Development of a HAMSTER: Hyperspectral Albedo Maps dataset with high Spatial and TEmporal Resolution
crossref(2024)
摘要
Abstract. Surface albedo is an important parameter in radiative transfer simulations of the Earth's system, as it is fundamental to correctly calculate the energy budget of the planet. The Moderate Resolution Imaging Spectroradiometer (MODIS) instruments on NASA's Terra and Aqua satellites continuously monitor daily and yearly changes in reflection at the planetary surface. The MODIS Surface Reflectance dataset (MCD43C3, Version 6.1) gives detailed albedo maps in seven different spectral bands in the visible and near-infrared range. These albedo maps allow us to classify different Lambertian surface types and their seasonal and yearly variability and change, albeit only in seven spectral bands. However, a complete set of albedo maps covering the entire wavelength range is required to simulate radiance spectra, and to correctly retrieve atmospheric and cloud properties from Earth's remote sensing. We use a Principal Component Analysis (PCA) regression algorithm to generate hyperspectral albedo maps of Earth. Combining different datasets of hyperspectral reflectance laboratory measurements for various dry soils, vegetation surfaces, and mixtures of both, we reconstruct the albedo maps in the entire wavelength range from 400 to 2500 nm. The PCA method is trained with a 10-years average of MODIS data for different times of the year. We obtain hyperspectral albedo maps with a spatial resolution of 0.05° in latitude and longitude, a spectral resolution of 10 nm, and a temporal resolution of 8 days. Using the hyperspectral albedo maps, we estimate the spectral profiles of different land surfaces, such as forests, deserts, cities and icy surfaces, and study their seasonal variability. These albedo maps shall enable to refine calculations of Earth's energy budget, its seasonal variability, and improve climate simulations.
更多查看译文
AI 理解论文
溯源树
样例
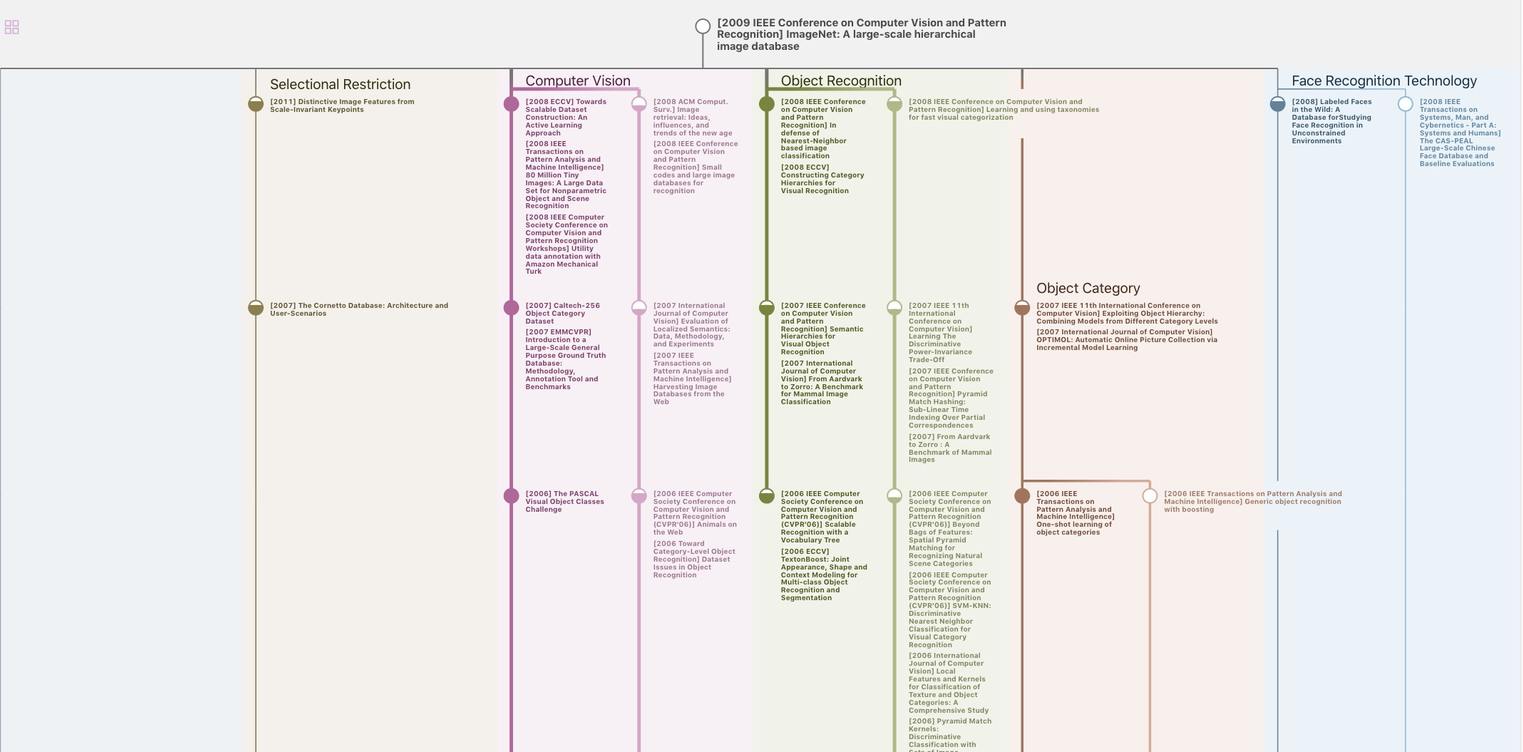
生成溯源树,研究论文发展脉络
Chat Paper
正在生成论文摘要