CD-MAE: Contrastive Dual-Masked Autoencoder Pre-Training Model for PCB CT Image Element Segmentation
ELECTRONICS(2024)
摘要
Element detection is an important step in the process of the non-destructive testing of printed circuit boards (PCB) based on computed tomography (CT). Compared with the traditional manual detection method, the image semantic segmentation method based on deep learning greatly improves efficiency and accuracy. However, semantic segmentation models often require a large amount of data for supervised training to generalize better model performance. Unlike natural images, the PCB CT image annotation task is more time-consuming and laborious than the semantic segmentation task. In order to reduce the cost of labeling and improve the ability of the model to utilize unlabeled data, unsupervised pre-training is a very reasonable and necessary choice. The masked image reconstruction model represented by a masked autoencoder is pre-trained on the unlabeled data, learning a strong feature representation ability by recovering the masked image, and shows a good generalization ability in various downstream tasks. In the PCB CT image element segmentation task, considering the characteristics of the image, it is necessary to use a model with strong feature robustness in the pre-training stage to realize the representation learning on a large number of unlabeled PCB CT images. Based on the above purposes, we proposed a contrastive dual-masked autoencoder (CD-MAE) pre-training model, which can learn more robust feature representation on unlabeled PCB CT images. Our experiments show that the CD-MAE outperforms the baseline model and fully supervised models in the PCB CT element segmentation task.
更多查看译文
关键词
unsupervised pre-training,image segmentation,PCB nondestructive testing,model finetuning
AI 理解论文
溯源树
样例
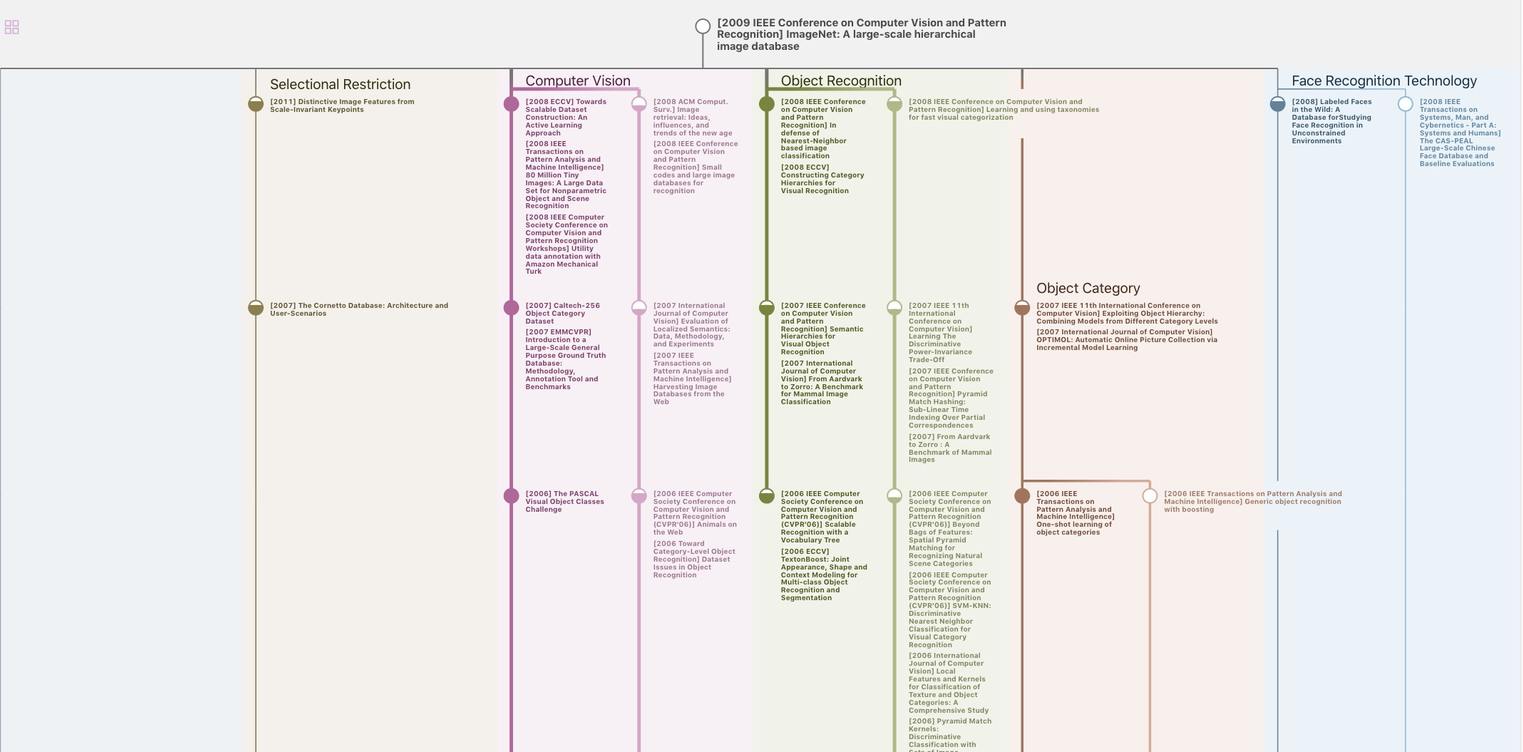
生成溯源树,研究论文发展脉络
Chat Paper
正在生成论文摘要