Deep Transfer Learning for Efficient and Accurate Prediction of Composite Pressure Vessel Behaviors
COMPOSITES PART A-APPLIED SCIENCE AND MANUFACTURING(2024)
摘要
Composite pressure vessels are manufactured by winding carbon fiber-reinforced plastic, while its performance is determined by fabrication conditions. To properly design composite pressure vessels, it is essential to analyze their behavior according to the design parameters. However, analytical or numerical methods have limitations in terms of accuracy or cost-effectiveness. In this study, a method is proposed to accurately and efficiently predict the behavior of composite pressure vessels through deep transfer learning. A prediction model is built by pre-training on a sufficient amount of analytical data, and then fine-tuning on a limited amount of numerical data. The performance of the deep transfer learning-based prediction model was evaluated through various error assessments. Its computational cost was also compared with that of finite element analysis. Furthermore, the deep transfer learning-based prediction model was used to analyze the impact of design parameters on the behavior of composite pressure vessels and for design optimization.
更多查看译文
关键词
Composite pressure vessel,Transfer learning,Machine learning,Carbon fiber-reinforced plastic (CFRP)
AI 理解论文
溯源树
样例
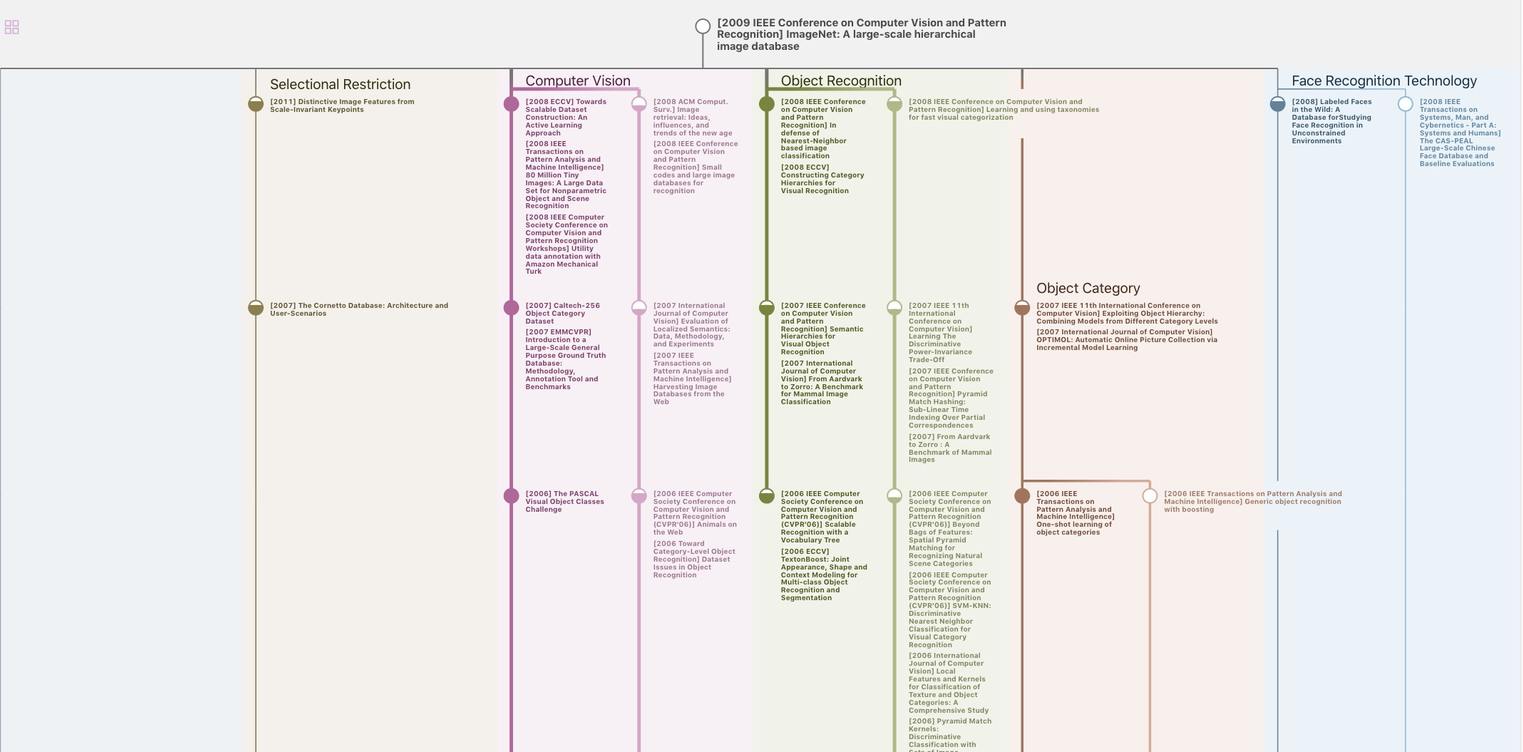
生成溯源树,研究论文发展脉络
Chat Paper
正在生成论文摘要