Three-dimensional autoencoder for the flow field reconstruction of an inclined circular disk
Ocean Engineering(2024)
摘要
At present, research on integrating deep learning with fluid dynamics has mostly focused on two-dimensional (2D) flow fields. Shifting from the study of 2D flow fields to the study of three-dimensional (3D) flow fields is crucial for accelerating the practical applications of deep learning in the real world. In this paper, we possess a high-quality direct numerical simulation dataset: 3D inclined circular disk flow fields with Reynolds numbers between 150 and 250, and a 3D convolutional autoencoder based on this dataset has been established to explore the specific performance of deep learning techniques in the reconstruction of 3D flow fields. The proposed model achieved significantly higher accuracy than proper orthogonal decomposition in terms of feature extraction in 3D inputs. When adding mask and noise to the inputs, the model exhibited impressive robustness: It had strong denoising capability under the Gaussian noise of k=10, and it can also reconstruct the flow fields well with inputs covered by one-eighth of the area. Additional slicing processing was applied to the model's output results during the research to highlight the differences between 3D flow field reconstruction tasks and the 2D scenario, allowing for detailed comparative analysis.
更多查看译文
关键词
Field reconstruction,Deep learning,Feature extraction
AI 理解论文
溯源树
样例
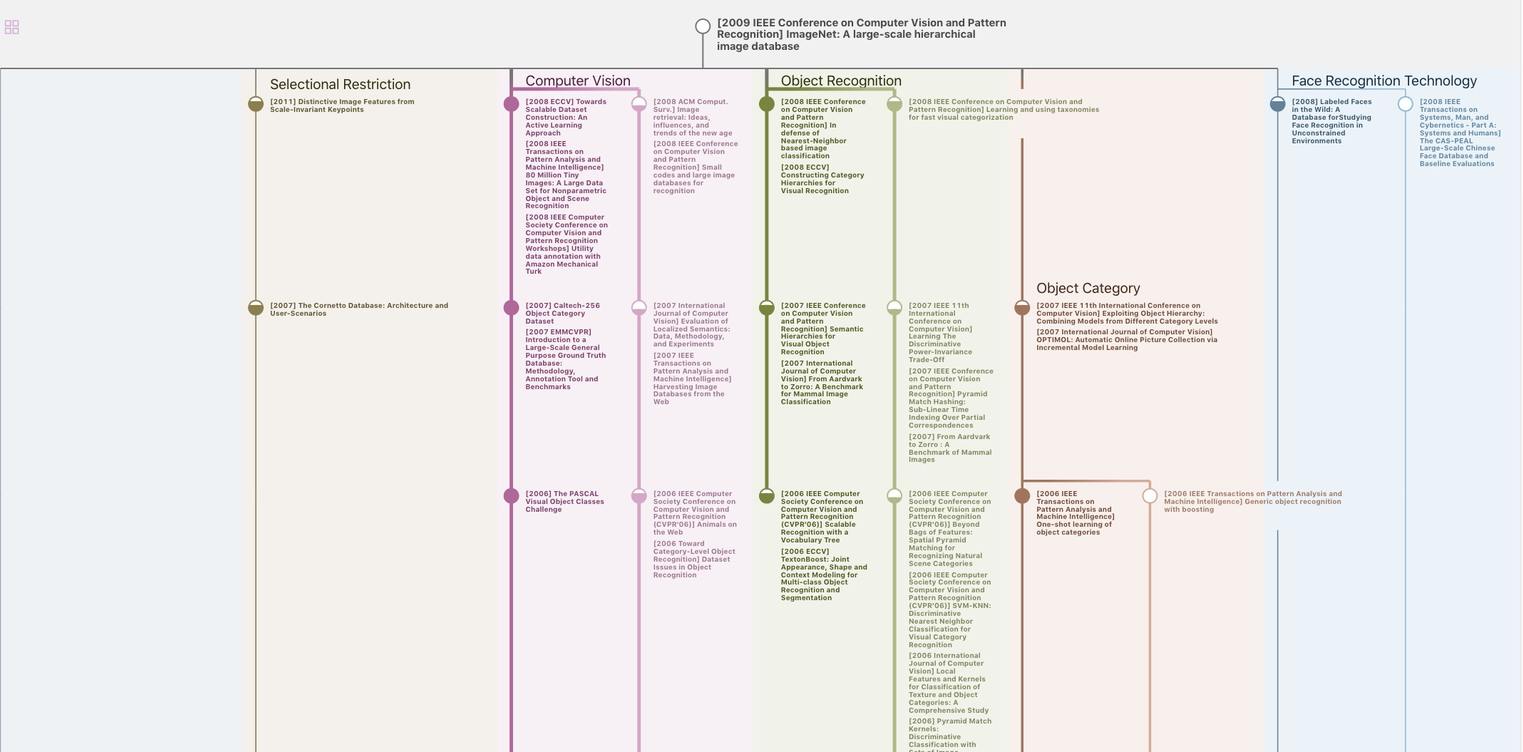
生成溯源树,研究论文发展脉络
Chat Paper
正在生成论文摘要