Learning Hierarchical World Models with Adaptive Temporal Abstractions from Discrete Latent Dynamics
ICLR 2024(2024)
摘要
Hierarchical world models can significantly improve model-based reinforcement learning (MBRL) and planning by enabling reasoning across multiple time scales. Nonetheless, the majority of state-of-the-art MBRL methods still employ flat, non-hierarchical models. We propose Temporal Hierarchies from Invariant Context Kernels (THICK), an algorithm that learns a world model hierarchy via discrete latent dynamics. The lower level of THICK updates parts of its latent state sparsely in time, forming invariant contexts. The higher level exclusively predicts situations involving context state changes. Our experiments demonstrate that THICK learns categorical, interpretable, temporal abstractions on the high level, while maintaining precise low-level predictions. Furthermore, we show that the emergent hierarchical predictive model seamlessly enhances the abilities of MBRL or planning methods. We believe that THICK contributes to the further development of hierarchical, context-conditioned, event-predictive world models that can enhance planning and reasoning abilities and produce more human-like behavior.
更多查看译文
关键词
world models,temporal abstraction,hierarchical learning,model-based reinforcement learning,hierarchical planning
AI 理解论文
溯源树
样例
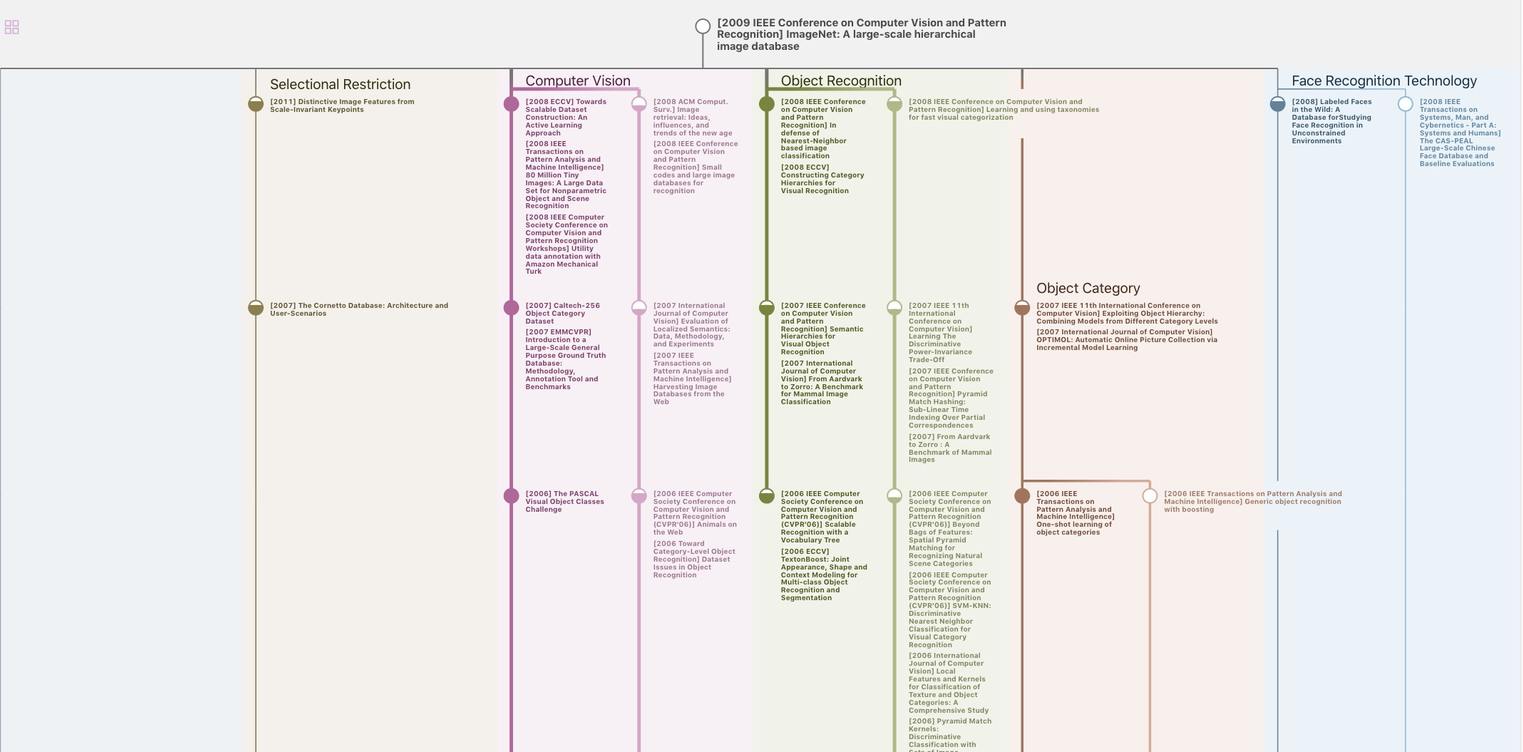
生成溯源树,研究论文发展脉络
Chat Paper
正在生成论文摘要