Brain MR image simulation for deep learning based medical image analysis networks
Computer Methods and Programs in Biomedicine(2024)
摘要
Background and ObjectiveAs large sets of annotated MRI data are needed for training and validating deep learning based medical image analysis algorithms, the lack of sufficient annotated data is a critical problem. A possible solution is the generation of artificial data by means of physics-based simulations. Existing brain simulation data is limited in terms of anatomical models, tissue classes, fixed tissue characteristics, MR sequences and overall realism.MethodsWe propose a realistic simulation framework by incorporating patient-specific phantoms and Bloch equations-based analytical solutions for fast and accurate MRI simulations. A large number of labels are derived from open-source high-resolution T1w MRI data using a fully automated brain classification tool. The brain labels are taken as ground truth (GT) on which MR images are simulated using our framework. Moreover, we demonstrate that the T1w MR images generated from our framework along with GT annotations can be utilized directly to train a 3D brain segmentation network. To evaluate our model further on larger set of real multi-source MRI data without GT, we compared our model to existing brain segmentation tools, FSL-FAST and SynthSeg.ResultsOur framework generates 3D brain MRI for variable anatomy, sequence, contrast, SNR and resolution. The brain segmentation network for WM/GM/CSF trained only on T1w simulated data shows promising results on real MRI data from MRBrainS18 challenge dataset with a Dice scores of 0.818/0.832/0.828. On OASIS data, our model exhibit a close performance to FSL, both qualitatively and quantitatively with a Dice scores of 0.901/0.939/0.937.ConclusionsOur proposed simulation framework is the initial step towards achieving truly physics-based MRI image generation, providing flexibility to generate large sets of variable MRI data for desired anatomy, sequence, contrast, SNR, and resolution. Furthermore, the generated images can effectively train 3D brain segmentation networks, mitigating the reliance on real 3D annotated data.
更多查看译文
关键词
Brain MRI simulation,Large synthetic population,Brain MRI segmentation,WM/GM/CSF segmentation
AI 理解论文
溯源树
样例
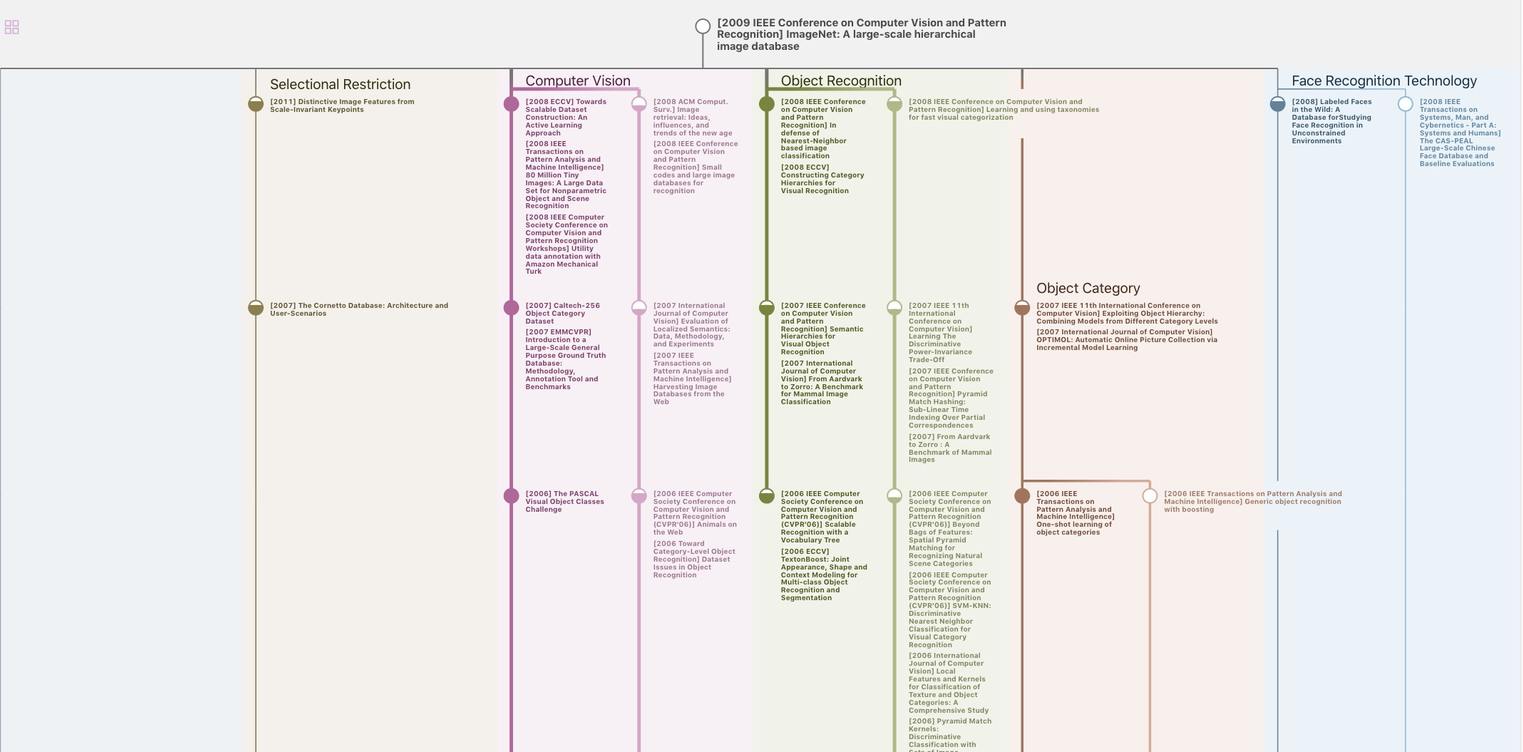
生成溯源树,研究论文发展脉络
Chat Paper
正在生成论文摘要