3D Seismic Fault Detection Via Contrastive-Reconstruction Representation Learning
Expert systems with applications(2024)
摘要
Fault detection is a critical step in structural modeling and characterizing reservoirs. 3D seismic fault labeling is almost impossible to obtain, while networks trained on synthetic fault data have limited generalization to real data. We use self-supervised representation learning to enable networks to learn seismic field data features during pre-training, improving generalization. However, widely popular ViT/SwinViT-based methods cannot capture low-level fault features well. CNN-based have limited capability in transferring to downstream tasks. Tackle this, we designed the Tiny Self-Attention and extensively embedded it into the HRNet. It merges the advantages of convolutional and self-attention, allowing for in-depth learning of seismic representation information during the representation learning phase, thus achieving better inter-class separation in downstream tasks. We crafted two proxy tasks. The contrastive task aims to minimize the projected feature distance of overlapping areas from two distinct views. To tackle the issue of memory overflow and training suspension caused by the high spatial and temporal complexity of 3D high-resolution feature matching, we introduced sparse distance matching and an adaptive feature aggregation. In reconstruction task, we designed a masking strategy tailored to the unique attributes of 3D seismic data. It process named ”Fault Detection via Contrast-Reconstruction Representation Learning” (FaultCRL). Experimentally, we compared the latest fault detection methods, and representation learning techniques like MAE and SwinUNETR, showcasing the notable advantage of FaultCRL in fault detection tasks. The appendix provides all qualitative results from mainstream geophysical journals regarding The Netherlands-F3 data in recent years, indicating that our approach has reached the current state-of-the-art level.
更多查看译文
关键词
Seismic data,Seismic fault,Image segmentation,Pretrained Foundation Models (PFMs),Contrastive learning,Representation learning,Self-supervised learning
AI 理解论文
溯源树
样例
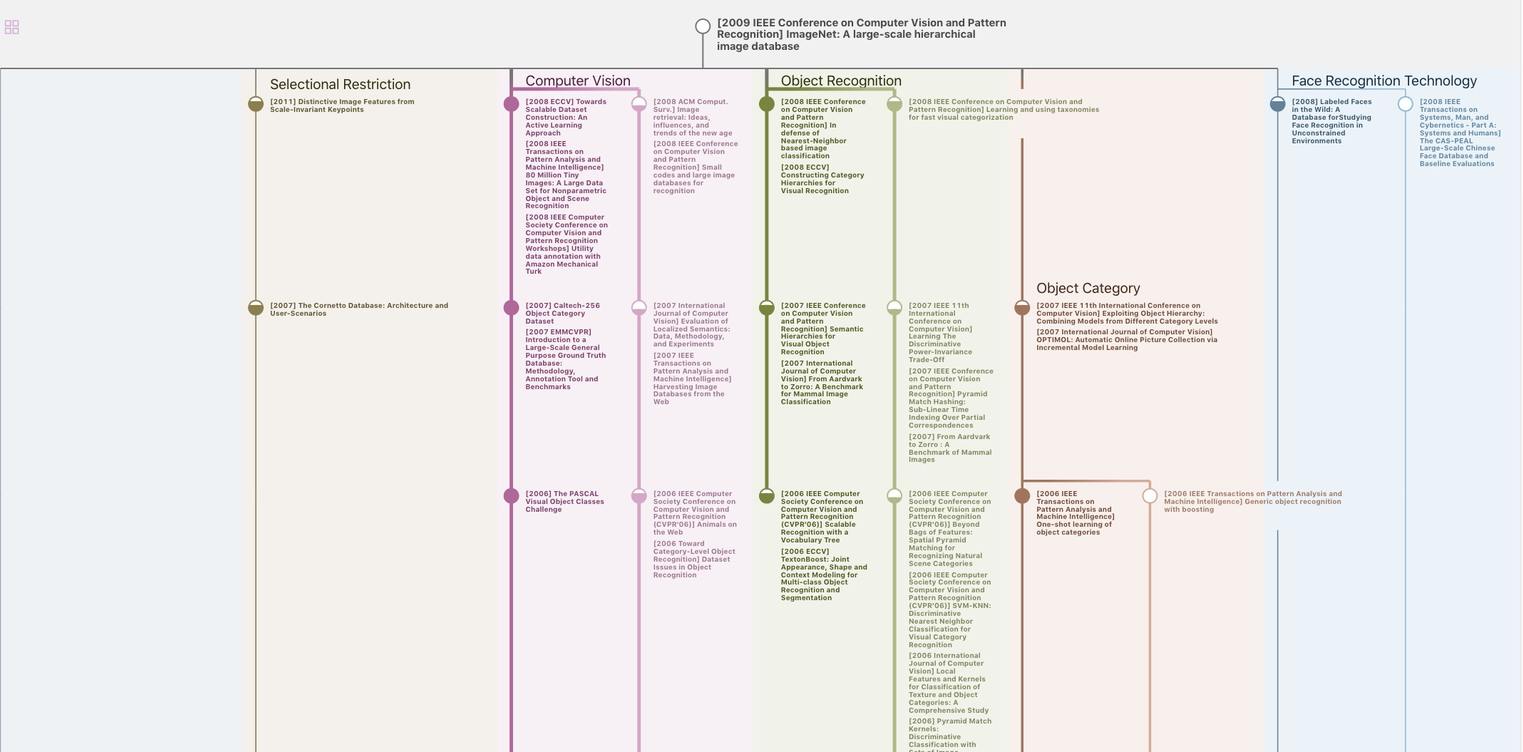
生成溯源树,研究论文发展脉络
Chat Paper
正在生成论文摘要