DCGG: drug combination prediction using GNN and GAE
Progress in Artificial Intelligence(2024)
摘要
Recent findings show that drug combination therapy can increase efficacy, decrease drug resistance, and reduce drug side effects. Due to the enormous number of possibilities in the selection of drugs, it is clinically impossible to screen all available combinations. Fortunately, artificial intelligence has opened up new perspectives for solving this problem by applying computationally intensive operations to predict drug combinations with high potential efficacy. These computational methods can be extremely resourceful for doctors and medical researchers to select drug combinations for the treatment of simple and complex diseases more cleverly and efficiently. In this paper, we propose an innovative solution for drug combination prediction called the DCGG method, in which the combination of node2vec, word2vec, indication, side effect, drug finger print, and drug targets is exploited for more enhanced prediction. DCGG is a combination of multiple Graph Auto Encoder (GAE) models that use Graph Neural Network (GNN) to prioritize potential novel, efficacious combination therapies. The comparison of DCGG with eight of the previous state-of-the-art models indicates the superiority of DCGG, which outperforms them by an average of 5
更多查看译文
关键词
Drug combination prediction,Drug–Drug Combinations,Graph Neural Network,Graph Auto Encoder,Graph convolution,Graph SAGE,Graph attention
AI 理解论文
溯源树
样例
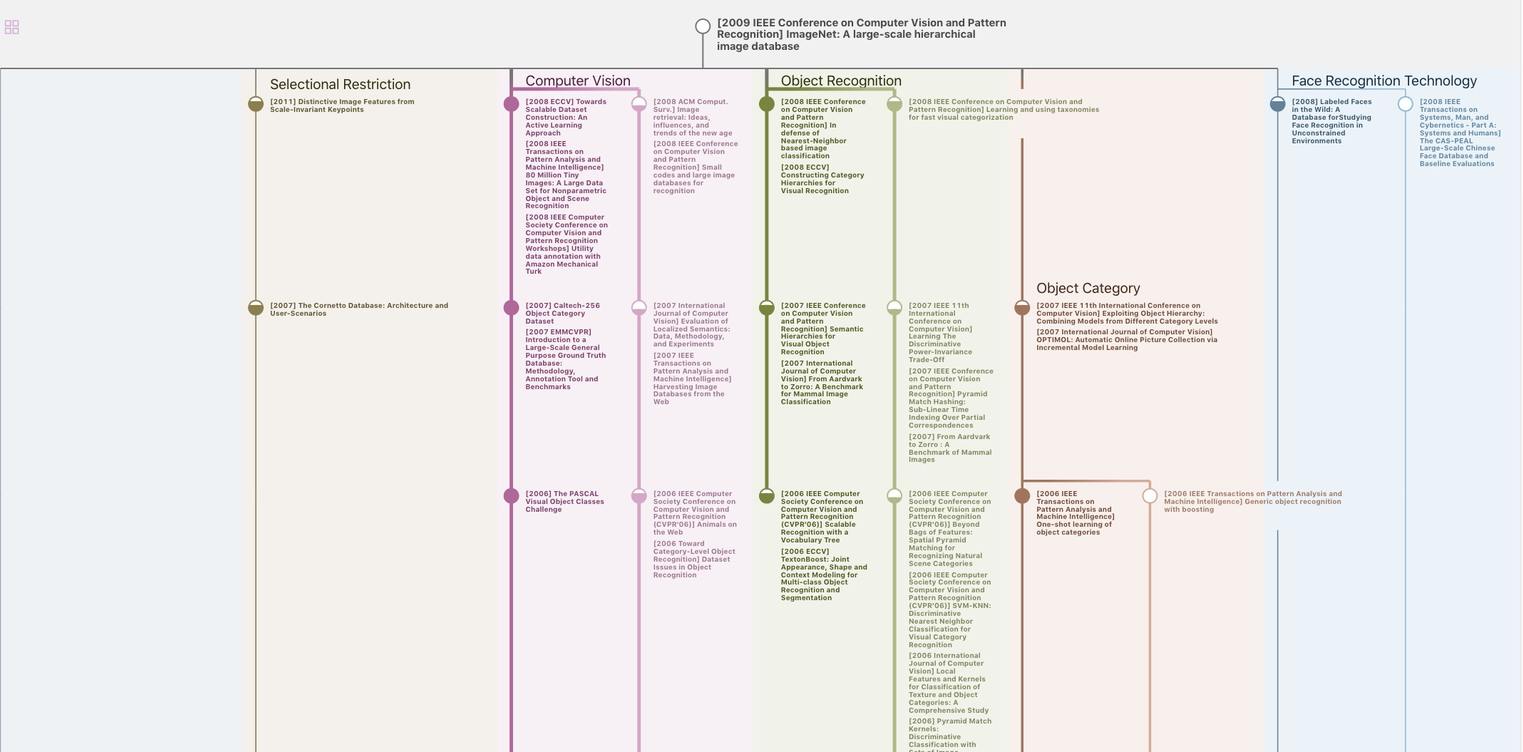
生成溯源树,研究论文发展脉络
Chat Paper
正在生成论文摘要