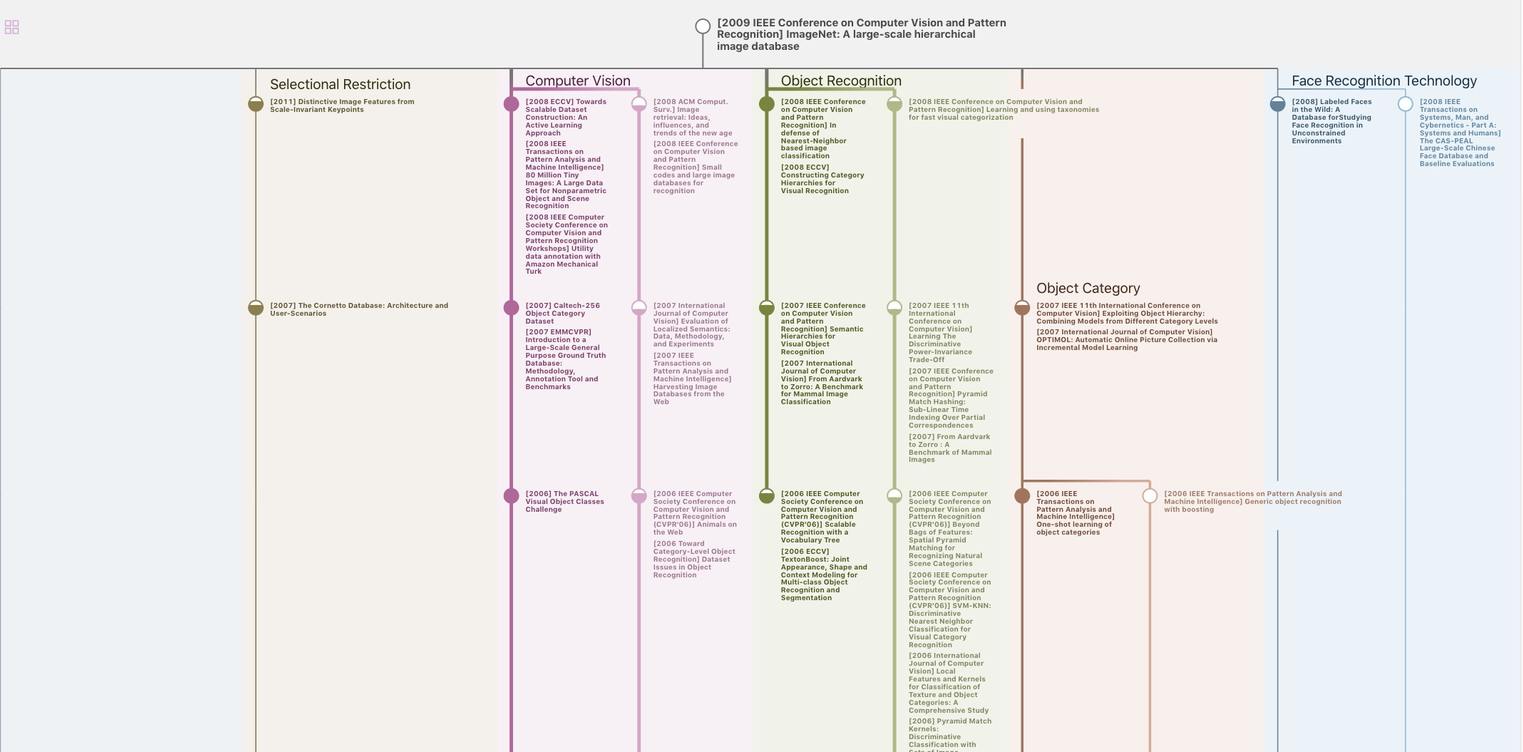
生成溯源树,研究论文发展脉络
Chat Paper
正在生成论文摘要