Reconstruction-Based Supervised Contrastive Learning for Unknown Device Identification in Nonintrusive Load Monitoring
IEEE TRANSACTIONS ON INSTRUMENTATION AND MEASUREMENT(2024)
摘要
Nonintrusive load monitoring (NILM) involves deploying monitoring devices at the customer's bus entrance to decompose the total power captured into individual operational data for each device. Existing NILM load identification algorithms primarily adopt closed-set identification, where the same types of devices are used for both training and testing. However, a more realistic scenario is open-set identification, where device types in the training and test sets differ, encompassing not only devices from the training set but also unknown devices. To address this challenge, we propose a method leveraging reconstruction and supervised contrastive learning based on voltage-current ( V - I ) trajectories. Supervised contrastive learning improves the coherence of V - I trajectories within identical appliance classes, facilitating the model's precise comprehension and emulation of distinctive appliance behavioral patterns. By introducing an autoencoder (AE) into the model, associating each appliance class with its unique subset of features. Known V - I trajectories activate associated features, preserving the topological characteristics of the trajectory. In contrast, unknown V - I trajectories lack reconstruction mappings, resulting in thoroughly mutated trajectories. Moreover, we innovate data augmentation for contrastive learning by introducing a denoising AE (DAE). This approach introduces noise in V - I trajectories, the model learns to remove noise while preserving real appliance characteristics, generating more robust V - I trajectories. Experimental results on two publicly available datasets demonstrate the method's outstanding performance.
更多查看译文
关键词
Autoencoder (AE),nonintrusive load monitor-ing (NILM),supervised contrastive learning,unknown devicesdetection,voltage-current (V-I) trajectory
AI 理解论文
溯源树
样例
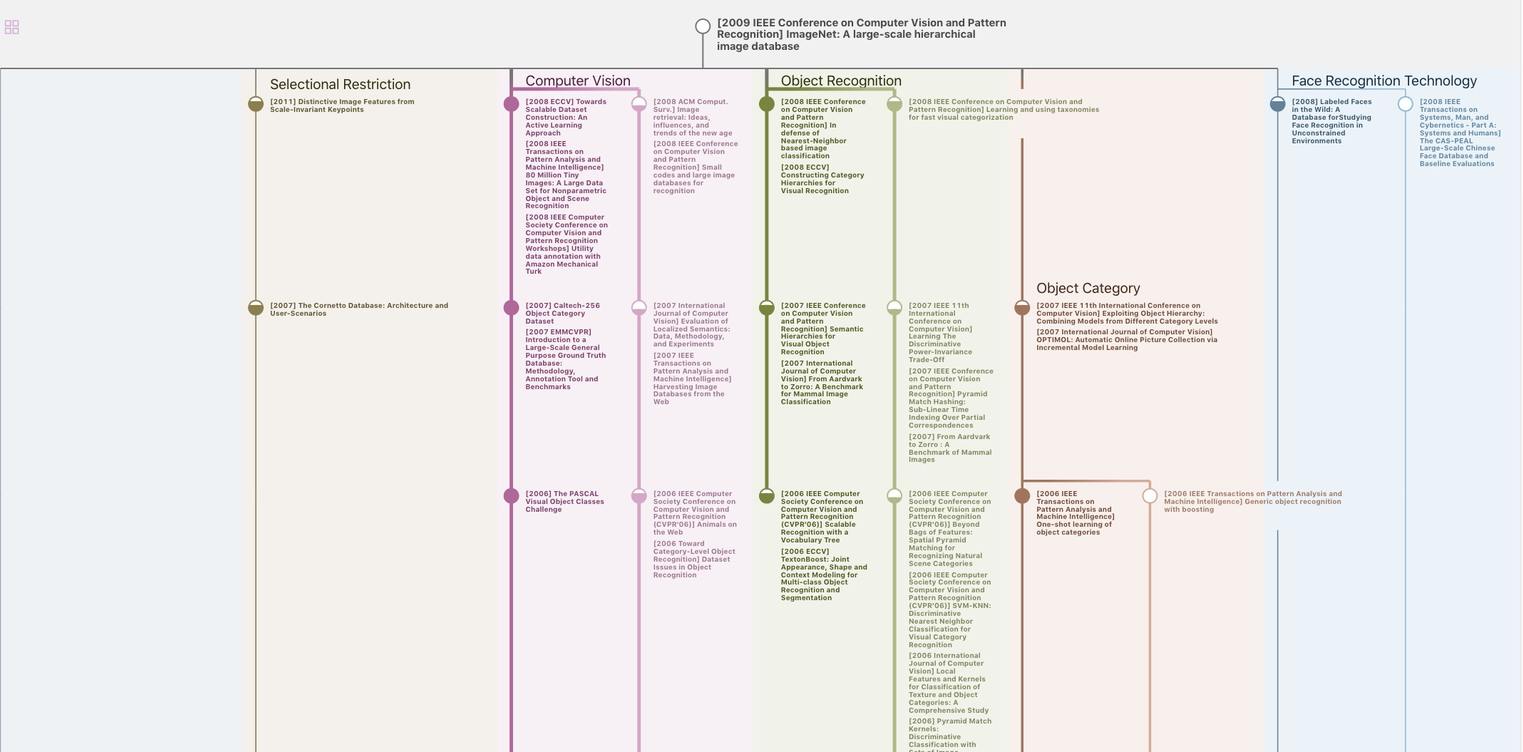
生成溯源树,研究论文发展脉络
Chat Paper
正在生成论文摘要