Task Allocation in Spatial Crowdsourcing: An Efficient Geographic Partition Framework
IEEE Transactions on Knowledge and Data Engineering(2024)
摘要
Recent years have witnessed a revolution in Spatial Crowdsourcing (SC), in which people with mobile connectivity can perform spatio-temporal tasks that involve traveling to specified locations. In this paper, we identify and study in depth a new multi-center-based task allocation problem in the context of SC, where multiple allocation centers exist. In particular, we aim to maximize the total number of the allocated tasks while minimizing the allocated task number difference. To solve the problem, we propose a two-phase framework, called Task Allocation with Geographic Partition, consisting of a geographic partition and a task allocation phase. The first phase divides the whole study area based on the allocation centers by using both a basic Voronoi diagram-based algorithm and an adaptive weighted Voronoi diagram-based algorithm. In the allocation phase, we utilize a Reinforcement Learning method to achieve the task allocation, where a graph neural network with the attention mechanism is used to learn the embeddings of allocation centers, delivery points, and workers. To further improve the efficiency, we propose an early stopping optimization strategy for the adaptive weighted Voronoi diagram-based algorithm in the geographic partition phase and give a distance-constrained graph pruning strategy for the Reinforcement Learning method in the task allocation phase. Extensive experiments give insight into the effectiveness and efficiency of the proposed solutions.
更多查看译文
关键词
Geographic partition,reinforcement learning,spatial crowdsourcing,task allocation
AI 理解论文
溯源树
样例
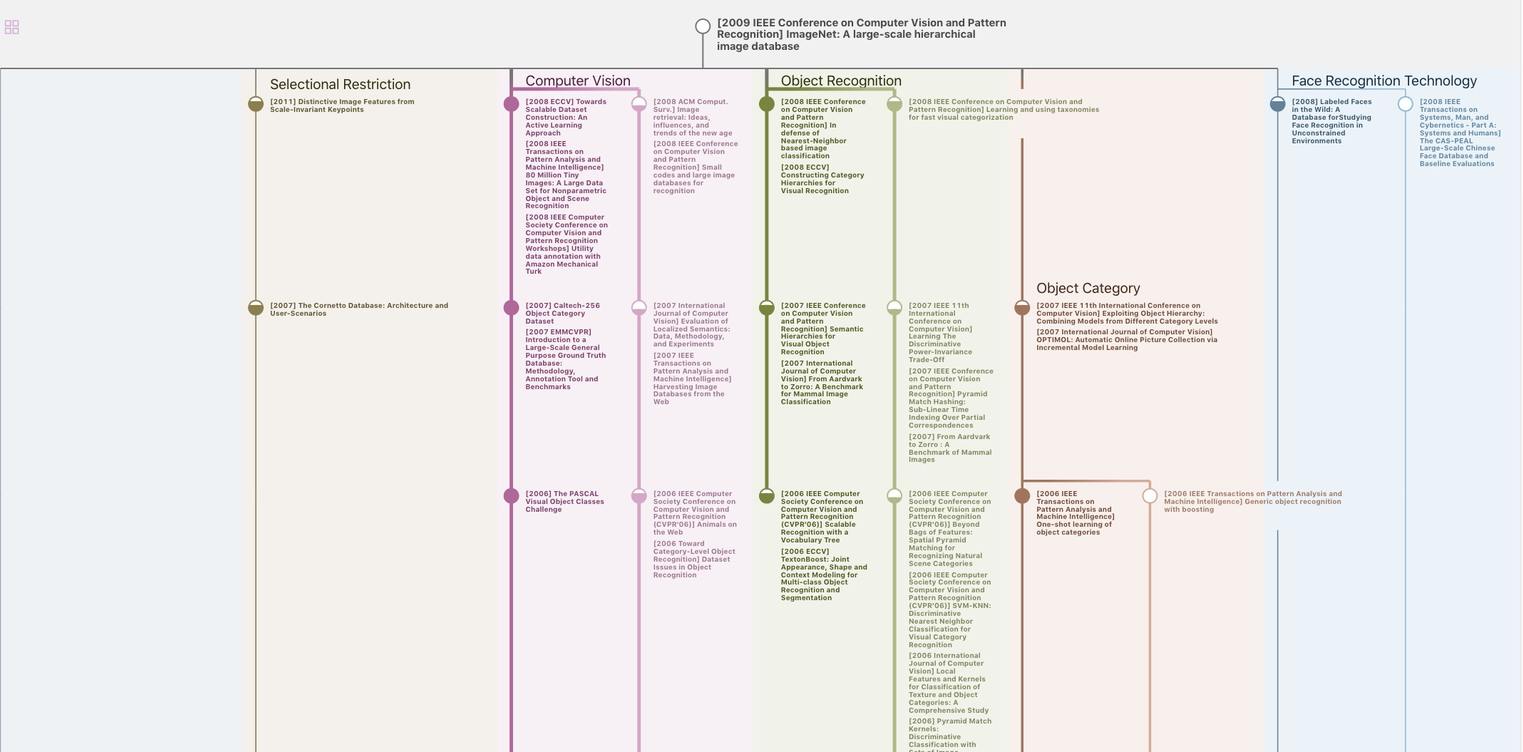
生成溯源树,研究论文发展脉络
Chat Paper
正在生成论文摘要