Independent Dual Graph Attention Convolutional Network for skeleton-based action recognition
Neurocomputing(2024)
摘要
Graph convolutional networks (GCNs) have been widely adopted in skeleton-based action recognition, achieving impressive outcomes. However, the convolution operations in GCNs fail to make full use of the original input data, which restricts its ability to accurately capture the correlation within the skeleton. To solve this issue, this study introduces an independent dual graph attention convolutional network (IDGAN). Specifically, IDGAN additionally incorporates an instinctive attention module that leverages self-attention to capture the correlation among the joints in the original input skeleton. In addition, two independent convolutional operations are used to process two self-attention modules, respectively, to further refine the relationship between skeleton joints. Extensive experiments on several publicly available datasets show that IDGAN outperforms most state-of-the-art algorithms.
更多查看译文
关键词
Action recognition,Graph convolutional networks,Independent dual attention
AI 理解论文
溯源树
样例
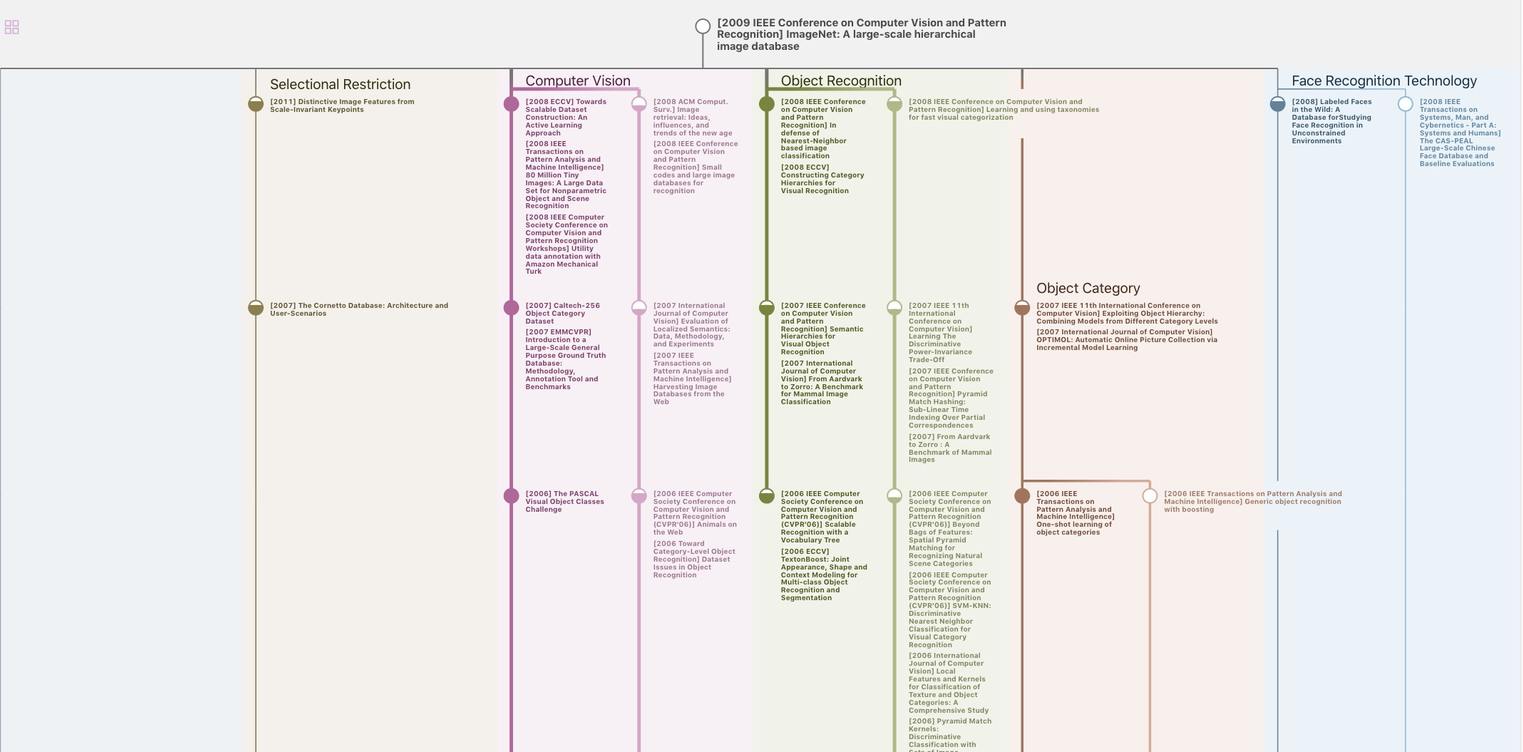
生成溯源树,研究论文发展脉络
Chat Paper
正在生成论文摘要