ISP-IRLNet: Joint optimization of interpretable sampler and implicit regularization learning network for accerlerated MRI
Pattern Recognition(2024)
摘要
Compressed Sensing Magnetic Resonance Imaging (CS-MRI) was proposed to accelerate data acquisition and reconstruct MR images from under-sampled data in k-space. However, the traditional approaches design the sampling patterns separately from the reconstruction process, which often leads to suboptimal reconstruction performance. To address this issue, we propose a joint optimization model dubbed as ISP-IRLNet to yield optimal recovery including an interpretable sampler and a reconstructor using the implicit regularization learning network. In particular, we introduce a probabilistic distribution model of the sampling incorporated into the prior of k-space locations, which provides a robust CS-MRI sample pattern with an explicit expression. To estimate the approximating gradient in backward propagation for more stable training, we employ a differentiable binarization strategy. In addition, we unroll the ADMM optimization for solving the regularized reconstruction model to be a deep network (i.e., IRLNet) with a learnable implicit regularization sub-network to learn a regularizer or prior term implicitly. The experiments on our collected brain and public knee datasets demonstrate that our method provides an optimal sample pattern and achieves superior image reconstruction performance compared with existing methods, especially at high acceleration rates.
更多查看译文
关键词
Compressed sensing magnetic resonance imaging,Deep learning,Interpretable sampler learning,Implicit regularization learning
AI 理解论文
溯源树
样例
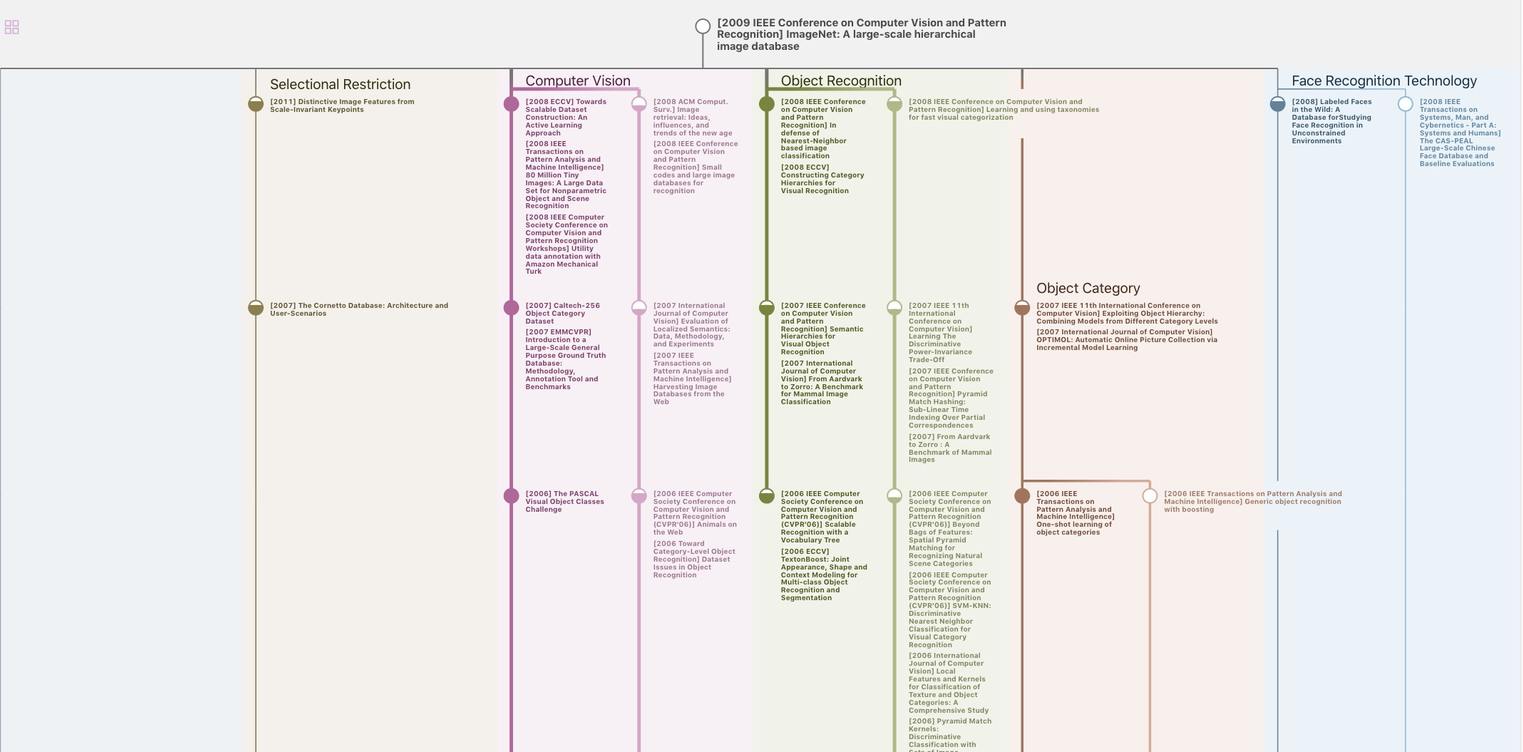
生成溯源树,研究论文发展脉络
Chat Paper
正在生成论文摘要