Generative Essential Graph Convolutional Network for Multi-View Semi-Supervised Classification
IEEE Transactions on Multimedia(2024)
摘要
Multi-view learning is a promising research field that aims to enhance learning performance by integrating information from diverse data perspectives. Due to the increasing interest in graph neural networks, researchers have gradually incorporated various graph models into multi-view learning. Despite significant progress, current methods face challenges in extracting information from multiple graphs while simultaneously accommodating specific downstream tasks. Additionally, the lack of a subsequent refinement process for the learned graph leads to the incorporation of noise. To address the aforementioned issues, we propose a method named generative essential graph convolutional network for multi-view semi-supervised classification. Our approach integrates the extraction of multi-graph consistency and complementarity, graph refinement, and classification tasks within a comprehensive optimization framework. This is accomplished by extracting a consistent graph from the shared representation, taking into account the complementarity of the original topologies. The learned graph is then optimized through downstream-specific tasks. Finally, we employ a graph convolutional network with a learnable threshold shrinkage function to acquire the graph embedding. Experimental results on benchmark datasets demonstrate the effectiveness of our approach.
更多查看译文
关键词
Muti-view learning,graph convolutional network,learnable graph,learnable threshold shrinkage activation
AI 理解论文
溯源树
样例
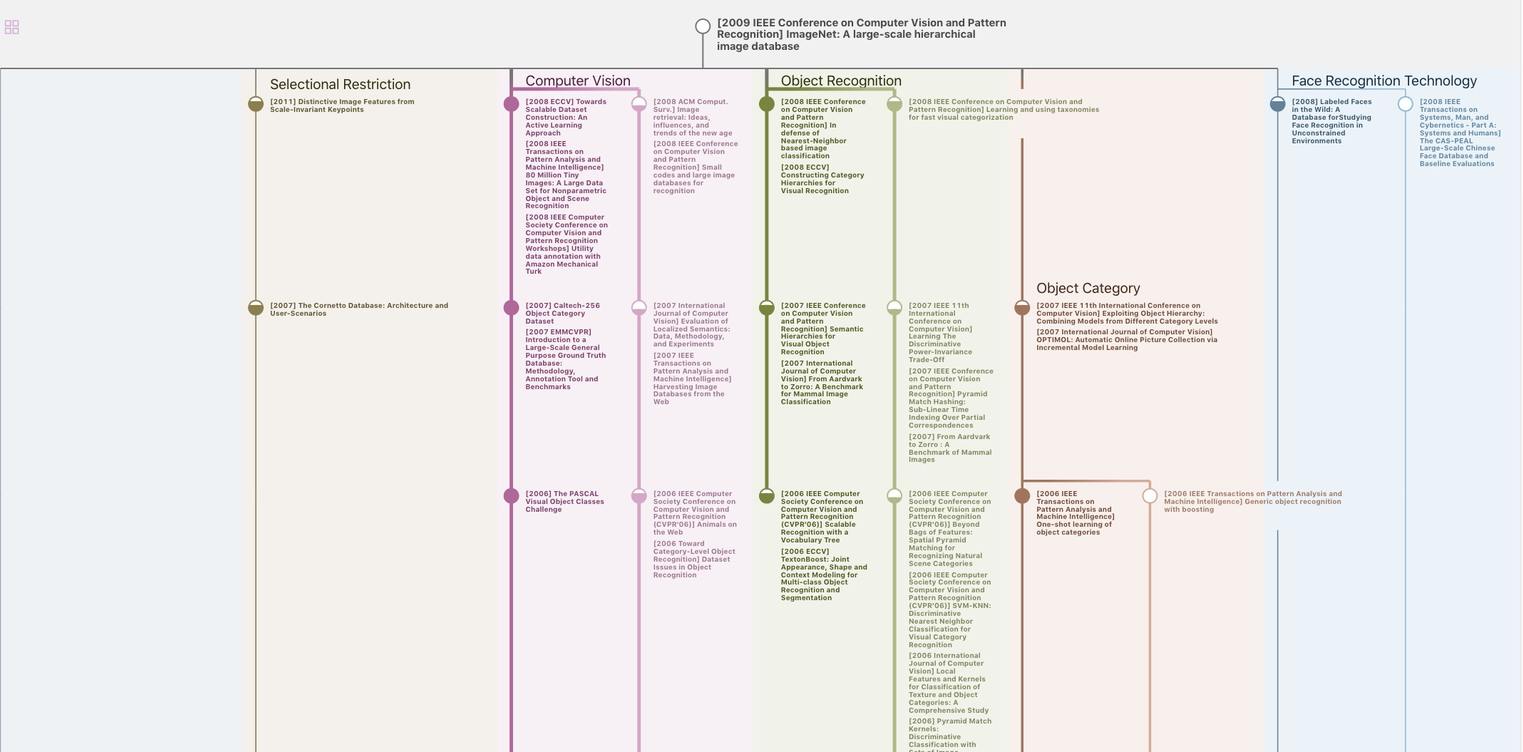
生成溯源树,研究论文发展脉络
Chat Paper
正在生成论文摘要