Segmentation and identification of brain tumour in MRI images using PG-OneShot learning CNN model
Multimedia Tools and Applications(2024)
摘要
Brain tumour segmentation plays a critical role in the diagnosis, treatment planning, and monitoring of brain tumour patients. However, accurate and efficient segmentation remains challenging due to the complex and heterogeneous structure of brain tumour regions. The current CNN models have shown good performance in brain tumour segmentation and identification, but several research challenges, like limited generalizability, Adaptive Model Complexity, etc., still need to be addressed. In this research, we propose a novel approach that combines the progressively growing and One-Shot learning approaches with a semantic segmentation network to enhance the accuracy and generalization of brain tumour segmentation in MRI images. Our method joins the strengths of progressively growing and One-Shot learning techniques with a semantic segmentation network, enabling improved generalization, effective feature selection, and continuous integration of contextual information at the pixel level. Experimental results on benchmark Br35H MRI image datasets demonstrate the dominance of our approach over existing methods in terms of segmentation accuracy and adaptability to diverse brain tumour instances. A total of 3000 images (1500 tumorous and 1500 non-tumorous images) were used during the training and testing of the model. The evaluation metrics reveal the high performance of our proposed model for brain tumour segmentation. Achieving high Dice Similarity Coefficients (0.9849), Intersection over Union (0.9319), accuracy (0.9520), precision (0.9235), and recall (0.9572) across average training, validation, and test sets. These results demonstrate the model's efficiency in accurately segmenting both tumorous and non-tumorous regions in MRI images.
更多查看译文
关键词
Brain Tumour,Segmentation,Progressively growing network,Semantic segmentation,CNN,Image processing
AI 理解论文
溯源树
样例
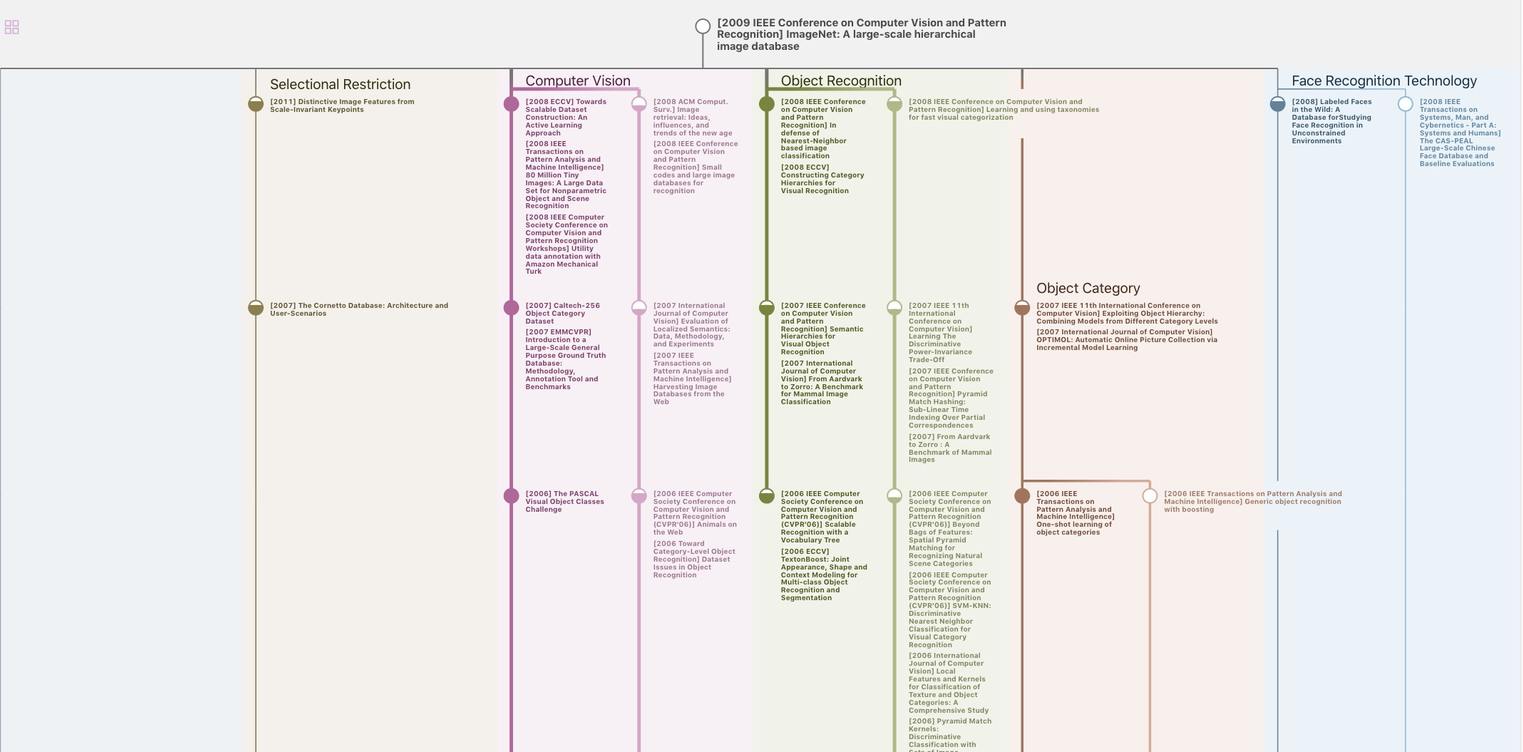
生成溯源树,研究论文发展脉络
Chat Paper
正在生成论文摘要