Development and validation of a deep learning model for predicting postoperative survival of patients with gastric cancer
BMC Public Health(2024)
摘要
Background Deep learning (DL), a specialized form of machine learning (ML), is valuable for forecasting survival in various diseases. Its clinical applicability in real-world patients with gastric cancer (GC) has yet to be extensively validated. Methods A combined cohort of 11,414 GC patients from the Surveillance, Epidemiology and End Results (SEER) database and 2,846 patients from a Chinese dataset were utilized. The internal validation of different algorithms, including DL model, traditional ML models, and American Joint Committee on Cancer (AJCC) stage model, was conducted by training and testing sets on the SEER database, followed by external validation on the Chinese dataset. The performance of the algorithms was assessed using the area under the receiver operating characteristic curve, decision curve, and calibration curve. Results DL model demonstrated superior performance in terms of the area under the curve (AUC) at 1, 3, and, 5 years post-surgery across both datasets, surpassing other ML models and AJCC stage model, with AUCs of 0.77, 0.80, and 0.82 in the SEER dataset and 0.77, 0.76, and 0.75 in the Chinese dataset, respectively. Furthermore, decision curve analysis revealed that the DL model yielded greater net gains at 3 years than other ML models and AJCC stage model, and calibration plots at 3 years indicated a favorable level of consistency between the ML and actual observations during external validation. Conclusions DL-based model was established to accurately predict the survival rate of postoperative patients with GC.
更多查看译文
关键词
Machine learning,Deep learning,Gastric cancer,Predictive model,Survival rate
AI 理解论文
溯源树
样例
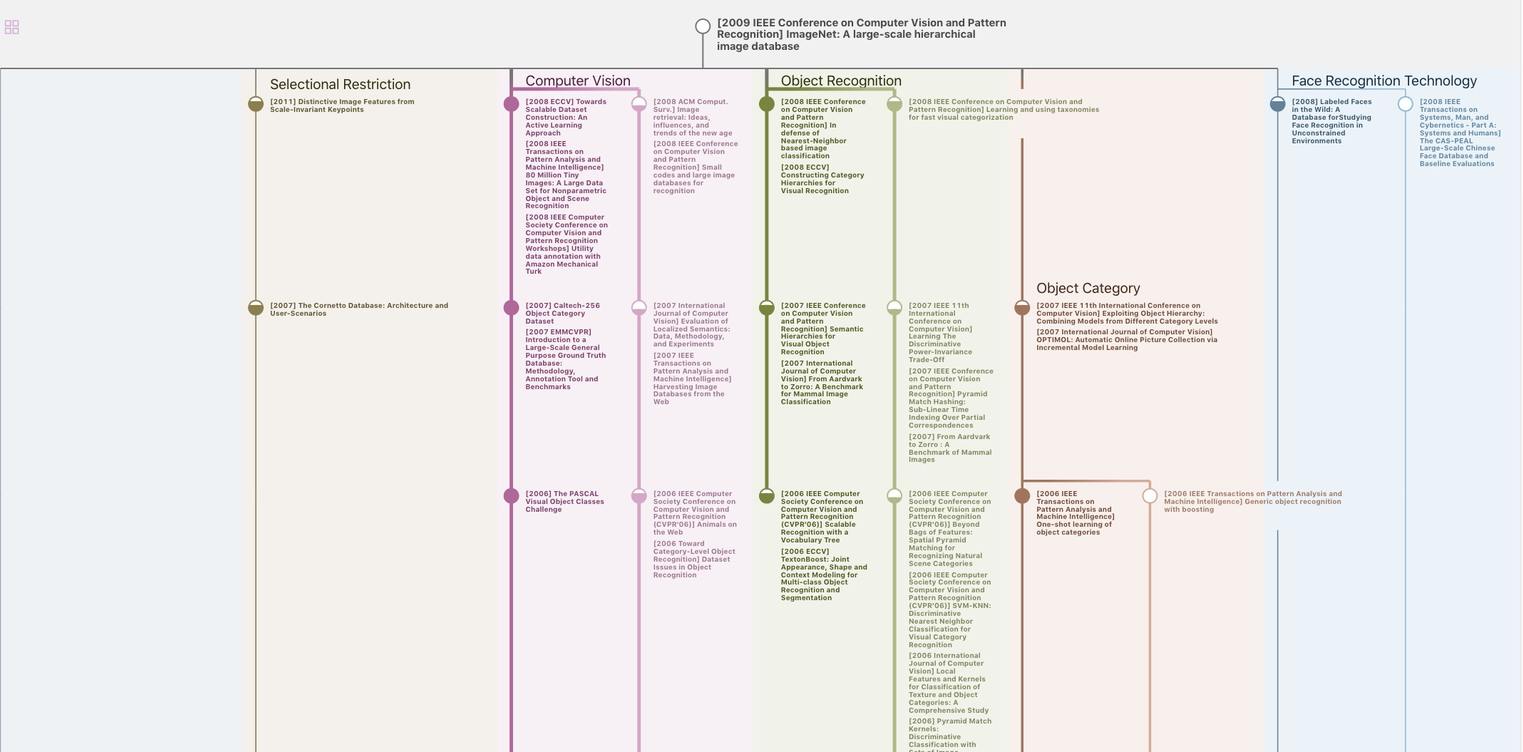
生成溯源树,研究论文发展脉络
Chat Paper
正在生成论文摘要