Generalized Policy Iteration using Tensor Approximation for Hybrid Control
ICLR 2024(2024)
摘要
Control of dynamic systems involving hybrid actions is a challenging task in robotics. To address this, we present a novel algorithm called Generalized Policy Iteration using Tensor Train (TTPI) that belongs to the class of Approximate Dynamic Programming (ADP). We use a low-rank tensor approximation technique called Tensor Train (TT) to approximate the state-value and advantage function which enables us to efficiently handle hybrid systems. We demonstrate the superiority of our approach over previous baselines for some benchmark problems with hybrid action spaces. Additionally, the robustness and generalization of the policy for hybrid systems are showcased through a real-world robotics experiment involving a non-prehensile manipulation task which is considered to be a highly challenging control problem.
更多查看译文
关键词
Optimal Control,Hybrid Actions,Robotics,Approximate Dynamic Programming,Tensor Approximation
AI 理解论文
溯源树
样例
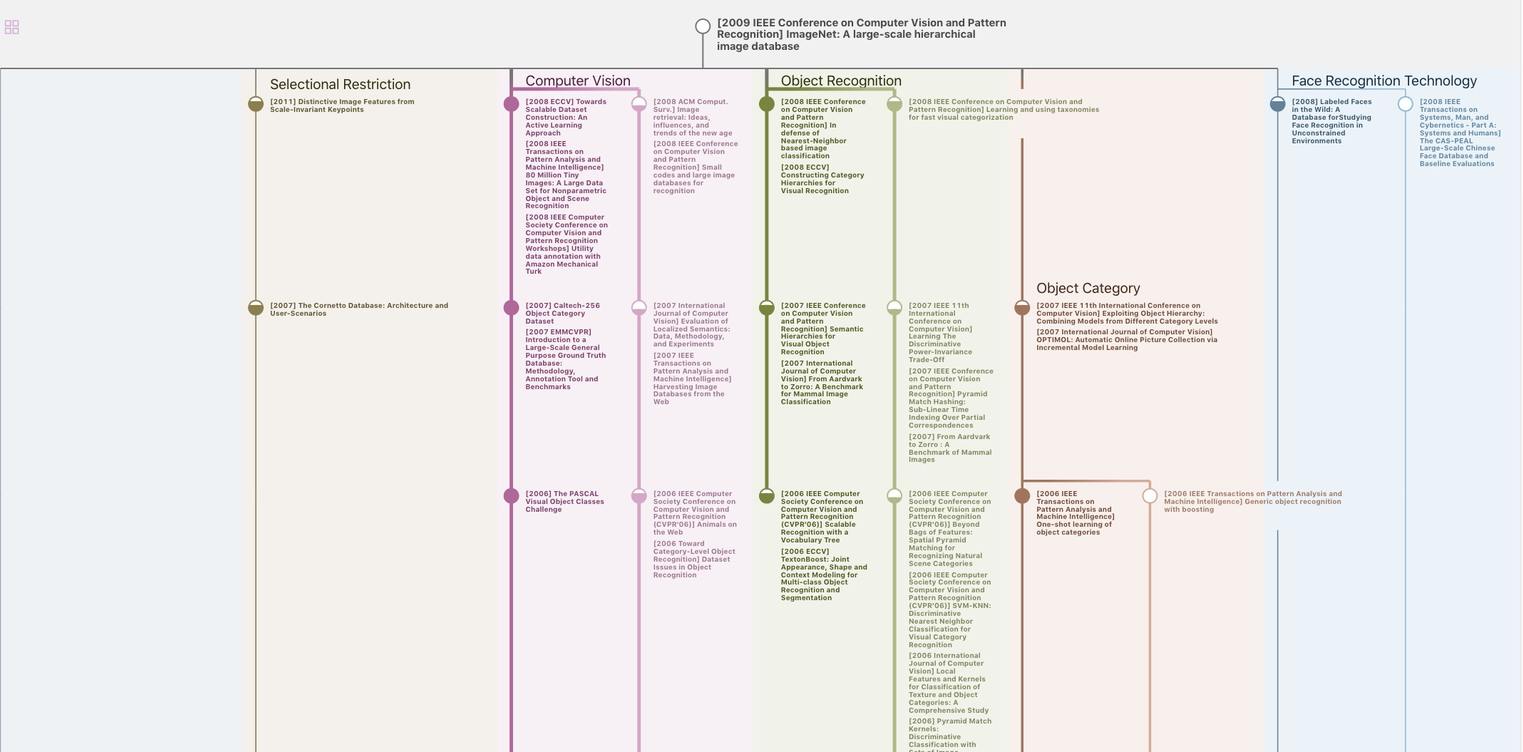
生成溯源树,研究论文发展脉络
Chat Paper
正在生成论文摘要