Semi-Supervised Dialogue Abstractive Summarization Via High-Quality Pseudolabel Selection
arXiv (Cornell University)(2024)
摘要
Semi-supervised dialogue summarization (SSDS) leverages model-generated summaries to reduce reliance on human-labeled data and improve the performance of summarization models. While addressing label noise, previous works on semi-supervised learning primarily focus on natural language understanding tasks, assuming each sample has a unique label. However, these methods are not directly applicable to SSDS, as it is a generative task, and each dialogue can be summarized in different ways. In this work, we propose a novel scoring approach, SiCF, which encapsulates three primary dimensions of summarization model quality: Semantic invariance (indicative of model confidence), Coverage (factual recall), and Faithfulness (factual precision). Using the SiCF score, we select unlabeled dialogues with high-quality generated summaries to train summarization models. Comprehensive experiments on three public datasets demonstrate the effectiveness of SiCF scores in uncertainty estimation and semi-supervised learning for dialogue summarization tasks. Our code is available at .
更多查看译文
关键词
Language Modeling,Part-of-Speech Tagging,Topic Modeling,Word Representation,Syntax-based Translation Models
AI 理解论文
溯源树
样例
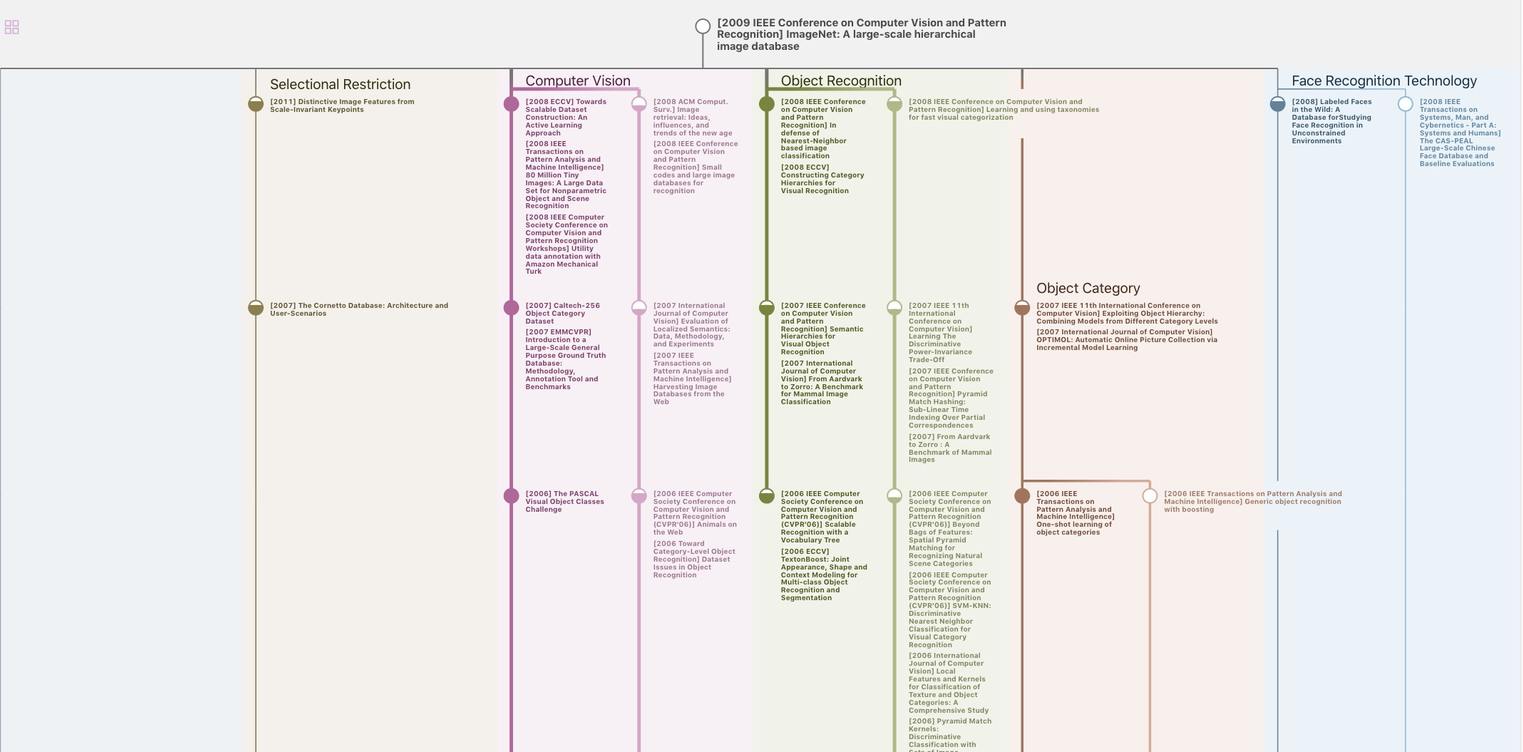
生成溯源树,研究论文发展脉络
Chat Paper
正在生成论文摘要