Missing Labels Feature Selection Based on Multilabel Multi-scale Fusion Fuzzy Rough Sets
2023 3rd International Conference on Digital Society and Intelligent Systems (DSInS)(2023)
摘要
The fuzzy rough set (FRS) theory has gained much attention due to its outstanding multilabel feature selection (MFS) performance. However, most existing FRS-based MFS algorithms assume that the training samples are accurately labeled, but this assumption is typically untenable in practical applications. In addition, the single-scale learning strategies are far from satisfying actual application needs. To this end, a missing label feature selection algorithm based on multilabel multi-scale fusion FRS (MFMS) is proposed. First, a novel multilabel multi-scale fuzzy information system is formalized, in which multilabel multi-scale fuzzy similarity relation is designed to characterize knowledge at different scales. Then, the classification relevance of multilabel data with missing labels is explored by fusing knowledge granularities at multiple scales to achieve accurate missing-label learning. On this basis, a multilabel multi-scale fusion FRS model for missing labels is constructed. Finally, an MFS algorithm for multilabel data containing missing labels is raised by using the multi-scale fusion fuzzy dependency function as inspiration. Extensive experiments prove the validity of the MFMS algorithm.
更多查看译文
AI 理解论文
溯源树
样例
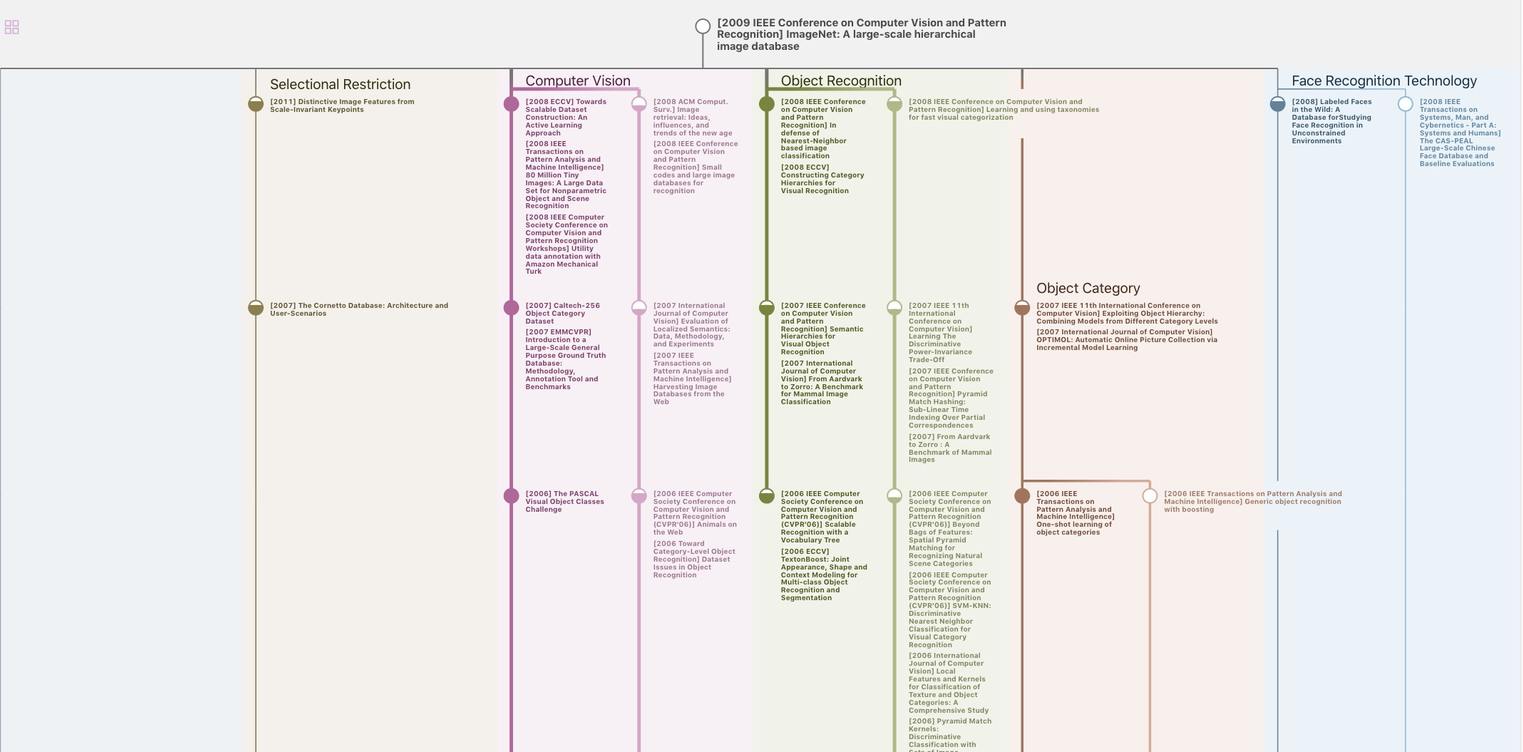
生成溯源树,研究论文发展脉络
Chat Paper
正在生成论文摘要