A General Calibrated Regret Metric for Detecting and Mitigating Human-Robot Interaction Failures
CoRR(2024)
摘要
Robot decision-making increasingly relies on expressive data-driven human
prediction models when operating around people. While these models are known to
suffer from prediction errors in out-of-distribution interactions, not all
prediction errors equally impact downstream robot performance. We identify that
the mathematical notion of regret precisely characterizes the degree to which
incorrect predictions of future interaction outcomes degraded closed-loop robot
performance. However, canonical regret measures are poorly calibrated across
diverse deployment interactions. We extend the canonical notion of regret by
deriving a calibrated regret metric that generalizes from absolute reward space
to probability space. With this transformation, our metric removes the need for
explicit reward functions to calculate the robot's regret, enables fairer
comparison of interaction anomalies across disparate deployment contexts, and
facilitates targetted dataset construction of "system- level" prediction
failures. We experimentally quantify the value of this high-regret interaction
data for aiding the robot in improving its downstream decision-making. In a
suite of closed- loop autonomous driving simulations, we find that fine-tuning
ego-conditioned behavior predictors exclusively on high-regret human-robot
interaction data can improve the robot's overall re-deployment performance with
significantly (77
更多查看译文
AI 理解论文
溯源树
样例
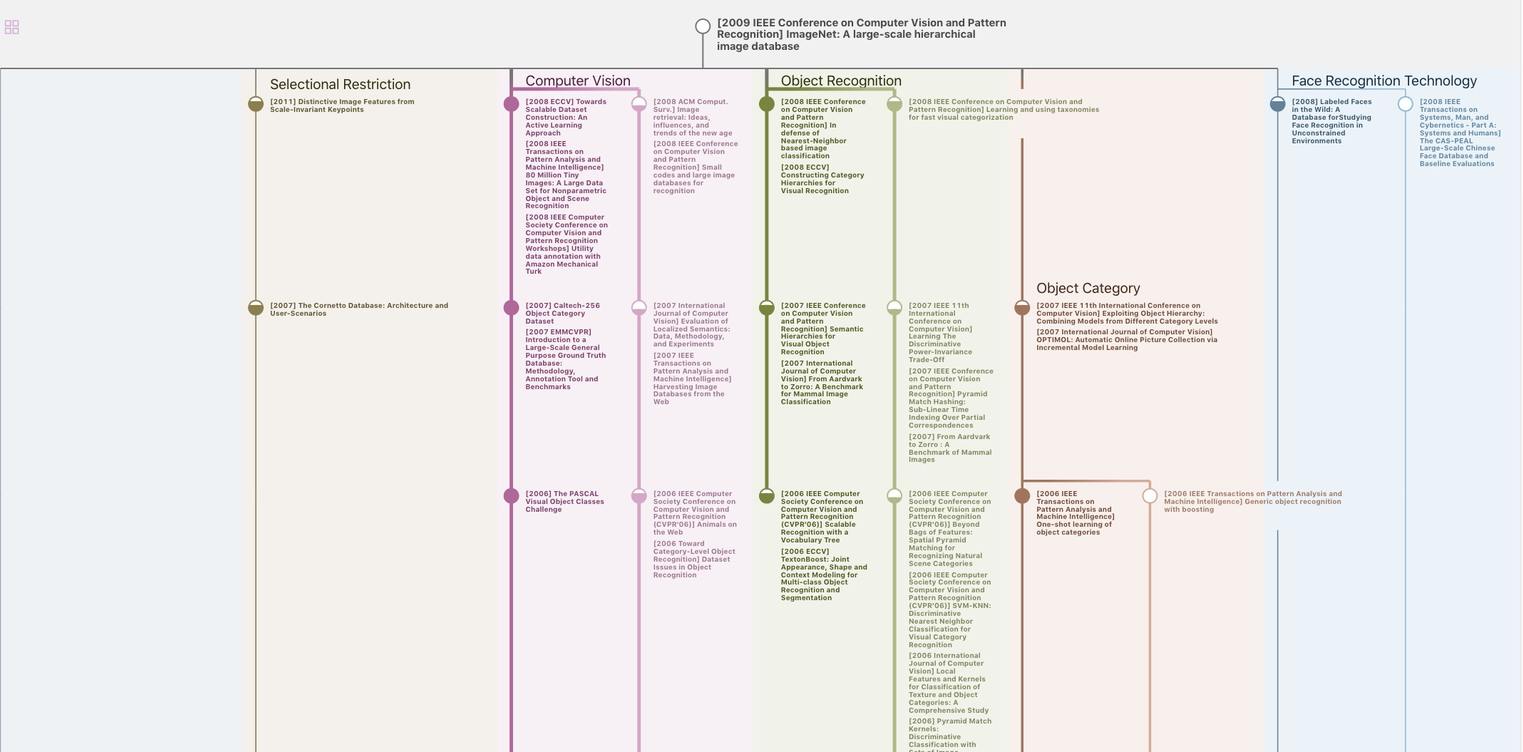
生成溯源树,研究论文发展脉络
Chat Paper
正在生成论文摘要