Prediction of tumor lysis syndrome in childhood acute lymphoblastic leukemia based on machine learning models: a retrospective study
FRONTIERS IN ONCOLOGY(2024)
摘要
Background Tumor lysis syndrome (TLS) often occurs early after induction chemotherapy for acute lymphoblastic leukemia (ALL) and can rapidly progress. This study aimed to construct a machine learning model to predict the risk of TLS using clinical indicators at the time of ALL diagnosis.Methods This observational cohort study was conducted at the National Clinical Research Center for Child Health and Disease. Data were collected from pediatric ALL patients diagnosed between December 2008 and December 2021. Four machine learning models were constructed using the Least Absolute Shrinkage and Selection Operator (LASSO) to select key clinical indicators for model construction.Results The study included 2,243 pediatric ALL patients, and the occurrence of TLS was 8.87%. A total of 33 indicators with missing values <= 30% were collected, and 12 risk factors were selected through LASSO regression analysis. The CatBoost model with the best performance after feature screening was selected to predict the TLS of ALL patients. The CatBoost model had an AUC of 0.832 and an accuracy of 0.758. The risk factors most associated with TLS were the absence of potassium, phosphorus, aspartate transaminase (AST), white blood cell count (WBC), and urea levels.Conclusion We developed the first TLS prediction model for pediatric ALL to assist clinicians in risk stratification at diagnosis and in developing personalized treatment protocols. This study is registered on the China Clinical Trials Registry platform (ChiCTR2200060616).Clinical trial registration https://www.chictr.org.cn/, identifier ChiCTR2200060616.
更多查看译文
关键词
machine learning,predictive modeling,acute lymphoblastic leukemia,tumor lysis syndrome,treatment toxicity
AI 理解论文
溯源树
样例
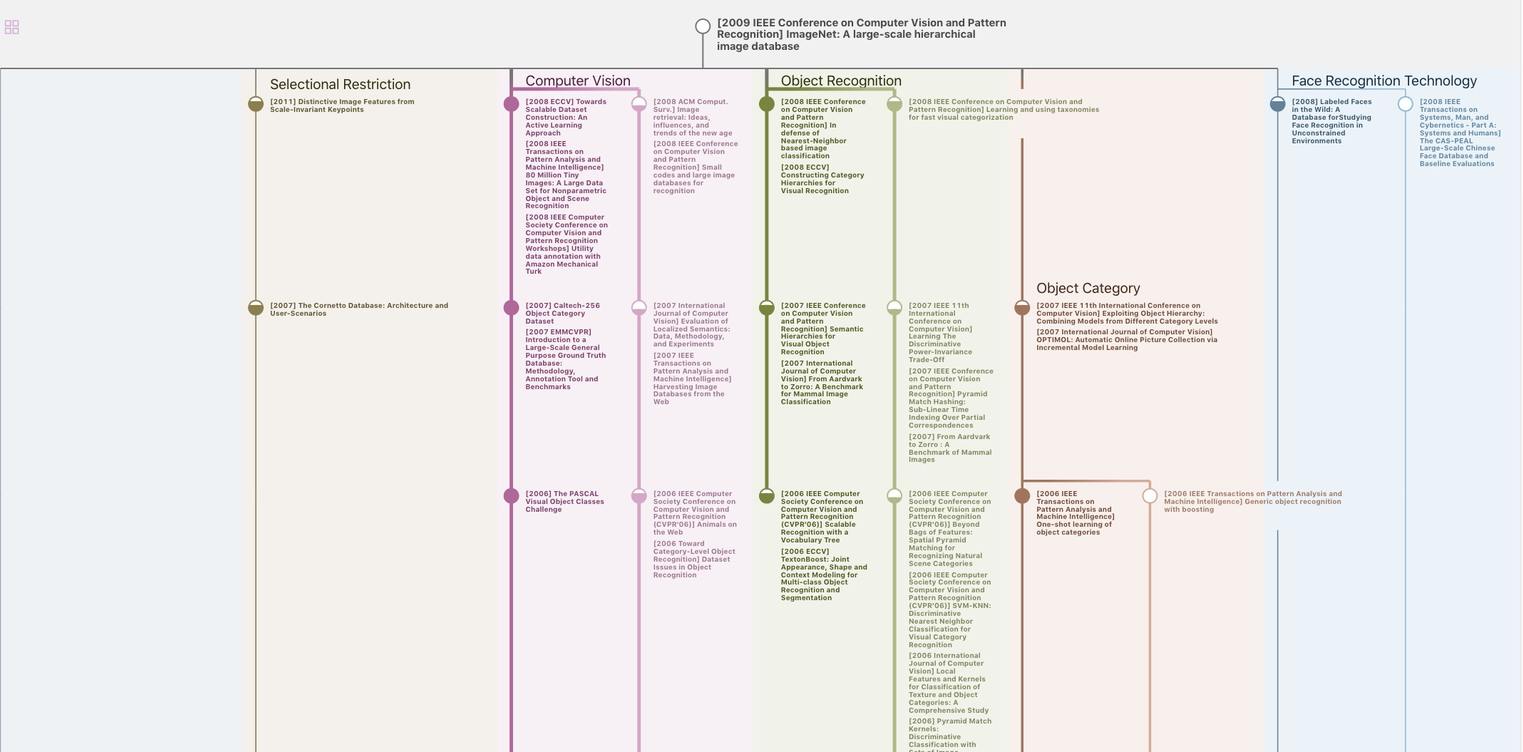
生成溯源树,研究论文发展脉络
Chat Paper
正在生成论文摘要